Algorithms For Overpredictive Signal Analytics In Federated Learning
28TH EUROPEAN SIGNAL PROCESSING CONFERENCE (EUSIPCO 2020)(2021)
摘要
Distributed signal processing will play a major role in performing learning and inference tasks in a client-server model proposed in federated learning. Clients (IoT devices) having many signal samples can aid a data-center (server) in learning the global signal model, by pooling these distributed samples. The clients may have privacy concerns, and the pooling of distributed samples will require accounting of communication cost involved. As a result, a processed approximation of these samples may be desirable. This decentralized learning approach is termed as distributed signal analytics in this work. Overpredictive signal approximations may be desired to perform such distributed signal analytics, which are primarily motivated by applications in network demand (capacity) estimation and planning. In this work, we propose algorithms that calculate an overpredictive signal approximation at the client devices using an efficient convex optimization framework. A tradeoff between the number of bits communicated by clients to the server and the signal approximation error is quantified. An analysis of our approximations is presented on an available residential energy consumption dataset.
更多查看译文
关键词
signal reconstruction, approximation methods
AI 理解论文
溯源树
样例
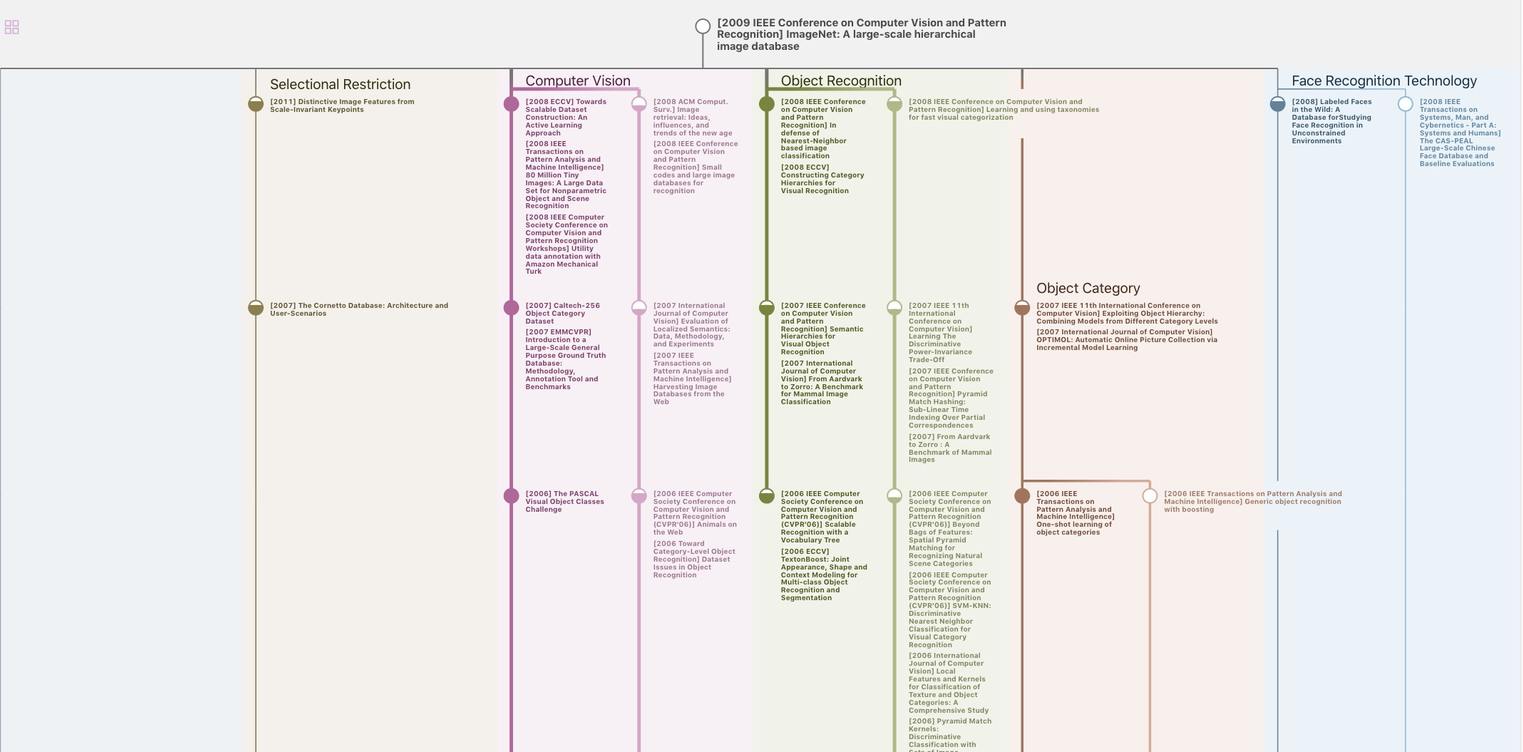
生成溯源树,研究论文发展脉络
Chat Paper
正在生成论文摘要