Unsupervised Embeddings For Categorical Variables
2020 INTERNATIONAL JOINT CONFERENCE ON NEURAL NETWORKS (IJCNN)(2020)
摘要
Real-world data sets often contain both continuous and categorical variables yet most popular machine learning methods cannot by default handle both data types. This creates the need for researchers to transform their data into a continuous format. When no prior information is available, the most widely applied methods are simple ones such as one hot encoding. However, they ignore many possible sources of information, in particular, categorical dependencies, which could enrich the vector representations. We investigate the effect of natural language processing techniques for learning continuous word-vector representations on categorical variables. We show empirically that the learned vector representations of the categorical variables capture information about the variables themselves and their dependencies with other variables similar to how word embeddings capture semantic and syntactic information. We also show that machine learning models using unsupervised categorical embeddings are competitive with supervised embeddings, and outperform them when fine-tuned, on various classification benchmark data sets.
更多查看译文
关键词
Machine Learning, Categorical Variables, Embedding Methods
AI 理解论文
溯源树
样例
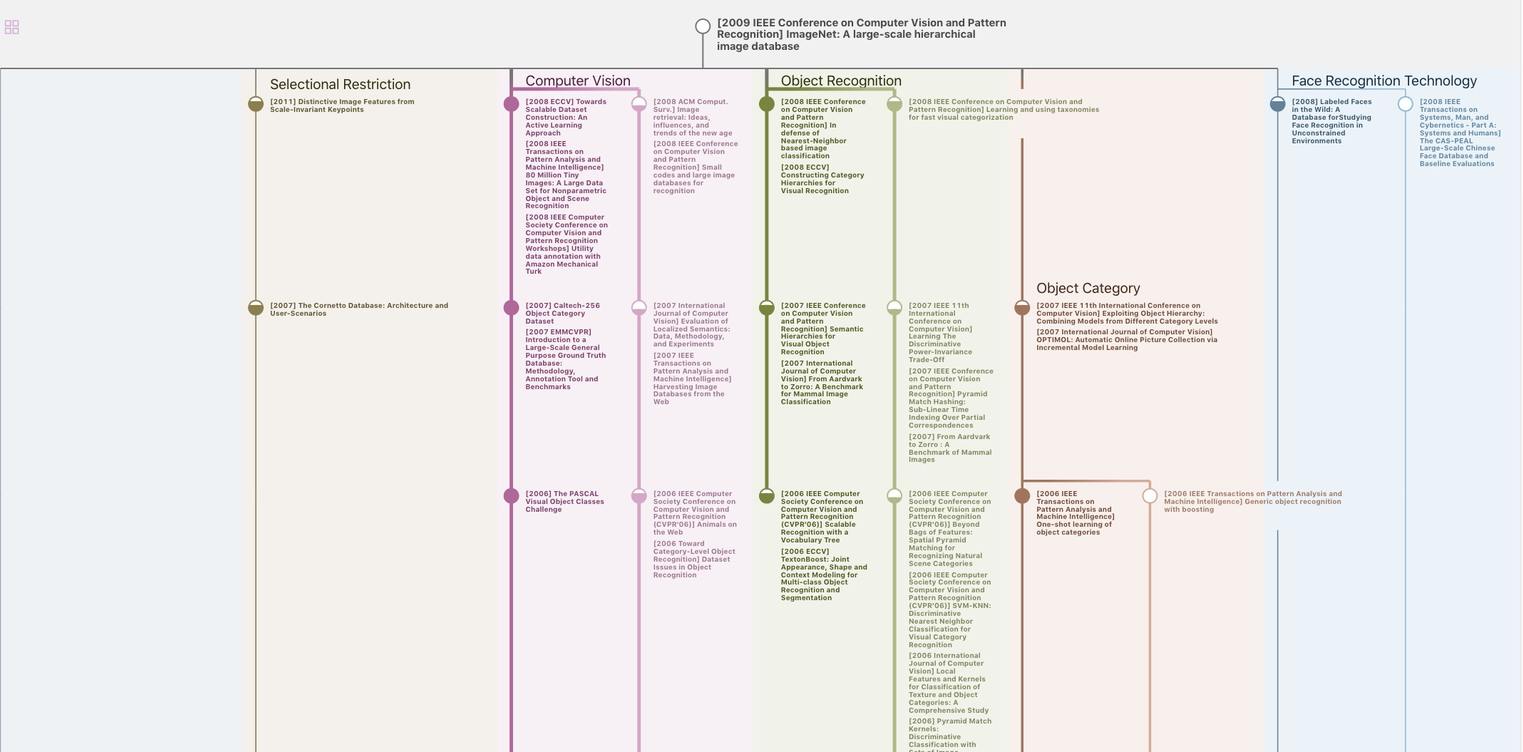
生成溯源树,研究论文发展脉络
Chat Paper
正在生成论文摘要