HeroGRAPH: A Heterogeneous Graph Framework for Multi-Target Cross-Domain Recommendation.
ORSUMRecSys(2020)
摘要
Cross-Domain Recommendation (CDR) is an important task in recommender systems. Information can be transferred from other domains to target domain to boost its performance and relieve the sparsity issue. Most of the previous work is single-target CDR (STCDR), and some researchers recently propose to study dualtarget CDR (DTCDR). However, there are several limitations. These works tend to capture pair-wise relations between domains. They will need to learn much more relations if they are extended to multitarget CDR (MTCDR). Besides, previous CDRworks prefer relieving the sparsity issue by extra information or overlapping users. This leads to a lot of pre-operations, such as feature-engineering and finding common users. In this work, we propose a heterogeneous graph framework for MTCDR (HeroGRAPH). First, we construct a shared graph by collecting users and items from multiple domains. This can obtain cross-domain information for each domain by modeling the graph only once, without any relation modeling. Second, we relieve the sparsity by aggregating neighbors from multiple domains for a user or an item. Then, we devise a recurrent attention to model heterogeneous neighbors for each node. This recurrent structure can help iteratively refine the process of selecting important neighbors. Experiments on real-world datasets show that HeroGRAPH can effectively transfer information between domains and alleviate the sparsity issue. CCS CONCEPTS • Information systems→Collaborative filtering;Recommender systems.
更多查看译文
关键词
Heterogeneous Networks,Signal Processing on Graphs,Context-Aware Recommender Systems,Content-Based Recommendation,Knowledge Graph Embedding
AI 理解论文
溯源树
样例
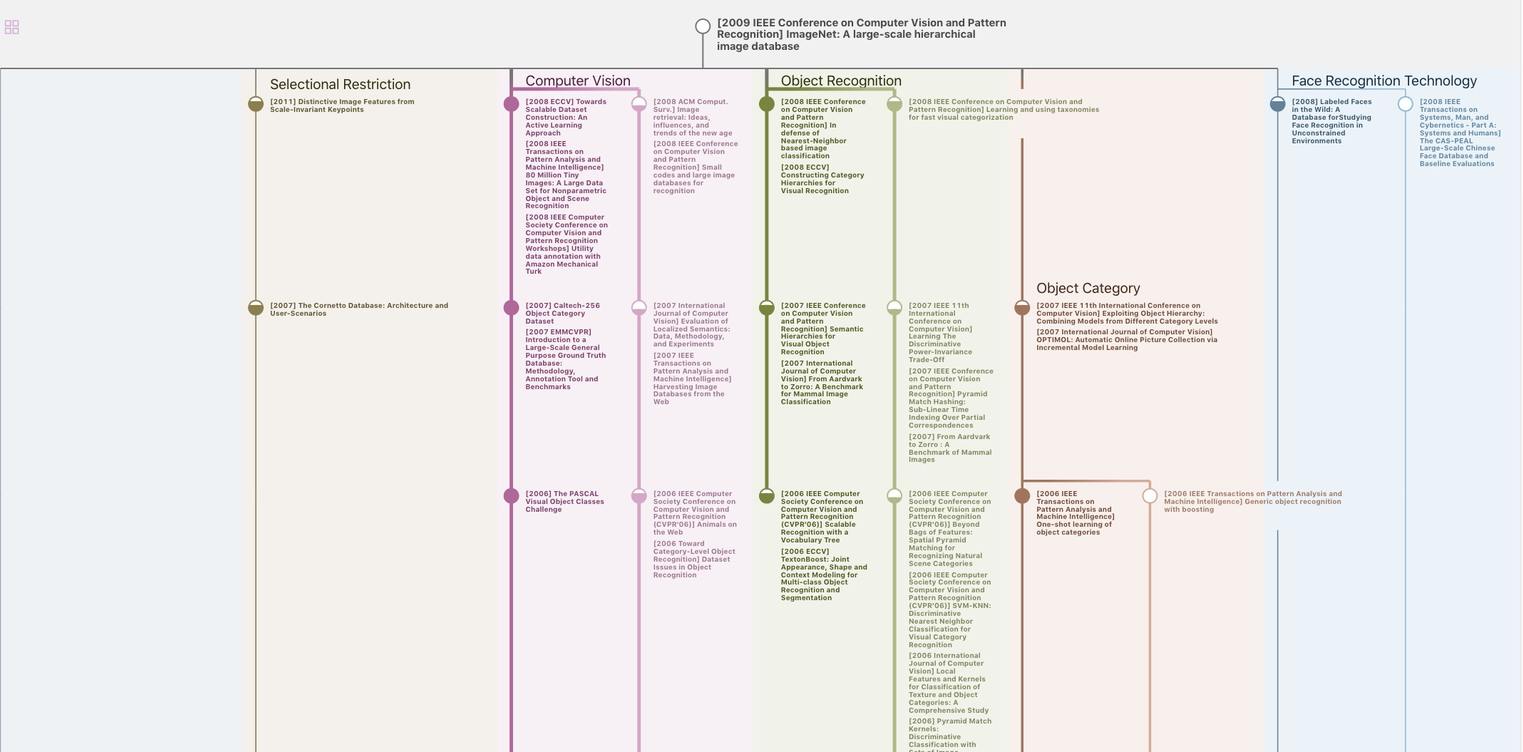
生成溯源树,研究论文发展脉络
Chat Paper
正在生成论文摘要