Trend Training Based RNN for Human Induced Pluripotent Stem Cell Reprogramming Prediction Using Time-Lapse Microscopy Images
2020 IEEE 20th International Conference on Bioinformatics and Bioengineering (BIBE)(2020)
摘要
Recurrent Neural Network (RNN) operates on sequential data using a recurrent hidden state dependent on previous data. Even proved successful in many fields, RNNs suffer from vanishing and exploding gradient problems. Gating approach of LSTM and GRU solves these problems but lose prediction accuracies if short-term changes occur in time-lapse microscopy images taken in cell culture. This study uses trend training (feed forward and pre-training) based RNN to improve the prediction accuracy for human iPS cell reprogramming and formation in future culture. The feed forward RNN uses the prediction of each time-step in the next recursive batch training for the next prediction recursively to give recurrent RNN internal states approaching optimal. Pre-training gives optimal initial RNN parameters selected from RNN models pre-trained by sets of time-lapse images taken from a completed cell culture process. An example shows the predicted accuracies and iPS cell colony shapes by feed forward RNN are apparently better than those without the feed forward training, the accuracies and iPS cell colony shapes furthermore applying the pre-trained models are better than only using the feed forward training. Thirty experimental results show the proposed method achieved 0.752 (SD 0.137) accuracy in future 72 time-steps (1.5 days).
更多查看译文
关键词
time series prediction,recurrent neural network,pre-training,trend based training,human iPS cell reprogramming
AI 理解论文
溯源树
样例
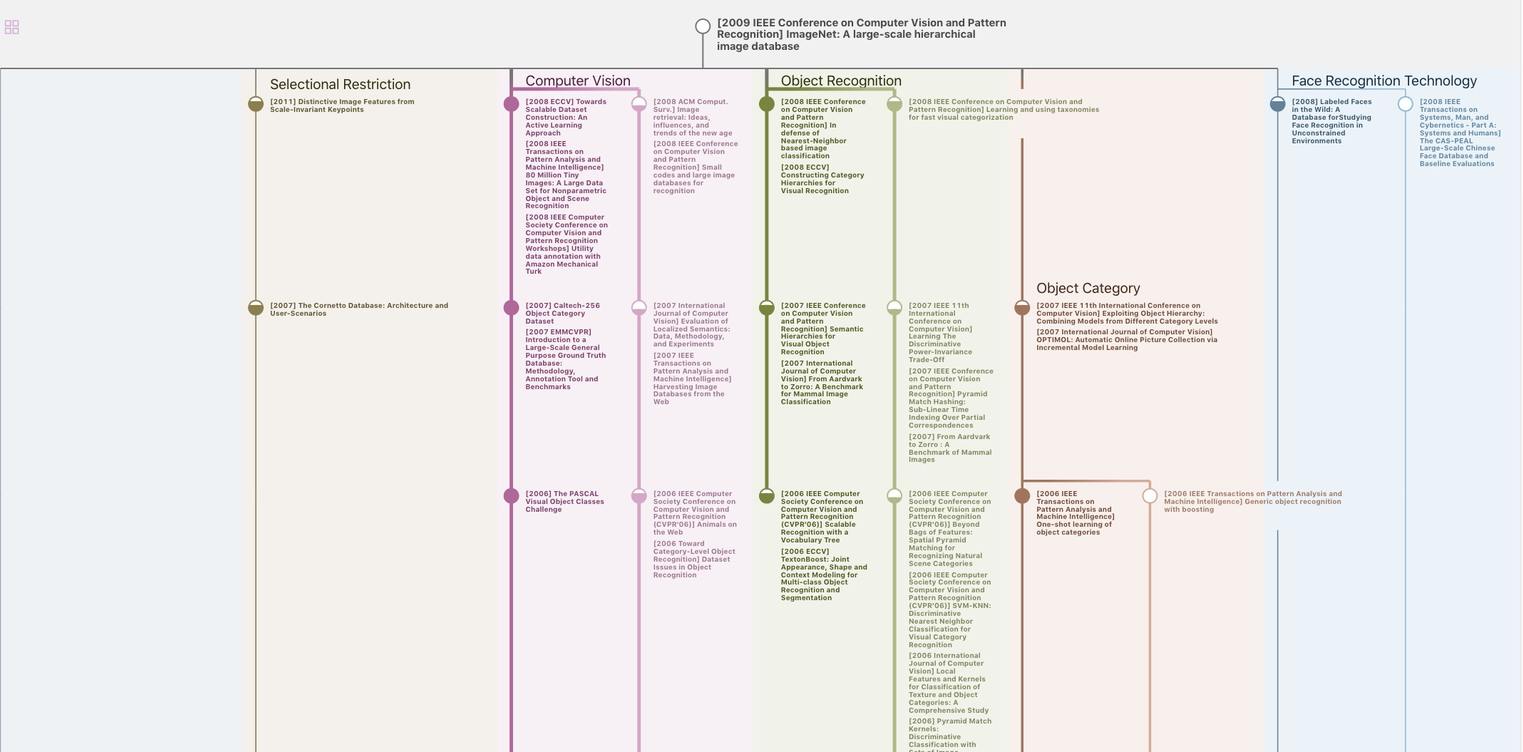
生成溯源树,研究论文发展脉络
Chat Paper
正在生成论文摘要