Lightweight Neural Networks From Pca & Lda Based Distilled Dense Neural Networks
2020 IEEE INTERNATIONAL CONFERENCE ON IMAGE PROCESSING (ICIP)(2020)
摘要
This paper presents two methods for building lightweight neural networks with similar accuracy than heavyweight ones with the advantage to be less greedy in memory and computing resources. So it can be implemented in edge and IoT devices. The presented distillation methods are respectively based on Principal Component Analysis (PCA) and Linear Discriminant Analysis (LDA). The two methods basically rely on the successive dimension reduction of a given dense neural network (teacher) hidden features, and the learning of a smaller neural network (student) which solves the initial learning problem along with a mapping problem to the reduced successive features spaces. The presented methods are compared to baselines-learning the student networks from scratch-, and we show that the additional mapping problem significantly improves the performance (accuracy, memory and computing resources) of the student networks.
更多查看译文
关键词
Teacher-Student Networks, Compression, Distillation, PCA, LDA, Lightweight Networks
AI 理解论文
溯源树
样例
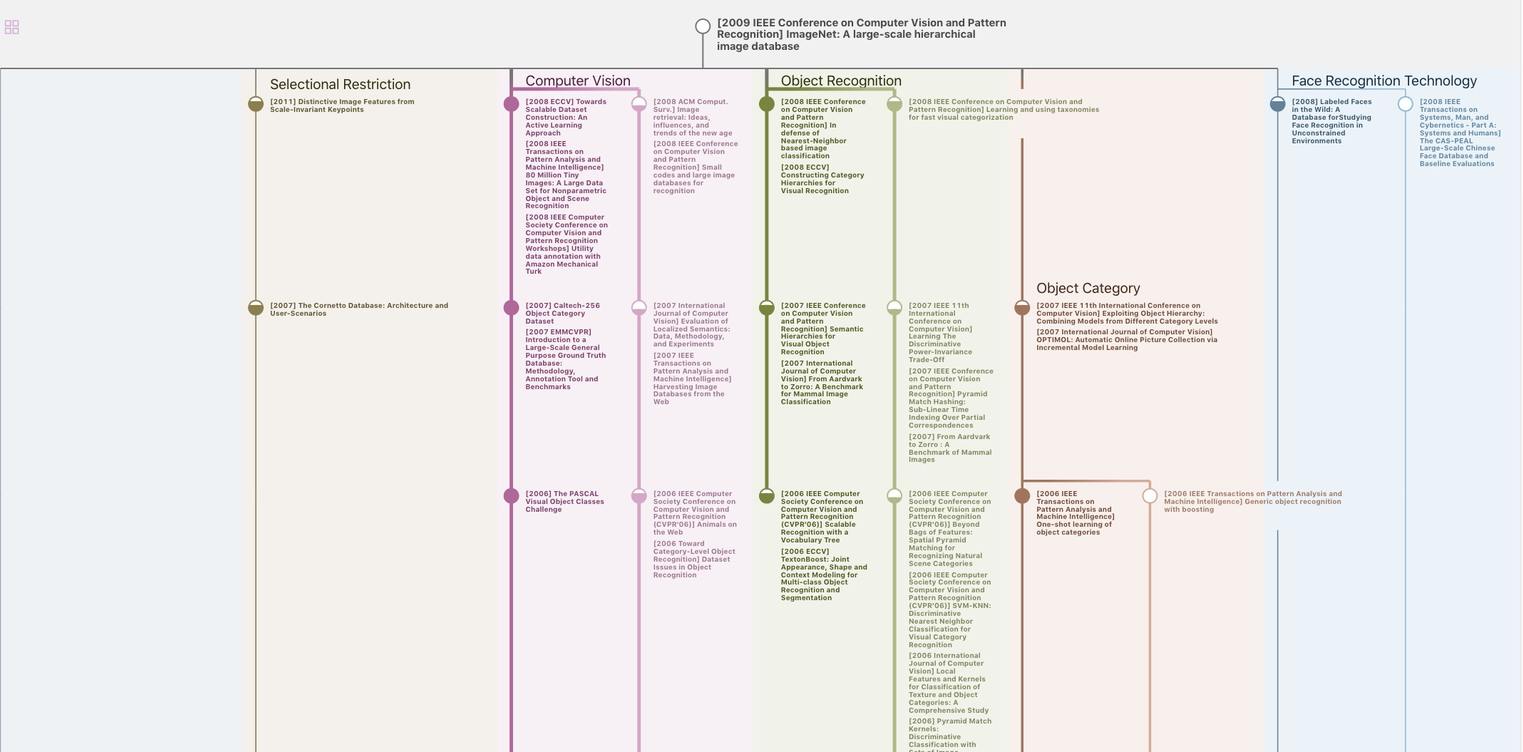
生成溯源树,研究论文发展脉络
Chat Paper
正在生成论文摘要