Online Proximal Gradient For Learning Graphs From Streaming Signals
28TH EUROPEAN SIGNAL PROCESSING CONFERENCE (EUSIPCO 2020)(2021)
摘要
We leverage proximal gradient iterations to develop an online graph learning algorithm from streaming network data. Our goal is to track the (possibly) time-varying network topology, and effect memory and computational savings by processing the data on-the-fly as they are acquired. The setup entails observations modeled as stationary graph signals generated by local diffusion dynamics on the unknown network. Moreover, we may have a priori information on the presence or absence of a few edges as in the link prediction problem. The stationarity assumption implies that the observations' covariance matrix and the so-called graph shift operator (GSO - a matrix encoding the graph topology) commute under mild requirements. This motivates formulating the topology inference task as an inverse problem, whereby one searches for a (e.g., sparse) GSO that is structurally admissible and approximately commutes with the observations' empirical covariance matrix. For streaming data said covariance can be updated recursively, and we show online proximal gradient iterations can be brought to bear to efficiently track the time-varying solution of the inverse problem with quantifiable guarantees. Specifically, we derive conditions under which the GSO recovery cost is strongly convex and use this property to prove that the online algorithm converges to within a neighborhood of the optimal time-varying batch solution. Preliminary numerical tests illustrate the effectiveness of the proposed graph learning approach in adapting to streaming information and tracking changes in the sought dynamic network.
更多查看译文
关键词
Network topology inference, graph signal processing, proximal gradient algorithm, online optimization
AI 理解论文
溯源树
样例
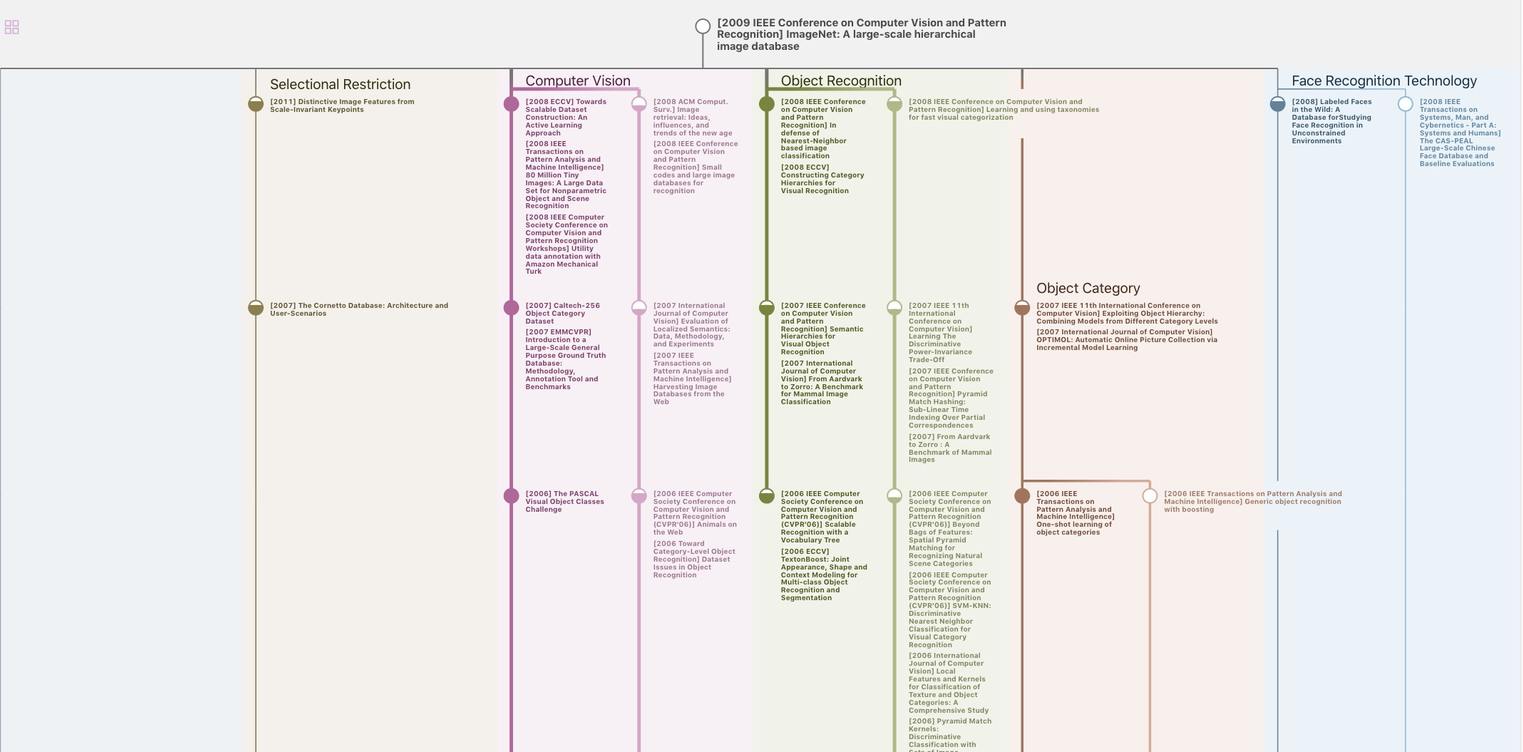
生成溯源树,研究论文发展脉络
Chat Paper
正在生成论文摘要