Leveraging The Manycore Architecture Of The Loihi Spiking Processor To Perform Quasi-Complete Constraint Satisfaction
2020 INTERNATIONAL JOINT CONFERENCE ON NEURAL NETWORKS (IJCNN)(2020)
摘要
In many cases, low power autonomous systems need to make decisions extremely efficiently. However, as a problem space becomes more complex, finding a solution quickly becomes nearly impossible using traditional computing methods. Thus, in this work we show that constraint satisfaction problems (CSPs) can be solved quickly and efficiently using spiking neural networks. Constraint satisfaction is a general problem solving technique that can be applied to a large number of different applications. To demonstrate the validity of this algorithm, we show successful execution of the Boolean satisfiability problem (SAT) on the Intel Loihi spiking neuromorphic research processor. In many cases, constraint satisfaction problems have solution sets as opposed to single solutions. Therefore, the manycore architecture of the Loihi chip is used to parallelize the solution finding process, leading to a quasi-complete solution set generated at extreme efficiency (dynamic energy as low as 8 micro joules per solution). Power consumption in this spiking processor is due primarily to the propagation of spikes, which are the key drivers of data movement and processing. Thus, the proposed SAT algorithm was customized for spiking neural networks to achieve the greatest efficiency gains. To the best of our knowledge, the work in this paper exhibits the first implementation of constraint satisfaction on a low power embedded neuromorphic processor capable of generating a solution set. In general, we show that embedded spiking neuromorphic hardware is capable parallelizing the constraint satisfaction problem solving process to yield extreme gains in terms of time, power, and energy.
更多查看译文
关键词
Spiking neural networks, Intel Loihi, neuromorphic hardware, constraint satisfaction, SAT
AI 理解论文
溯源树
样例
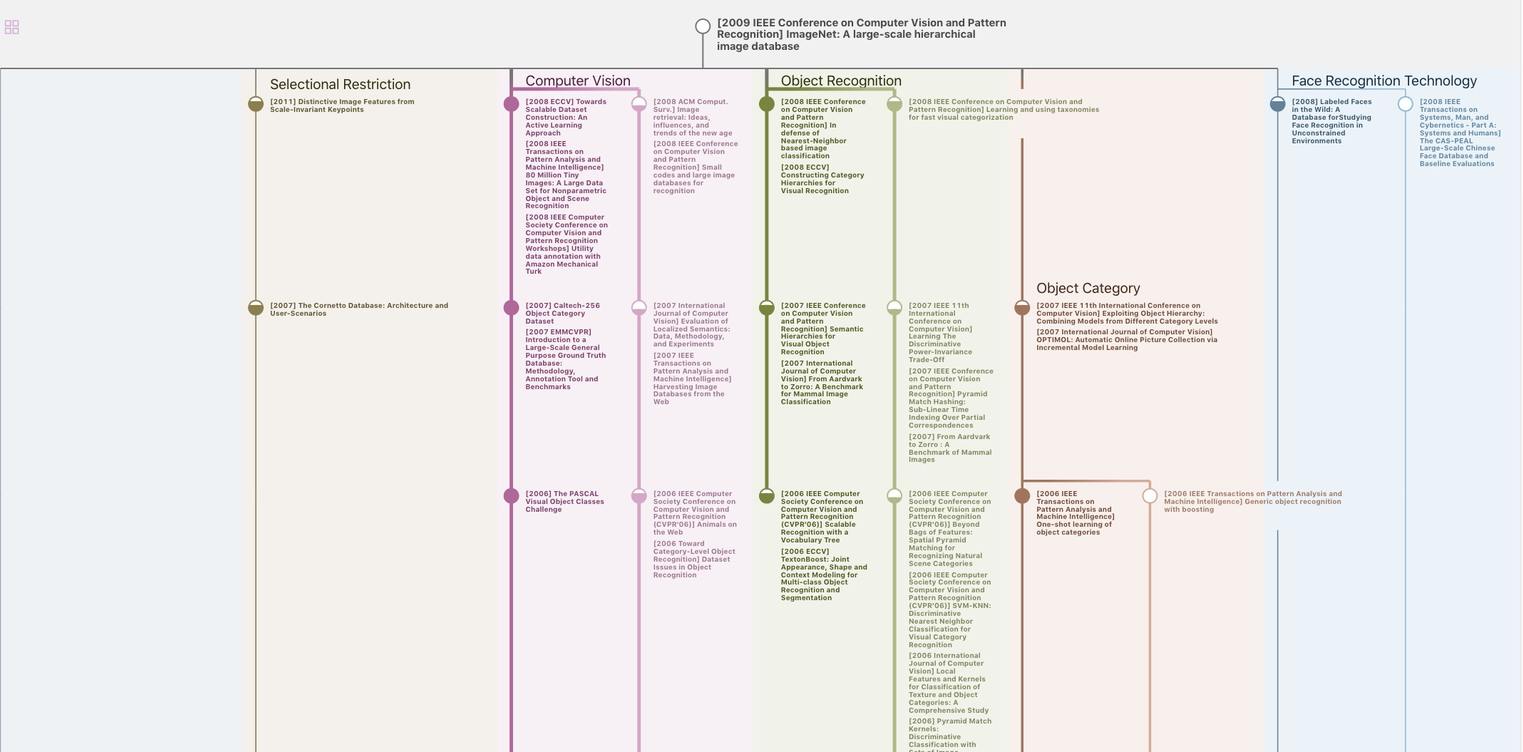
生成溯源树,研究论文发展脉络
Chat Paper
正在生成论文摘要