A Deep Learning Approach To Localization For Navigation On A Miniature Autonomous Blimp
2020 IEEE 16TH INTERNATIONAL CONFERENCE ON CONTROL & AUTOMATION (ICCA)(2020)
摘要
The Georgia Tech Miniature Autonomous Blimp (GT-MAB) needs localization algorithms to navigate to way-points in an indoor environment without leveraging an external motion capture system. Indoor aerial robots often require a motion capture system for localization or employ simultaneous localization and mapping (SLAM) algorithms for navigation. The proposed strategy for GT-MAB localization can be accomplished using lightweight sensors on a weight-constrained platform like the GT-MAB. We train an end-to-end convolutional neural network (CNN) that predicts the horizontal position and heading of the GT-MAB using video collected by an onboard monocular RGB camera. On the other hand, the height of the GT-MAB is estimated from measurements through a time-of-flight (ToF) single-beam laser sensor. The monocular camera and the single-beam laser sensor are sufficient for the localization algorithm to localize the GT-MAB in real time, achieving the averaged 3D positioning errors to be less than 20 cm, and the averaged heading errors to be less than 3 degrees. With the accuracy of our proposed localization method, we are able to use simple proportional-integral-derivative controllers to control the GT-MAB for waypoint navigation. Experimental results on the waypoint following are provided, which demonstrates the use of a CNN as the primary localization method for estimating the pose of an indoor robot that successfully enables navigation to specified waypoints.
更多查看译文
关键词
navigation,Georgia Tech Miniature Autonomous Blimp,simultaneous localization and mapping algorithm,external motion capture system,GT-MAB localization,time-of-flight single-beam laser sensor,primary localization method
AI 理解论文
溯源树
样例
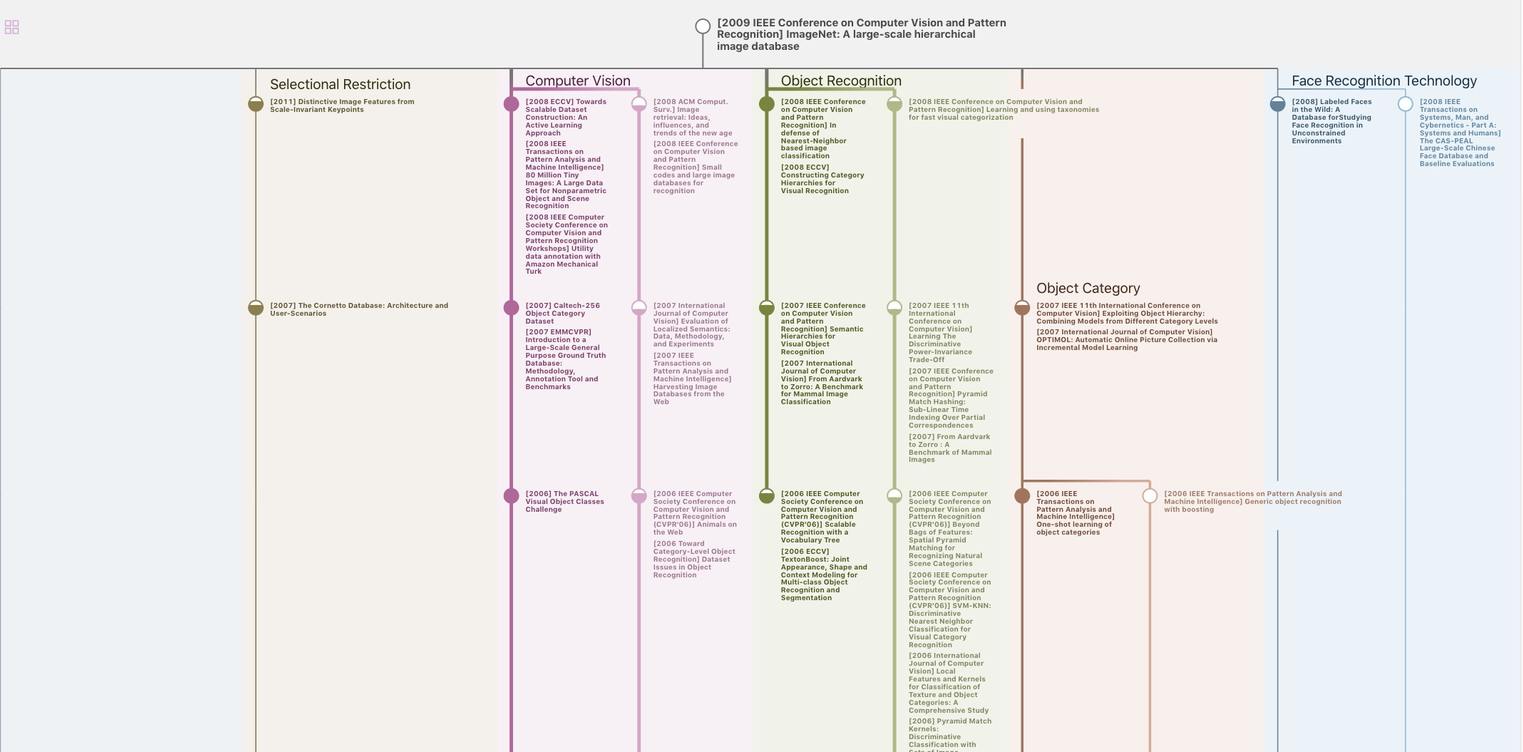
生成溯源树,研究论文发展脉络
Chat Paper
正在生成论文摘要