Syntax-Informed Interactive Neural Machine Translation
2020 INTERNATIONAL JOINT CONFERENCE ON NEURAL NETWORKS (IJCNN)(2020)
摘要
In interactive machine translation (MT), human translators correct errors in automatic translations in collaboration with the MT systems, and this is an effective way to improve productivity gain in translation. Phrase-based statistical MT (PB-SMT) has been the mainstream approach to MT for the past 30 years, both in academia and industry. Neural MT (NMT), an end-to-end learning approach to MT, represents the current state-of-the-art in MT research. The recent studies on interactive MT have indicated that NMT can significantly outperform PB-SMT.In this work, first we investigate the possibility of integrating lexical syntactic descriptions in the form of supertags into the state-of-the-art NMT model, Transformer. Then, we explore whether integration of supertags into Transformer could indeed reduce human efforts in translation in an interactive-predictive platform. From our investigation we found that our syntaxaware interactive NMT (INMT) framework significantly reduces simulated human efforts in the French-to-English and Hindi-to-English translation tasks, achieving a 2.65 point absolute corresponding to 5.65% relative improvement and a 6.55 point absolute corresponding to 19.1% relative improvement, respectively, in terms of word prediction accuracy (WPA) over the respective baselines.
更多查看译文
关键词
machine translation, neural machine translation, interactive neural machine translation
AI 理解论文
溯源树
样例
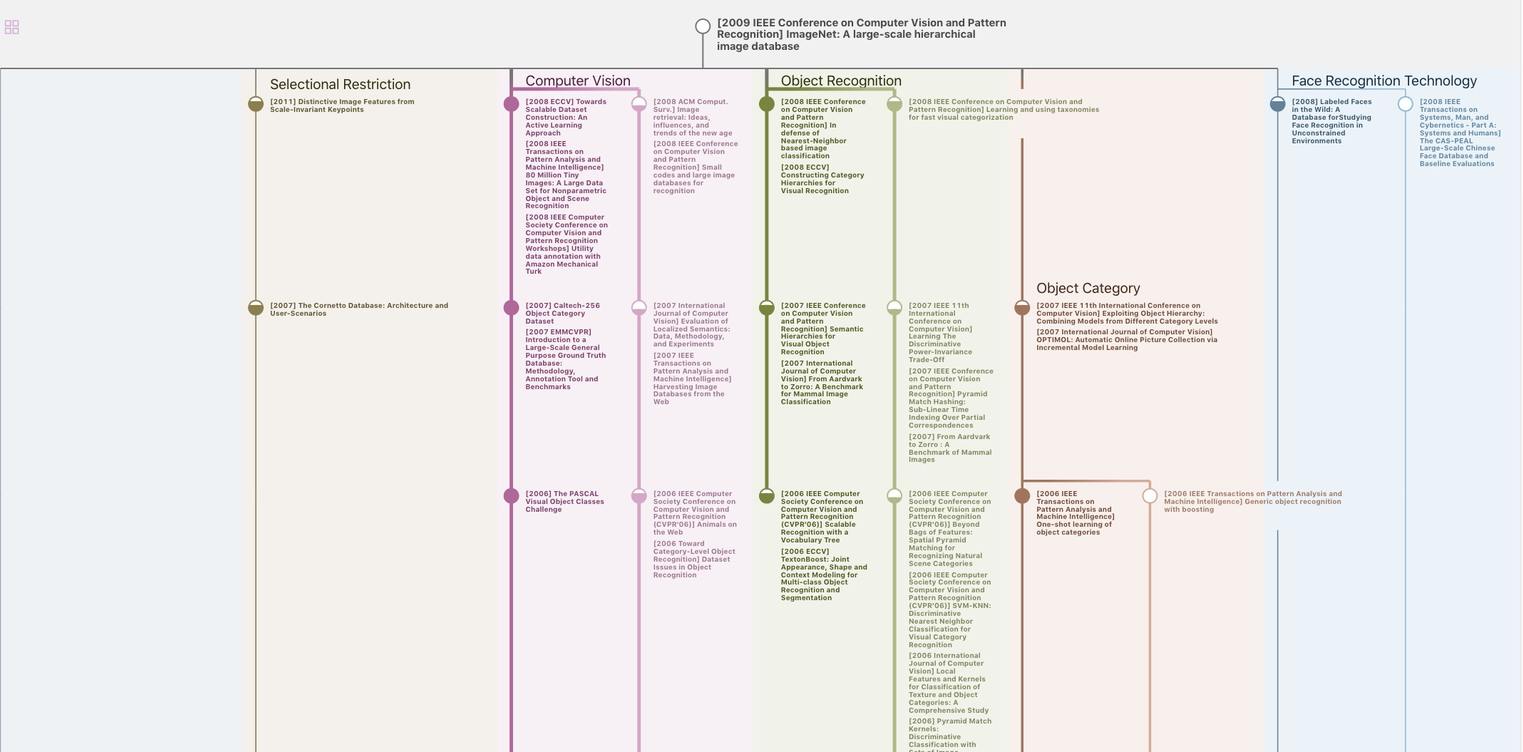
生成溯源树,研究论文发展脉络
Chat Paper
正在生成论文摘要