Multiple Timescale And Gated Mechanisms For Action And Language Learning In Robotics
2020 INTERNATIONAL JOINT CONFERENCE ON NEURAL NETWORKS (IJCNN)(2020)
摘要
Recurrent Neural Network (RNN) have been used for sequence-related learning tasks, such as language and action, in the field of cognitive robotics. Gated mechanisms used in LSTM and GRU perform well in remembering long-term dependency. But to better mimic the neural dynamics in cognitive processes, the Multiple Time-scales (MT) RNN uses a hierarchical organization of memory updates which is similar to human cognition. Since the MT feature is typically used with a vanilla RNN or different gated mechanisms, its effect on the updates and training is still not fully uncovered. Therefore, we conduct a comparative experiment on two MT recurrent neural network models, i.e. the Multiple Time-Scale Recurrent Neural Network (MTRNN) and the Multiple Time-Scale Gated Recurrent Unit (MTGRU), for action sequence learning in robotics. The experiment shows that the MTRNN model can be used in learning tasks with low requirements for learning of long-term dependency due to its low computation. On the other hand, the MTGRU model is appropriate for learning the long-term dependency. Furthermore, because of the duplicated feature of the MT and the GRU feature, we also propose a simplified MTGRU model, named Multiple Time-scale Single-Gate Recurrent Unit (MTSRU) which could reduce computational cost while it achieves the similar performance as the original version.
更多查看译文
关键词
cognitive robotics, Neural Network, Multiple Time-scale, MTSRU
AI 理解论文
溯源树
样例
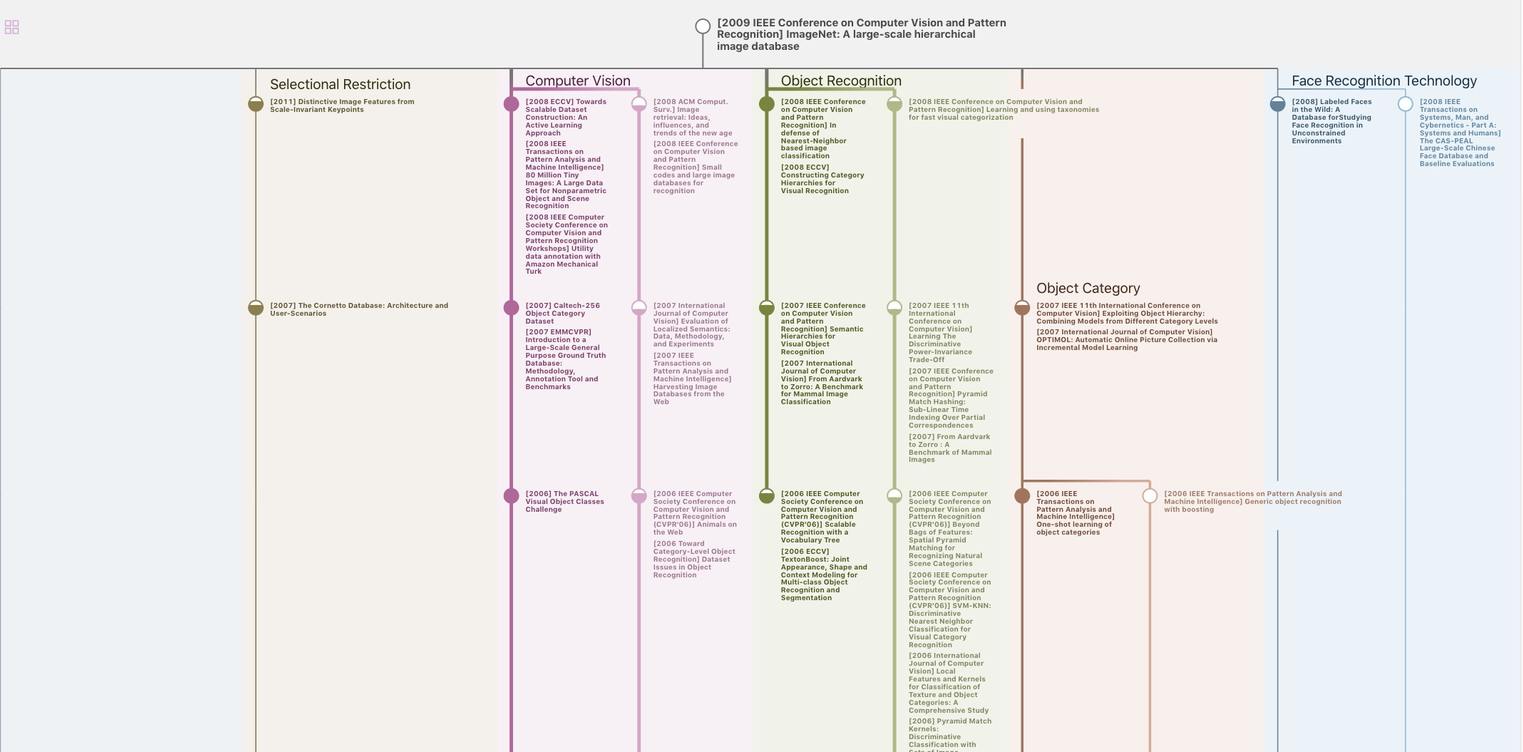
生成溯源树,研究论文发展脉络
Chat Paper
正在生成论文摘要