SA-Net: Robust State-Action Recognition for Learning from Observations
ICRA(2020)
摘要
Learning from observation (LfO) offers a new paradigm for transferring task behavior to robots. LfO requires the robot to observe the task being performed and decompose the sensed streaming data into sequences of state-action pairs, which are then input to LfO methods. Thus, recognizing the state-action pairs correctly and quickly in sensed data is a crucial prerequisite. We present SA-Net a deep neural network architecture that recognizes state-action pairs from RGB-D data streams. SA-Net performs well in two replicated robotic applications of LfO - one involving mobile ground robots and another involving a robotic manipulator - which demonstrates that the architecture could generalize well to differing contexts. Comprehensive evaluations including deployment on a physical robot show that SA-Net significantly improves on the accuracy of the previous methods under various conditions.
更多查看译文
关键词
robust state-action recognition,LfO methods,SA-Net,RGB-D data streams,replicated robotic applications,mobile ground robots,robotic manipulator,physical robot,deep neural network architecture,learning from observation
AI 理解论文
溯源树
样例
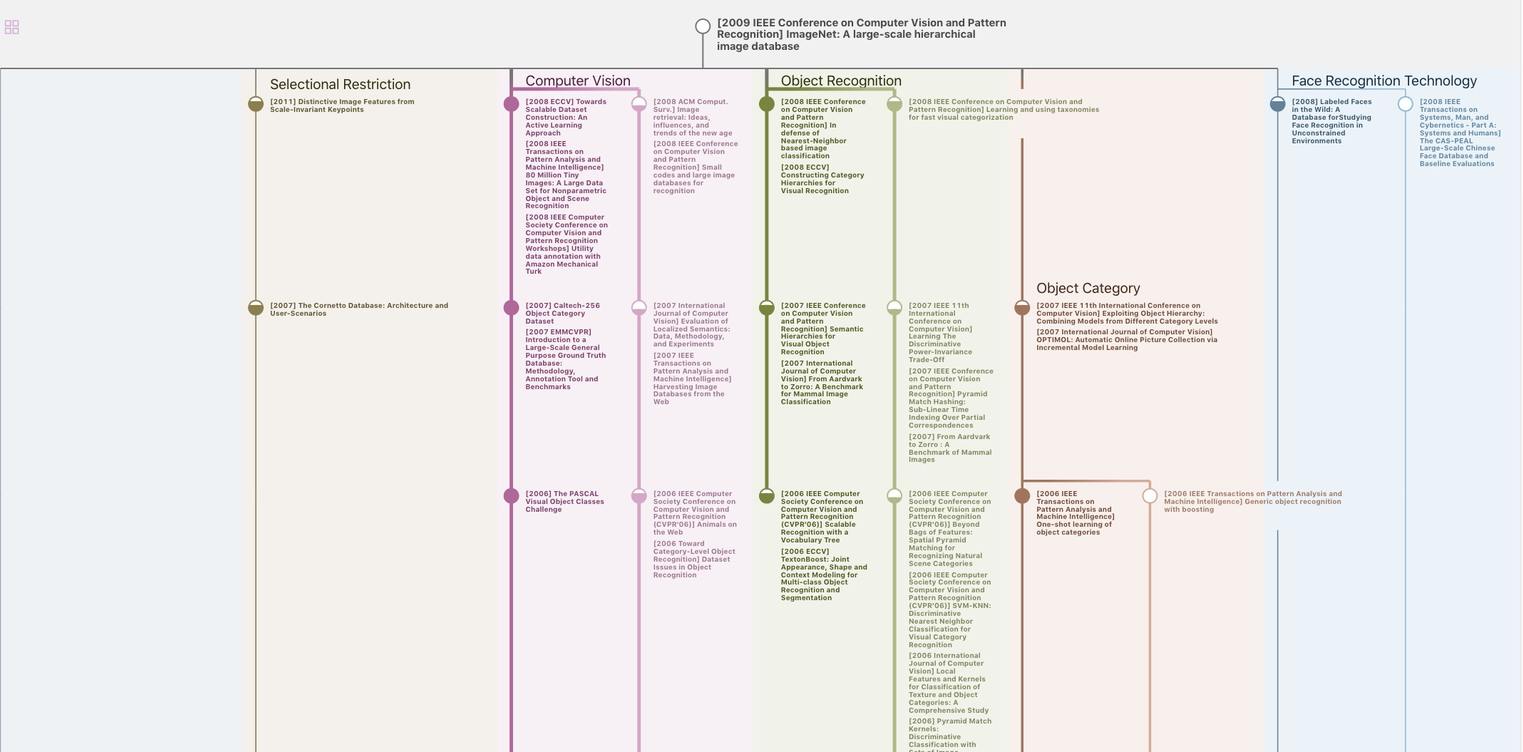
生成溯源树,研究论文发展脉络
Chat Paper
正在生成论文摘要