Label-Embedding-Based Multi-core Convolution for Text Categorization
2020 12th International Conference on Advanced Computational Intelligence (ICACI)(2020)
摘要
Text categorization is a classical problem in Natural Language Processing (NLP) tasks. There have been a number of studies that applied Convolutional Neural Networks (CNN) to this problem. This paper proposes a novel model to improve the traditional convolution for text classification, which is based on label embedding. It learns the attention on a training set oflabeled samples to ensure that the weight of related words is higher than that of irrelevant words. Meanwhile, the model also encodes the bi-gram features extracted from training data into convolutional filters, instead of randomly initializing them, which can help it focus on learning important semantic features at the beginning of the training. Experimental results on several text datasets indicate that the proposed model is competitive to the state-of-the-art models.
更多查看译文
关键词
text categorization,label embedding,convolution,filter initialization
AI 理解论文
溯源树
样例
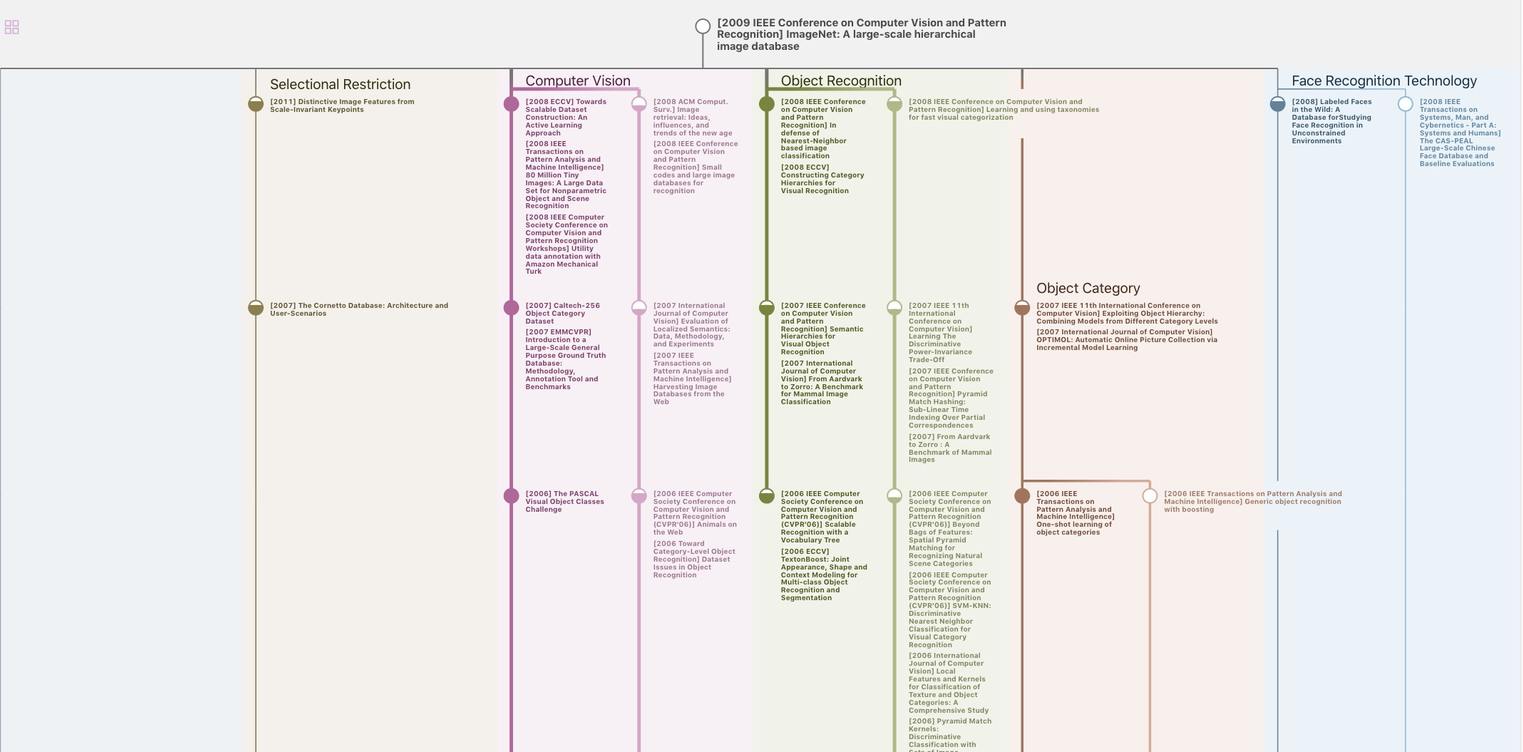
生成溯源树,研究论文发展脉络
Chat Paper
正在生成论文摘要