Drg2vec: Learning Word Representations From Definition Relational Graph
2020 INTERNATIONAL JOINT CONFERENCE ON NEURAL NETWORKS (IJCNN)(2020)
摘要
Even with larger and larger text data available, encoding linguistic knowledge into word embeddings directly from corpora is still difficult. The most intuitive way to incorporate knowledge into word embeddings is to use external resources, such as the largest datasource for describing words - natural language dictionaries. However, previous methods usually neglect the recursive nature of dictionaries and cannot capture the high-order relation. In this paper, we present DRG2vec, a novel and efficient approach for learning word representations, which exploits the inherent recursiveness of dictionaries by modeling the whole dictionary as a homogeneous graph based on the co-occurrence of entry and word in the definition. Moreover, a tailormade sampling strategy is introduced to generate word sequences from the definition relational graph, and then, the generated sequences are fed to the Skip-gram model with semantic negative sampling for word representation learning. Extensive experiments on sixteen benchmark datasets show that leveraging the recursive dictionary graph indeed achieves better performance than other state-of-the-art methods, especially exhibits a weighted average improvement of 8.6% in the word similarity task.
更多查看译文
关键词
Word embedding, Word similarity, Dictionary, Graph Representation, Text Classification
AI 理解论文
溯源树
样例
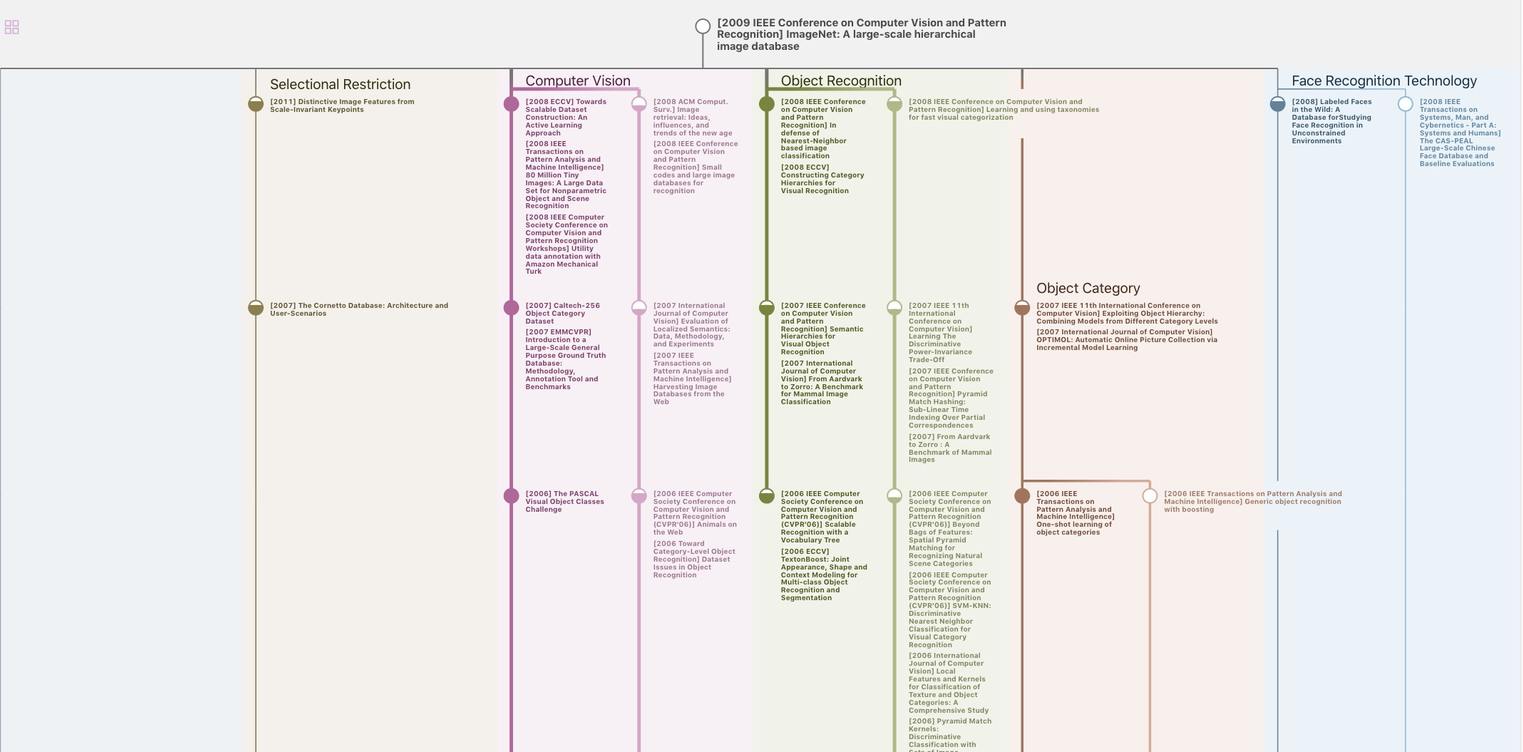
生成溯源树,研究论文发展脉络
Chat Paper
正在生成论文摘要