A Light-Weight Crowdsourcing Aggregation In Privacy-Preserving Federated Learning System
2020 INTERNATIONAL JOINT CONFERENCE ON NEURAL NETWORKS (IJCNN)(2020)
摘要
Federated Machine Learning (FML) sheds light on secure distributed machine learning. However, generic FML methods may lead to privacy-leakage through the sharing of training information of individual models and have relatively poor performance when the training datasets for individual models are biased and diversified. This is a problem in combining models trained in different scenarios of IoT devices since the available training datasets are usually limited and biased. To tackle this problem, we propose a novel approach to precisely ensemble results from different models in distributed edge devices. Instead of passing the training information of individual models around that requires a relatively large amount of bandwidth and compromises data privacy, we suggest employing a trusted central agent that only collects different inference results from edge devices. Then based on a limited amount of labeled data, the agent runs a designed statistical iterative crowdsourcing algorithm to combine results for a more accurate aggregated prediction towards a user query. Our proposed system model, "Privacy-Preserving Federated Learning System", together with our light-weight Secure Crowdsourcing Aggregation (SC-Agg) algorithm, provide a more accurate prediction for outside queries at little cost without any prior knowledge of what query will be submitted. We experimentally verify that in our system, SC-Agg consistently outperforms the majority voting method and the best performing model of the ensemble in all testing scenarios. We believe that SC-Agg fits the real-world IoT applications better than other methods, such as the vanilla majority voting, for its robustness and better performance.
更多查看译文
关键词
Federated Learning, Crowdsourcing, Privacy, IoT
AI 理解论文
溯源树
样例
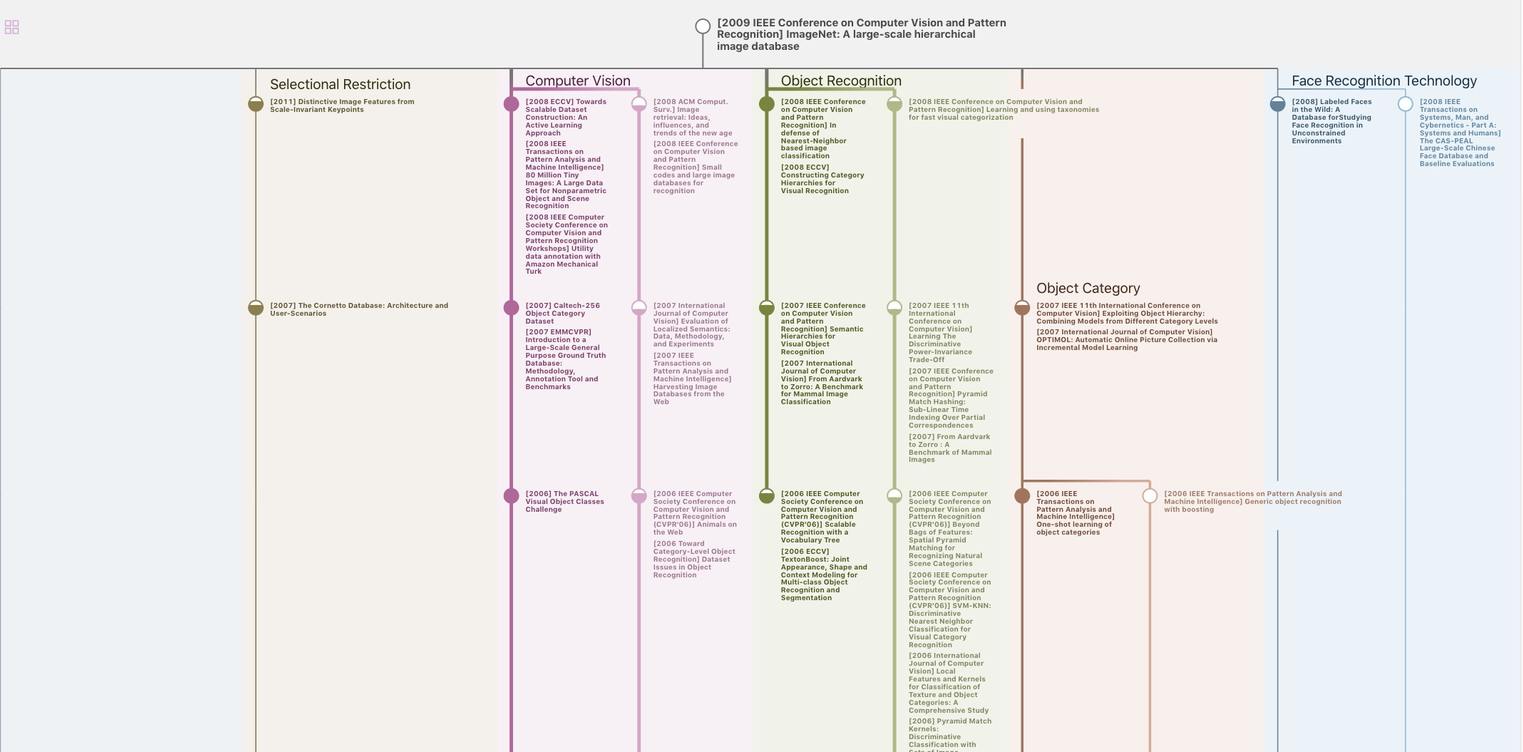
生成溯源树,研究论文发展脉络
Chat Paper
正在生成论文摘要