Convolutional Neural Networks For Underwater Pipeline Segmentation Using Imperfect Datasets
28TH EUROPEAN SIGNAL PROCESSING CONFERENCE (EUSIPCO 2020)(2021)
摘要
In this paper, we investigate a solution to the problem of underwater pipeline segmentation, based on an unbalanced dataset generated by a deterministic algorithm which employs computer vision techniques. We use manually selected masks to train two types of neural networks, U-Net and Deeplabv3+, to solve the same semantic segmentation task. We show that neural networks are able to learn from imperfect datasets, artificially generated by other algorithms. Deep convolutional architectures outperform the algorithm based on computer vision techniques. In order to find the best model, a comparison was made between the two architectures, thereby concluding that Deeplabv3+ achieves better results and features robust operation under adverse environmental conditions.
更多查看译文
关键词
Deep Learning, Convolutional Neural Networks, Semantic Segmentation
AI 理解论文
溯源树
样例
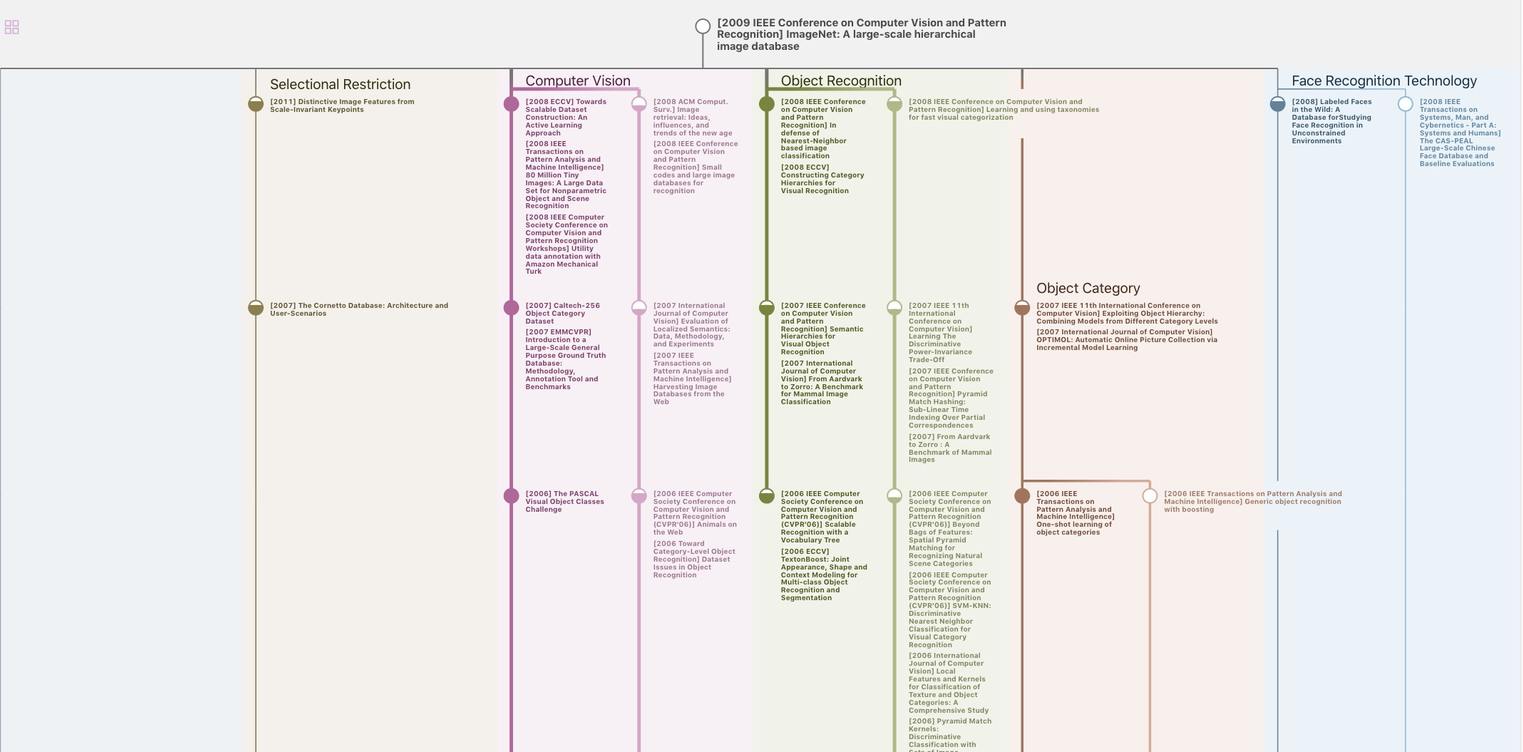
生成溯源树,研究论文发展脉络
Chat Paper
正在生成论文摘要