Multi-Agent Context-Aware Dynamic-Scheduling for Large-scale Processing Networks
2020 25TH IEEE INTERNATIONAL CONFERENCE ON EMERGING TECHNOLOGIES AND FACTORY AUTOMATION (ETFA)(2020)
摘要
Optimally scheduling jobs in processing networks to meet multiple objectives for economic considerations and operational efficiencies has been a hot topic. However, most data-driven methods are rarely applied. One of the critical reasons is that the scheduling policy generated by these methods tends to bias toward the specific environment.In order to better deal with the discrepancy, this work in progress paper presents a context-aware dynamic scheduling method (CADS) that can adaptively select a specific policy based on the on-demand context. The CADS has two components: 1) The evaluation module that evaluates the performances of the policies that are learned from each operational context; 2) The decision-making module that maintains the knowledge of each policy’s performance under each context and constructs the weighted best-fit policy based on the identified context. The promising preliminary result using numerical simulation that demonstrates the effectiveness of CADS is presented. CADS outperforms traditional scheduling methods in various kinds of processing network environments.
更多查看译文
关键词
context-aware, generalization, trustablity, scheduling, processing network
AI 理解论文
溯源树
样例
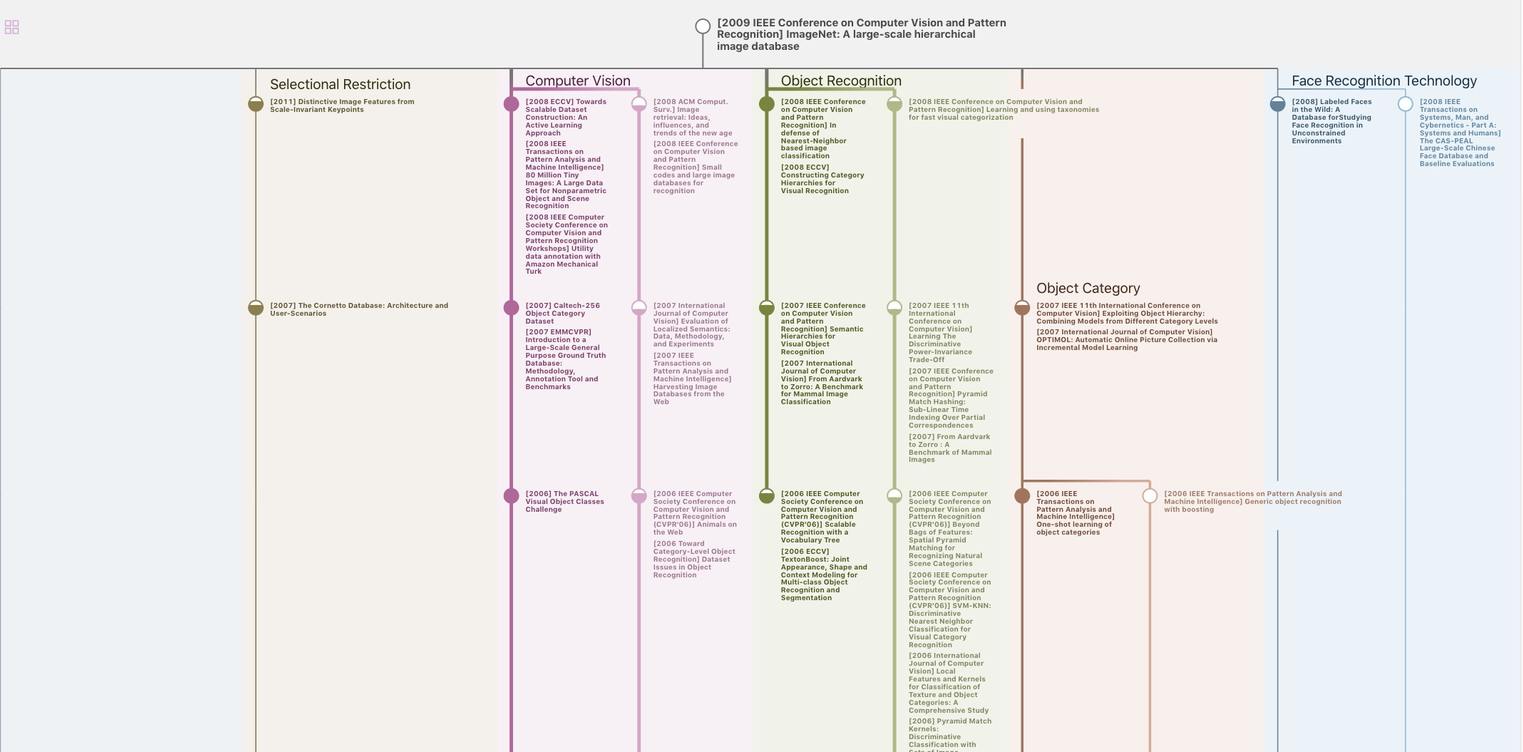
生成溯源树,研究论文发展脉络
Chat Paper
正在生成论文摘要