Grammatical Error Detection With Self Attention By Pairwise Training
2020 INTERNATIONAL JOINT CONFERENCE ON NEURAL NETWORKS (IJCNN)(2020)
摘要
Automatic grammatical error detection system is useful for language learners to identify whether the texts written by themselves have errors. Researches have paid more attention on different models to deal with this task, various approaches have been proposed and better results have been achieved compare with rules base methods. It is known that artificially generated incorrect texts can further improve the performance of grammatical error correction and pairwise training is necessary for many recommendation algorithms. We incorporating these two techniques together to solve the error detection task with pre-trained words embeddings from BERT in this paper. It is the first work that adopt pairwise training with pairs of samples to detect grammatical errors since all previous work were training models with batches of samples piontwisely. Pairwise training is useful for models to capture the differences within the pair of samples, which are intuitive useful for model to distinguish errors. Extensive experiments have been carried out to prove the effectiveness of pairwise training mechanism. The experimental results shown that the proposed method can achieve the state of the art performance on four different standard benchmarks. With the help of data augmentation and filtering, the value of F0:5 can be further improved. The overall improvements among the four test set are around 2.5% which demonstrate the generality of pairwise training for datasets from differen domains.
更多查看译文
关键词
pairwise training, grammatical error detection, BERT, data augmentation
AI 理解论文
溯源树
样例
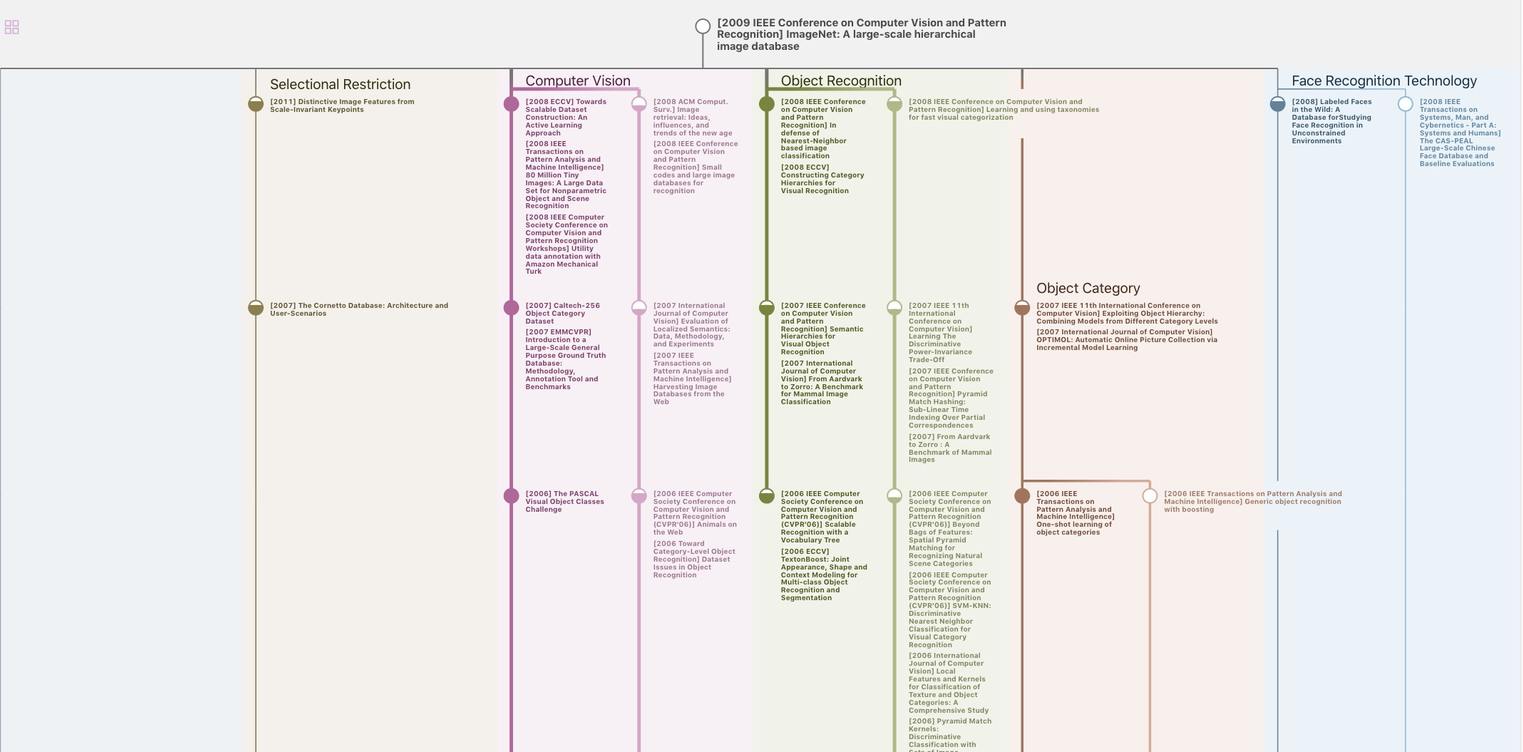
生成溯源树,研究论文发展脉络
Chat Paper
正在生成论文摘要