Bottom-up Discovery of Context-aware Quality Constraints for Heterogeneous Knowledge Graphs.
KDIR(2020)
摘要
As knowledge graphs are getting increasingly adopted, the question of how to maintain the validity and accuracy of our knowledge becomes ever more relevant. We introduce context-aware constraints as a means to help preserve knowledge integrity. Context-aware constraints offer a more fine-grained control of the domain onto which we impose restrictions. We also introduce a bottom-up anytime algorithm to discover context-aware constraint directly from heterogeneous knowledge graphs-graphs made up from entities and literals of various (data) types which are linked using various relations. Our method is embarrassingly parallel and can exploit prior knowledge in the form of schemas to reduce computation time. We demonstrate our method on three different datasets and evaluate its effectiveness by letting experts on knowledge validation and management assess candidate constraints in a real-world knowledge validation use case. Our results show that overall, context-aware constraints are to an extent useful for knowledge validation tasks, and that the majority of the generated constraints are well balanced with respect to complexity.
更多查看译文
关键词
Knowledge Graphs, Data Validation, Data Quality, Constraints, Pattern Mining
AI 理解论文
溯源树
样例
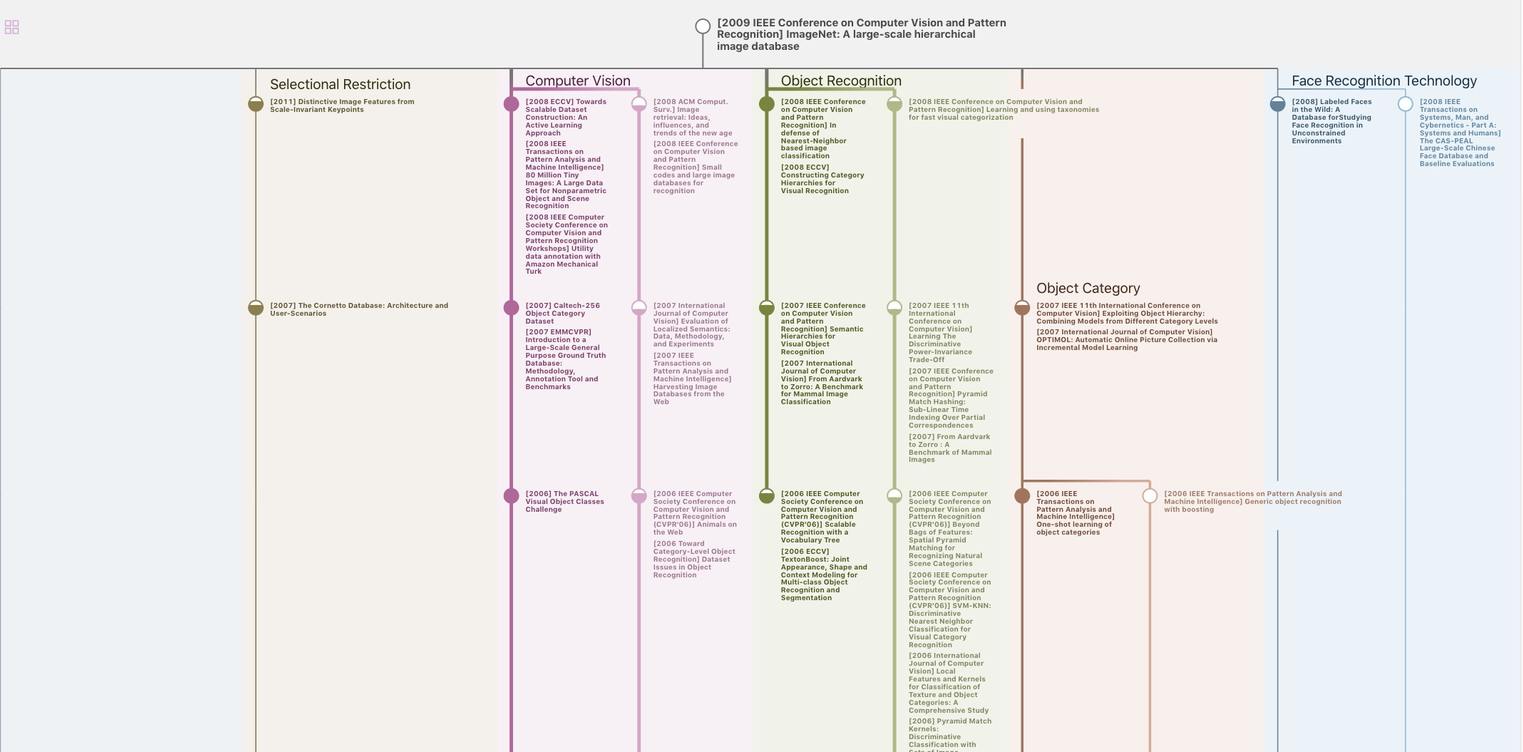
生成溯源树,研究论文发展脉络
Chat Paper
正在生成论文摘要