Combining Slow and Fast Learning for Improved Credit Scoring.
SMC(2020)
摘要
The financial credibility of a person is a relevant factor to determine whether a loan should be approved or not, and it is quantified by a credit score, which is computed using past performance on debt obligations, profiling, and other data available. Credit scoring becomes even a hotter topic in emerging countries, as interest rates and customer behavior swiftly vary, given the economic (in)stability of the country and as fintechs are chasing robust solutions for improved credit scoring solutions. Batch machine learning is often deployed for credit scoring, yet, they are tailored for static scenarios, i.e., they are not prepared to swiftly detect and adapt to changes in customer behavior, thus leading to slow recovery in such scenarios. In this paper, we bring forward an analysis on how batch machine learning can be combined with data stream mining techniques, thus leading to better recognition rates in credit scoring scenarios. We analyze three different real-world datasets from Brazilian financial institutions, whilst keeping their secrecy preserved, and show how batch and stream learning can be combined towards improved credit scoring systems, as well as highlighting relevant gaps that still require attention.
更多查看译文
关键词
Credit scoring,machine learning,data streams
AI 理解论文
溯源树
样例
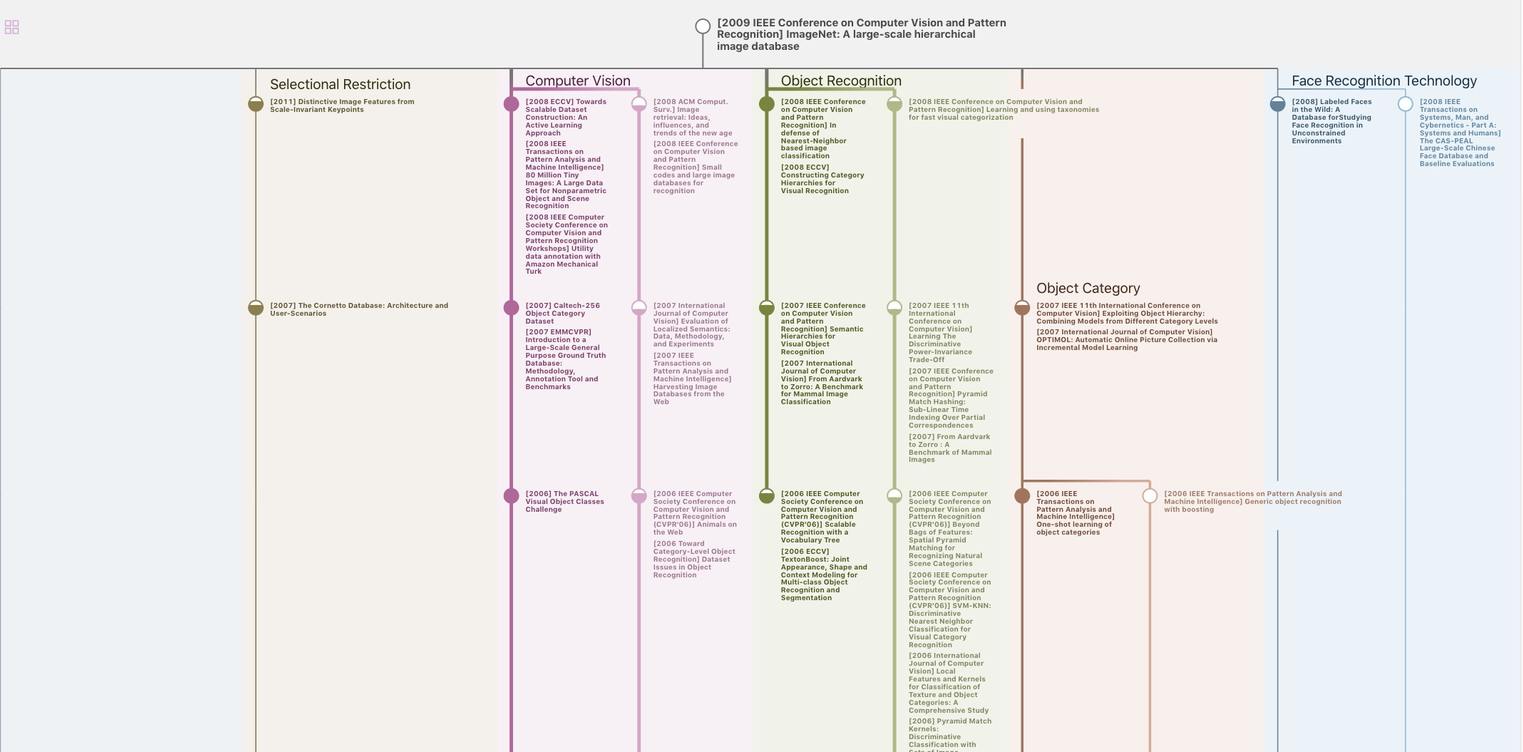
生成溯源树,研究论文发展脉络
Chat Paper
正在生成论文摘要