Sequential Possibilistic Local Information One-Means Clustering For Image Segmentation
2020 IEEE International Conference on Fuzzy Systems (FUZZ-IEEE)(2020)
摘要
Clustering has long been applied to the problem of image segmentation. Because of spatial connectivity constraints, several approaches have been proposed to incorporate local consistency into image segmentation by clustering. One popular method, the fuzzy local information c-means (FLICM) has been shown to produce good segmentation results. Like the fuzzy cmeans (FCM) from which it is derived, FLICM requires that pixels "share" memberships across clusters, that is, the memberships of a pixel across all clusters need to sum to one. The possibilistic c-means (PCM) clustering was introduced to relax the membership sum-to-one constraint of the FCM, and has found a place in the clustering universe, particularly in those situations where the data contains outliers, is noisy, or highly overlapped. This paper extends the structure of FLICM to possibilistic versions for image segmentation. Two approaches are proposed. The first, called possibilistic local information cmeans (PLICM) inserts the local information term of FLICM into the basic PCM model. PLICM, like PCM, can produce coincident cluster centers. Recently, a sequential application of PCM (with c = 1) has been developed to mitigate negative effects of the co-incident cluster formation. Three algorithms form the family of sequential possibilistic 1-means (SP1M). These algorithms are extended to account for local information in image segmentation (SPLI1M). After development of the approach, experiments are performed on images which show that the SPLI1M family has superior performance in image segmentation over FLICM, PLICM and other clustering algorithms that don't combine local spatial information of the image.
更多查看译文
关键词
clustering,possibilistic c-means,image segmentation,spatial constraints
AI 理解论文
溯源树
样例
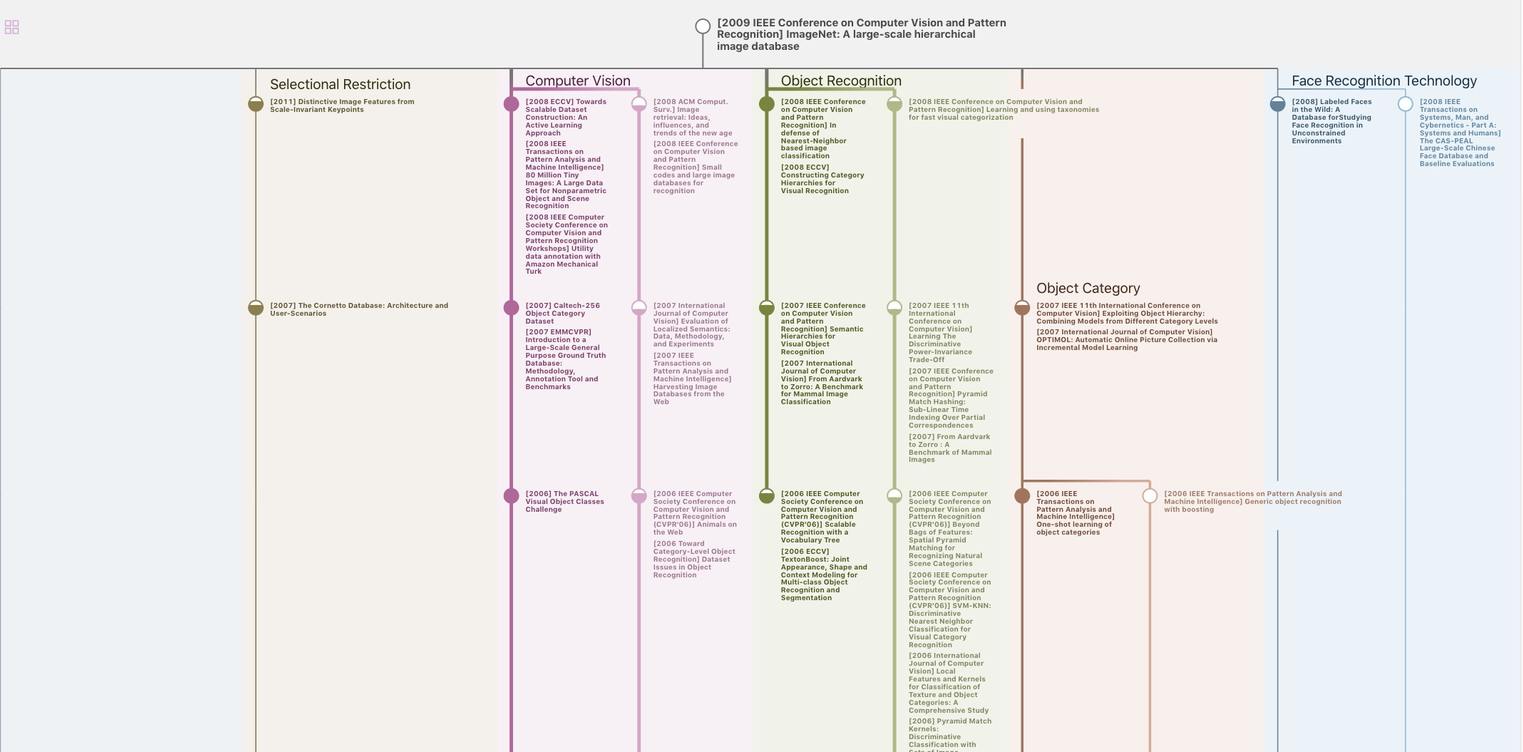
生成溯源树,研究论文发展脉络
Chat Paper
正在生成论文摘要