Learning & Visualizing Genomic Signatures Of Cancer Tumors Using Deep Neural Networks
2020 INTERNATIONAL JOINT CONFERENCE ON NEURAL NETWORKS (IJCNN)(2020)
摘要
Deep learning for medical diagnosis using genomics is extremely challenging given the high dimensionality of the data and lack of sufficient patient samples. Another challenge is that deep models are conceived as black boxes without much interpretation on how these complex models make predictions. We propose a deep transfer learning framework for cancer diagnosis with the capability of learning the sequence of DNA and RNA in cancer cells and identifying genetic changes that alter cell behavior and cause uncontrollable growth and malignancy. We design a new Convolutional Neural Network architecture with capabilities of learning the genomic signatures of whole-transcriptome gene expressions collected from multiple tumor types covering multiple organ sites. We demonstrate how our trained model can function as a comprehensive multi-tissue cancer classifier by using transfer learning to build classifiers for tumors lacking sufficient human samples to be trained independently. We introduce visualization procedures to provide more biological insight on how our model is learning genomic signatures and accurately making predictions across multiple cancer tissue types.
更多查看译文
关键词
Cancer classification, Convolutional neural networks, Deep learning, Gene expressions, RNA sequencing
AI 理解论文
溯源树
样例
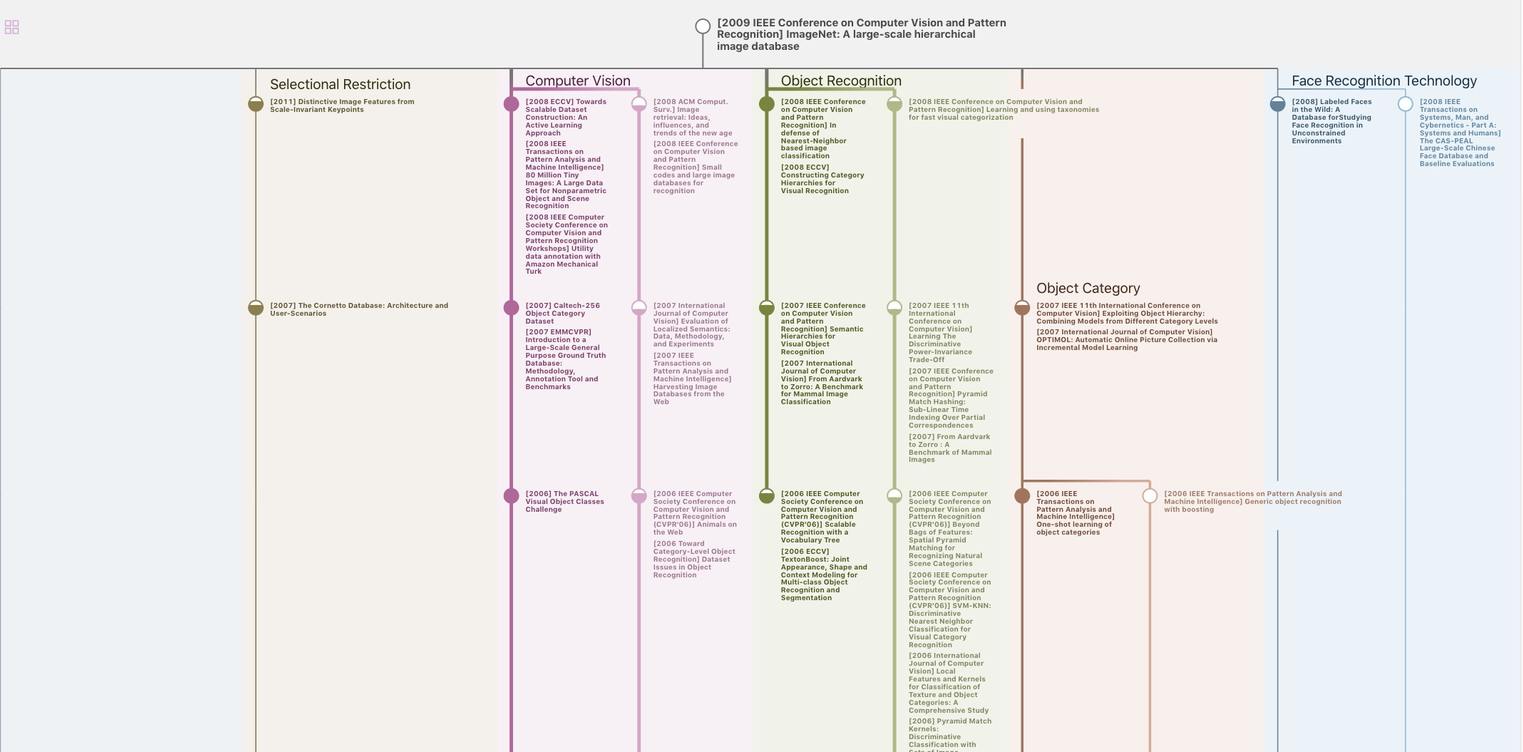
生成溯源树,研究论文发展脉络
Chat Paper
正在生成论文摘要