Reinforcement Learning and Adaptive Optimal Control for Continuous-Time Nonlinear Systems: A Value Iteration Approach
IEEE Transactions on Neural Networks and Learning Systems(2022)
摘要
This article studies the adaptive optimal control problem for continuous-time nonlinear systems described by differential equations. A key strategy is to exploit the value iteration (VI) method proposed initially by Bellman in 1957 as a fundamental tool to solve dynamic programming problems. However, previous VI methods are all exclusively devoted to the Markov decision processes and discrete-time dynamical systems. In this article, we aim to fill up the gap by developing a new continuous-time VI method that will be applied to address the adaptive or nonadaptive optimal control problems for continuous-time systems described by differential equations. Like the traditional VI, the continuous-time VI algorithm retains the nice feature that there is no need to assume the knowledge of an initial admissible control policy. As a direct application of the proposed VI method, a new class of adaptive optimal controllers is obtained for nonlinear systems with totally unknown dynamics. A learning-based control algorithm is proposed to show how to learn robust optimal controllers directly from real-time data. Finally, two examples are given to illustrate the efficacy of the proposed methodology.
更多查看译文
关键词
Adaptive optimal control,nonlinear systems,value iteration (VI)
AI 理解论文
溯源树
样例
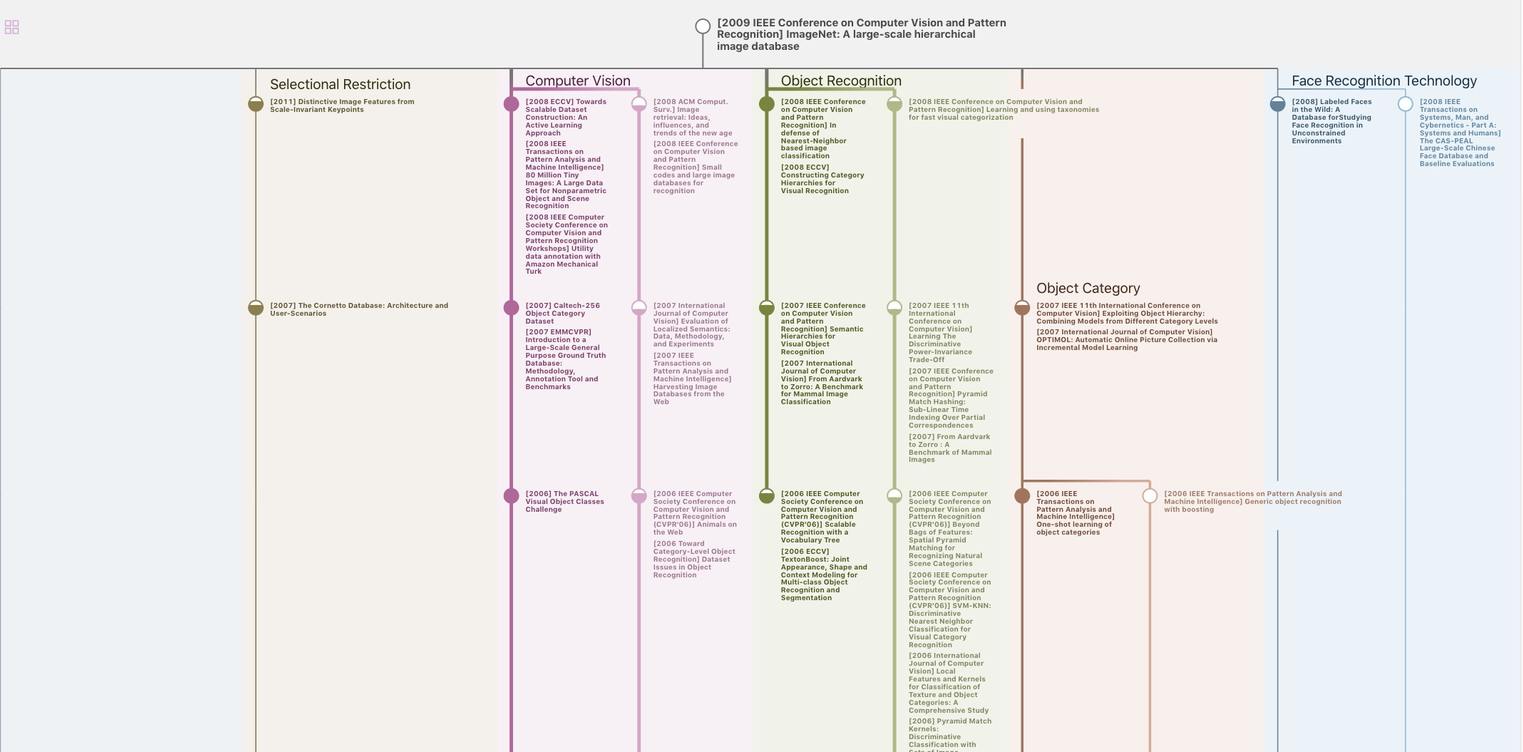
生成溯源树,研究论文发展脉络
Chat Paper
正在生成论文摘要