Performance of in Silico Prediction Tools for the Detection of Germline Copy Number Variations in Cancer Predisposition Genes in 4208 Female Index Patients with Familial Breast and Ovarian Cancer.
Cancers(2021)
摘要
The identification of germline copy number variants (CNVs) by targeted next-generation sequencing (NGS) frequently relies on in silico CNV prediction tools with unknown sensitivities. We investigated the performances of four in silico CNV prediction tools, including one commercial (Sophia Genetics DDM) and three non-commercial tools (ExomeDepth, GATK gCNV, panelcn.MOPS) in 17 cancer predisposition genes in 4208 female index patients with familial breast and/or ovarian cancer (BC/OC). CNV predictions were verified via multiplex ligation-dependent probe amplification. We identified 77 CNVs in 76 out of 4208 patients (1.81%); 33 CNVs were identified in genes other than BRCA1/2, mostly in ATM, CHEK2, and RAD51C and less frequently in BARD1, MLH1, MSH2, PALB2, PMS2, RAD51D, and TP53. The Sophia Genetics DDM software showed the highest sensitivity; six CNVs were missed by at least one of the non-commercial tools. The positive predictive values ranged from 5.9% (74/1249) for panelcn.MOPS to 79.1% (72/91) for ExomeDepth. Verification of in silico predicted CNVs is required due to high frequencies of false positive predictions, particularly affecting target regions at the extremes of the GC content or target length distributions. CNV detection should not be restricted to BRCA1/2 due to the relevant proportion of CNVs in further BC/OC predisposition genes.
更多查看译文
关键词
breast/ovarian cancer susceptibility genes,HBOC,CNV,multigene panel sequencing
AI 理解论文
溯源树
样例
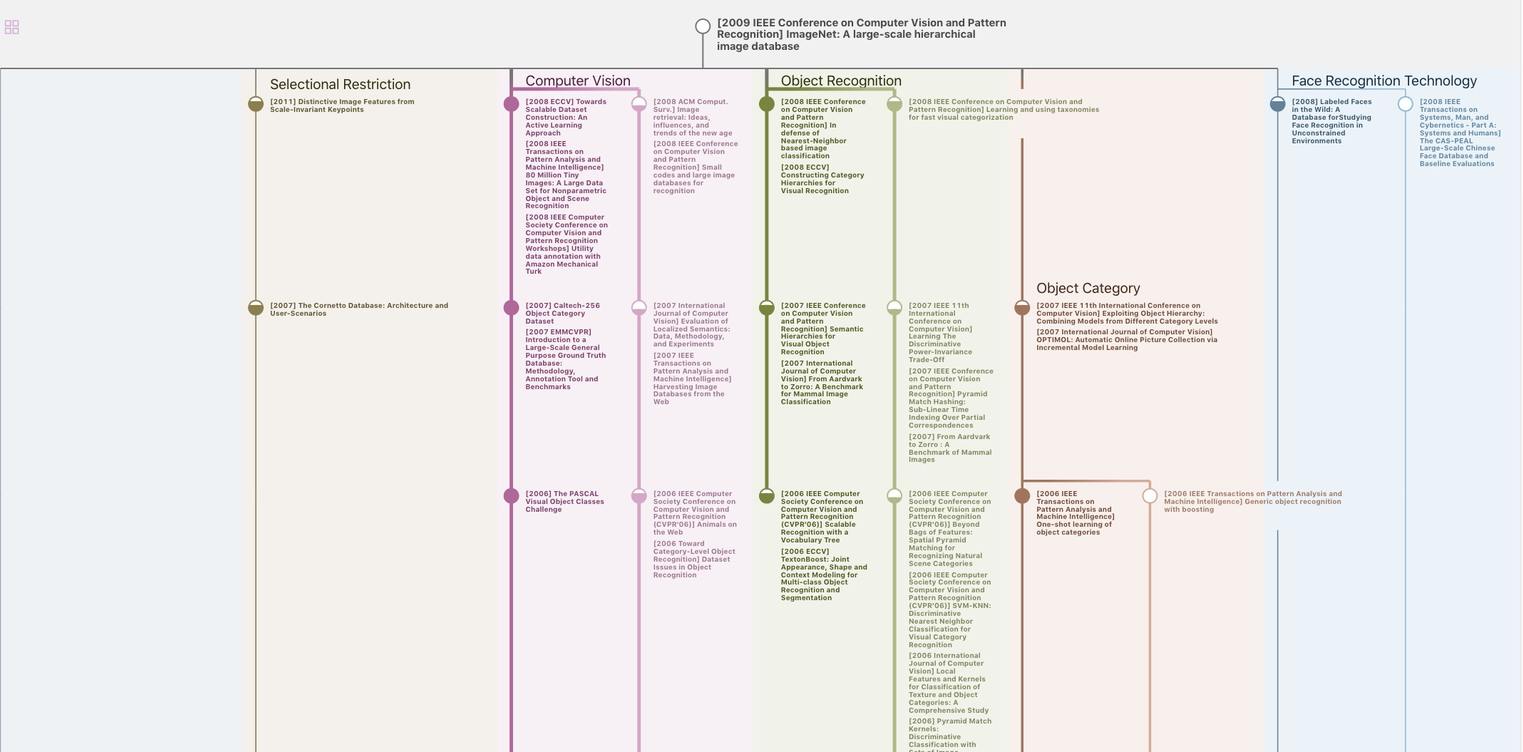
生成溯源树,研究论文发展脉络
Chat Paper
正在生成论文摘要