Lung Cancer Detection And Characterisation Through Genomic And Radiomic Biomarkers
2020 INTERNATIONAL JOINT CONFERENCE ON NEURAL NETWORKS (IJCNN)(2020)
摘要
Medical image bio-markers of cancer are expected to improve patient care through advances in precision medicine. Compared to genomic bio-markers, bio-markers obtained directly from medical images provide the advantages of being a non-invasive procedure, and characterizing a heterogeneous tumor in its entirety, as opposed to limited tissue available for biopsy. In this paper, with the aim to demonstrate that non-invasive features can obtain better performances if compared to invasive ones in lung cancer detection and characterisation, we propose a method to discriminate between different lung cancers (i.e., Adenocarcinoma and Squamous Cell Carcinoma) by adopting both invasive (genomic) and non-invasive (radiomic) bio-markers, by building supervised machine learning models exploiting both invasive and non-invasive features. Experiments on a data-set of 130 patients show that radiomic bio-markers obtain better performances (with an f-measure equal to 0.993) if compared to the ones obtained by considering genomic ones (reaching an f-measure equal to 0.929) in lung cancer detection and characterisation.
更多查看译文
关键词
radiomics, genomics, lung cancer, MRI, machine learning, neural network, supervised learning, classification
AI 理解论文
溯源树
样例
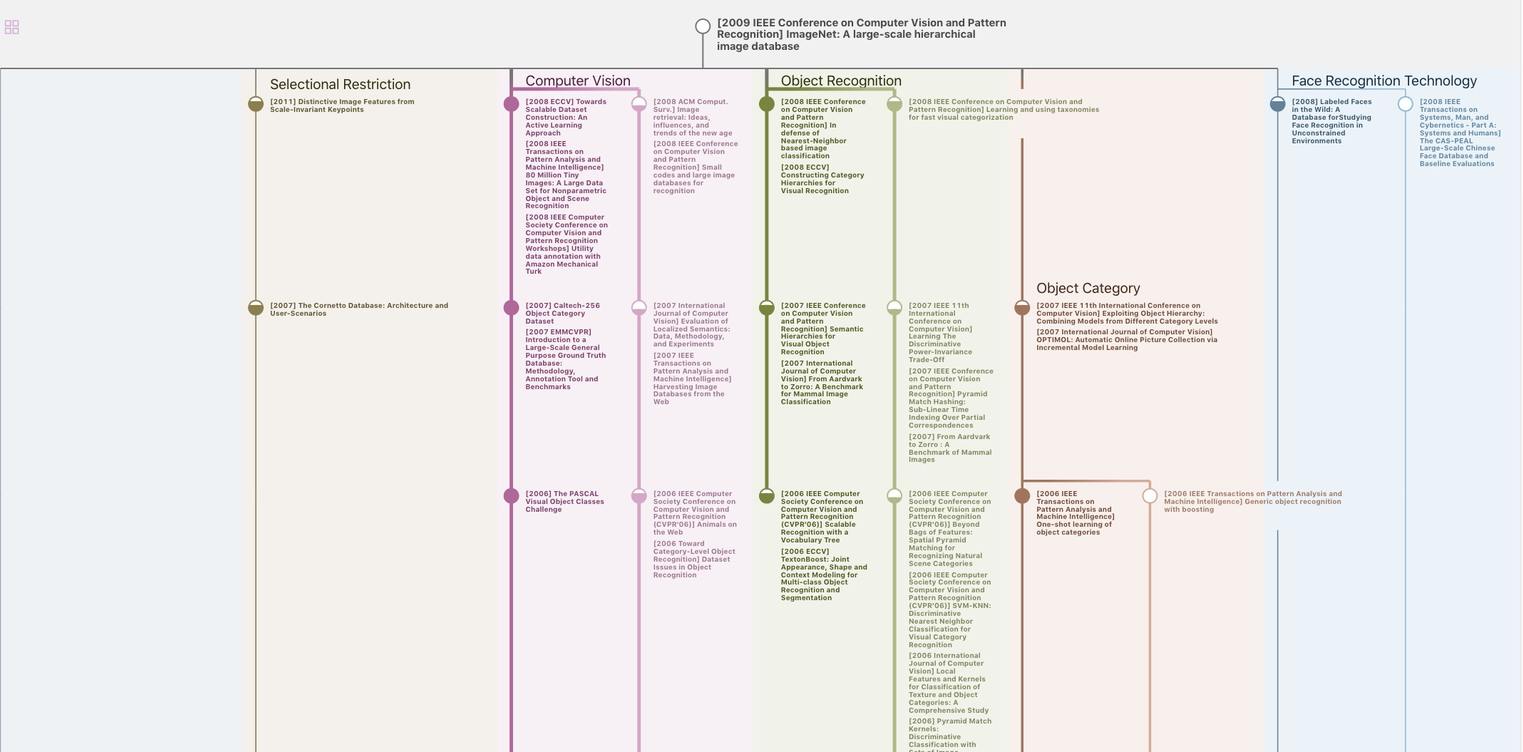
生成溯源树,研究论文发展脉络
Chat Paper
正在生成论文摘要