Learning Single-View Object Reconstruction With Scaling Volume-View Supervision
2020 INTERNATIONAL JOINT CONFERENCE ON NEURAL NETWORKS (IJCNN)(2020)
摘要
Producing a 3D voxel from a single view by deep learning-based methods has garnered increasing attention. Several state-of-the-art works introduce the recurrent neural network(RNN) to fuse features and generate full volumetric occupancy. However, the inputs are unable to be fully exploited to improve the reconstruction due to long-term memory loss. And most of the works have considered using 3D supervision for the whole optimization to recover the full volume, but lack detailed silhouette supervision to refine the reconstruction process. To address these issues, an end-to-end object reconstruction network with scaling volume-view supervision is proposed. We introduce an auto-encoder 3D volume predicting network that takes a single arbitrary image as input and outputs a voxel occupancy grid. And a scaling volume-view supervision module, which uses up-sampling to zoom errors and increase penalties, is leveraged to improve both the global and local optimization. Extensive experimental analysis on ShapeNet dataset shows that our network has superior performance when the scaling volume view supervision is involved and the deep residual module boosts the reconstruction performance and speeds up the optimization effectively.
更多查看译文
关键词
single-view object reconstruction,deep learning-based methods,state-of-the-art works,long-term memory loss,detailed silhouette supervision,reconstruction process,end-to-end object reconstruction network,auto-encoder 3D,single arbitrary image,voxel occupancy grid,scaling volume-view supervision module,scaling volumeview supervision,reconstruction performance
AI 理解论文
溯源树
样例
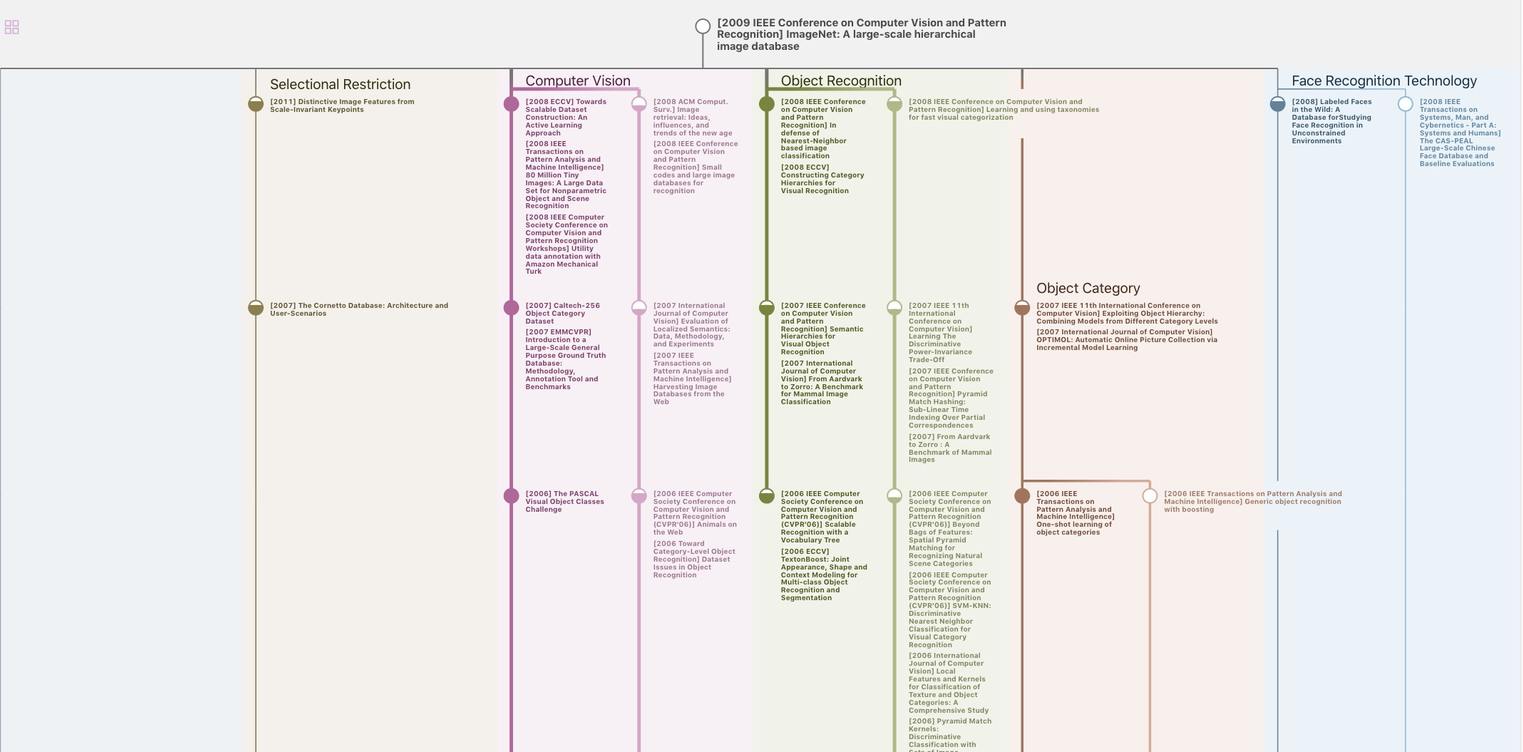
生成溯源树,研究论文发展脉络
Chat Paper
正在生成论文摘要