Triggering Proactive Business Process Adaptations via Online Reinforcement Learning.
BPM(2020)
摘要
Proactive process adaptation can prevent and mitigate upcoming problems during process execution by using predictions about how an ongoing case will unfold. There is an important trade-off with respect to these predictions: Earlier predictions leave more time for adaptations than later predictions, but earlier predictions typically exhibit a lower accuracy than later predictions, because not much information about the ongoing case is available. An emerging solution to address this trade-off is to continuously generate predictions and only trigger proactive adaptations when prediction reliability is greater than a predefined threshold. However, a good threshold is not known a priori. One solution is to empirically determine the threshold using a subset of the training data. While an empirical threshold may be optimal for the training data used and the given cost structure, such a threshold may not be optimal over time due to non-stationarity of process environments, data, and cost structures. Here, we use online reinforcement learning as an alternative solution to learn when to trigger proactive process adaptations based on the predictions and their reliability at run time. Experimental results for three public data sets indicate that our approach may on average lead to 12.2% lower process execution costs compared to empirical thresholding.
更多查看译文
关键词
Machine learning, Business process monitoring, Prediction, Adaptation, Accuracy, Earliness
AI 理解论文
溯源树
样例
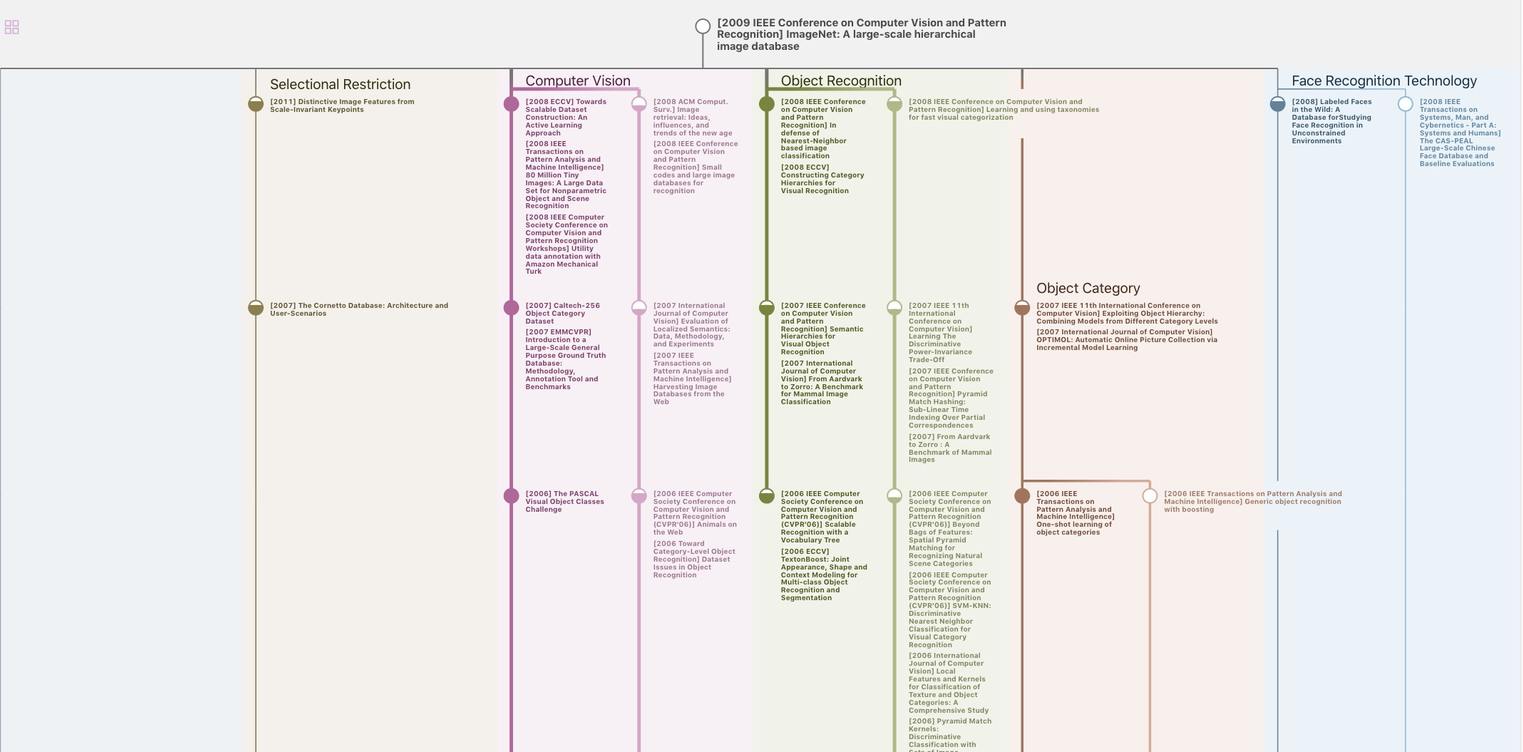
生成溯源树,研究论文发展脉络
Chat Paper
正在生成论文摘要