Transitive Sequential Pattern Mining for Discrete Clinical Data.
AIME(2020)
摘要
Electronic health records (EHRs) contain important temporal information about disease progression and patients. However, mining temporal representations from discrete EHR data (e.g., diagnosis, medication, or procedure codes) for use in standard Machine Learning is challenging. We propose a transitive Sequential Pattern Mining approach (tSPM) to address the temporal irregularities involved in recording discrete records in EHRs. We perform experiments to compare the classification performance metrics for predicting “true” diagnosis between traditional sequential pattern mining (SPM) and the proposed tSPM algorithms across multiple diseases. We demonstrate that transitive approach is superior to the traditional SPM in mining temporal representations for diagnosis prediction.
更多查看译文
关键词
Transitive Sequential Pattern Mining, Electronic Health Records, Temporal representations
AI 理解论文
溯源树
样例
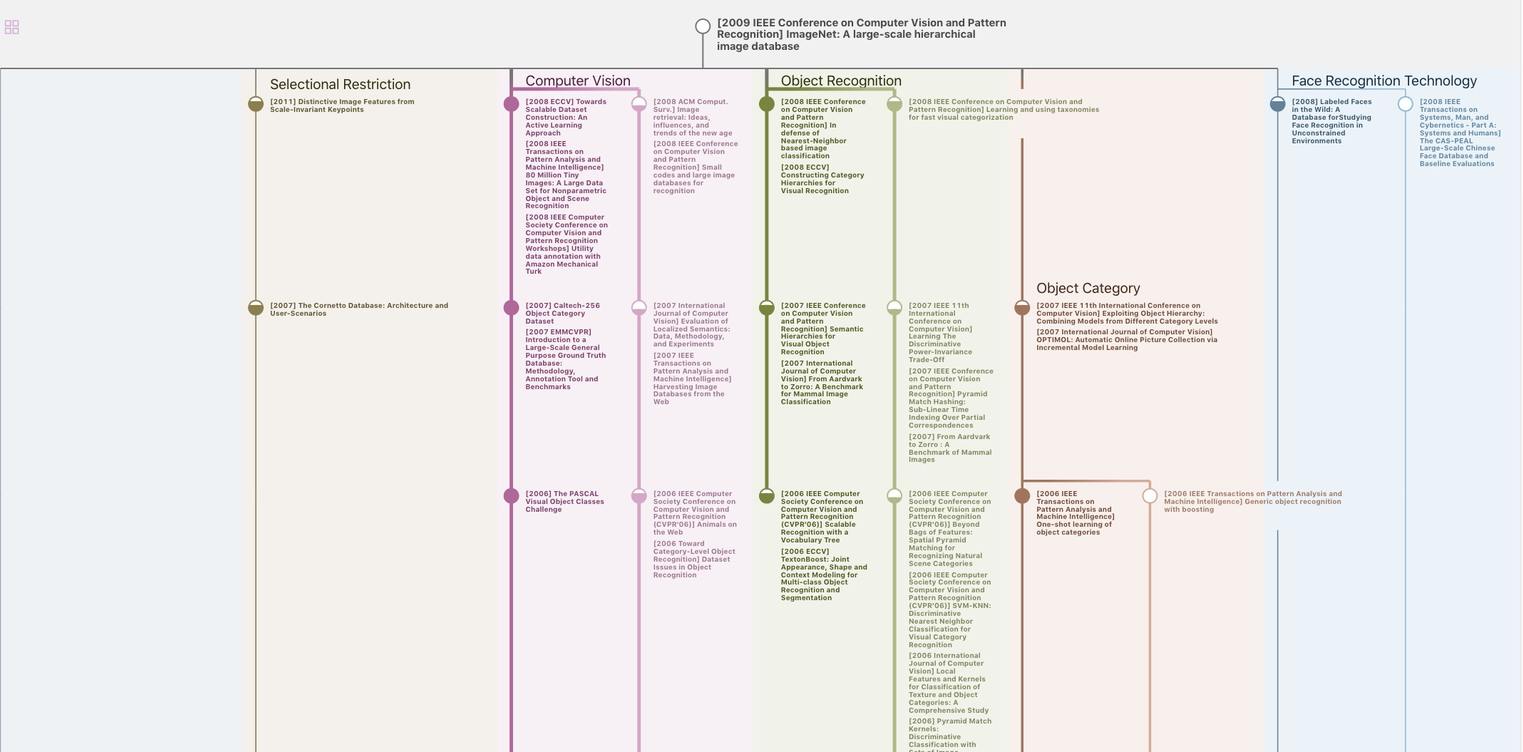
生成溯源树,研究论文发展脉络
Chat Paper
正在生成论文摘要