A Large-Scale Mapping Method Based on Deep Neural Networks Applied to Self-Driving Car Localization.
IJCNN(2020)
摘要
We propose a new approach for real time inference of occupancy maps for self-driving cars using deep neural networks (DNN) named NeuralMapper. NeuralMapper receives LiDAR sensor data as input and generates as output the occupancy grid map around the car. NeuralMapper infers the probability of each grid map cell from one of the three following classes: Occupied, Free and Unknown. The system was tested with two datasets and achieved an average accuracy of 76.48% and 73.81%. We also evaluated our approach for localization purposes in a self-driving car and most of the localization pose errors were less than 0.20m with an RMSE of 0.28 which are close to the results in the literature for methods using other grid mapping approaches.
更多查看译文
关键词
self-driving cars, mapping, deep neural networks
AI 理解论文
溯源树
样例
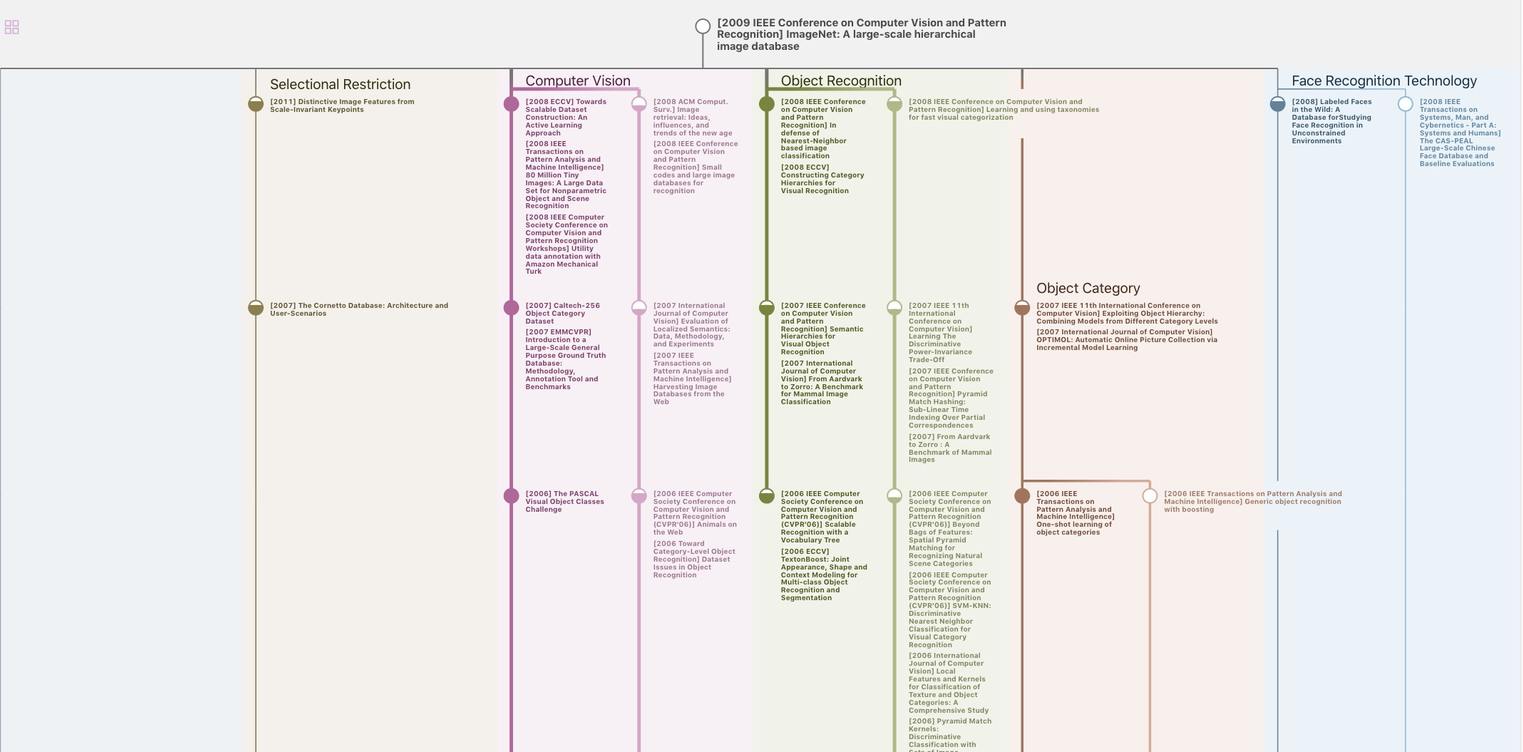
生成溯源树,研究论文发展脉络
Chat Paper
正在生成论文摘要