A Dual Network Solution (Dns) For Lag-Free Time Series Forecasting
2020 INTERNATIONAL JOINT CONFERENCE ON NEURAL NETWORKS (IJCNN)(2020)
摘要
When it comes to time series forecasting, lag in the predicted sequence can be a predominant issue. Unfortunately, this is often overlooked in most of the time series literature as this does not contribute to a high prediction error (i.e. MSE). However, it leads to a rather poor forecast in terms of movement prediction in time series. In this article, we tackle this basic problem with a novel trend driven mechanism. Trend, defined as the inherent pattern of the data, is extracted here and utilized next to perform a lag-free forecasting. We propose a generic and light Dual Network Solution (DNS), where the first network predicts the trend and the second network utilizes that predicted trend along with its historical information to capture the dynamical behavior of the time series efficiently. DNS exhibits a substantially improved (approximate to 10% better) performance compared to more complex and resource-intensive state-of-the-art algorithms in large scale regression problems. Apart from the traditional Mean Squared Error (MSE), we also propose a new Movement Prediction Metric or MPM (for detection of lag in time series) as a new complementary performance metric to evaluate the efficacy of DNS better.
更多查看译文
关键词
Lag in time series prediction, Dual network solution, Movement prediction, Lag free time series prediction
AI 理解论文
溯源树
样例
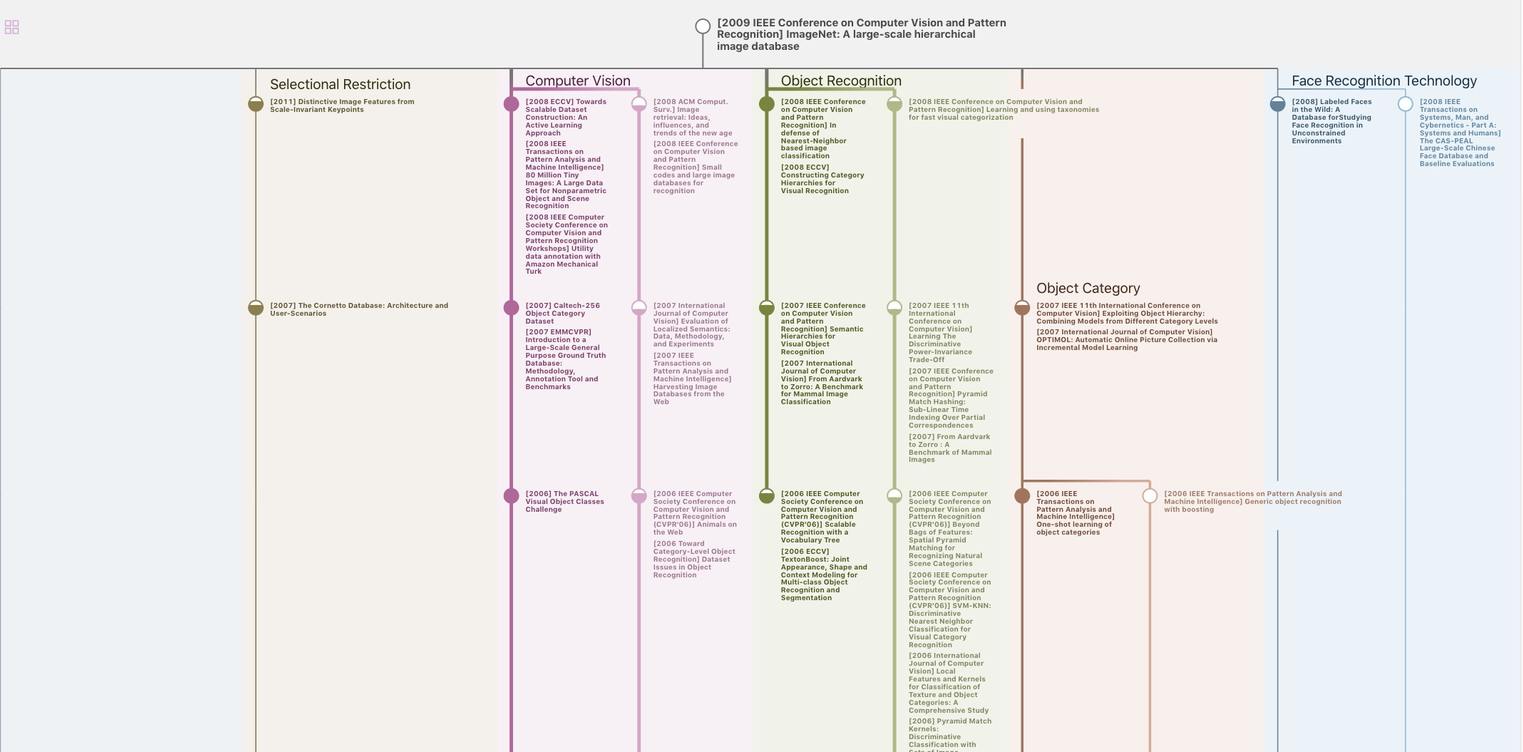
生成溯源树,研究论文发展脉络
Chat Paper
正在生成论文摘要