Differentially Private Federated Learning for Cancer Prediction
arxiv(2021)
摘要
Since 2014, the NIH funded iDASH (integrating Data for Analysis, Anonymization, SHaring) National Center for Biomedical Computing has hosted yearly competitions on the topic of private computing for genomic data. For one track of the 2020 iteration of this competition, participants were challenged to produce an approach to federated learning (FL) training of genomic cancer prediction models using differential privacy (DP), with submissions ranked according to held-out test accuracy for a given set of DP budgets. More precisely, in this track, we are tasked with training a supervised model for the prediction of breast cancer occurrence from genomic data split between two virtual centers while ensuring data privacy with respect to model transfer via DP. In this article, we present our 3rd place submission to this competition. During the competition, we encountered two main challenges discussed in this article: i) ensuring correctness of the privacy budget evaluation and ii) achieving an acceptable trade-off between prediction performance and privacy budget.
更多查看译文
关键词
private federated learning,prediction
AI 理解论文
溯源树
样例
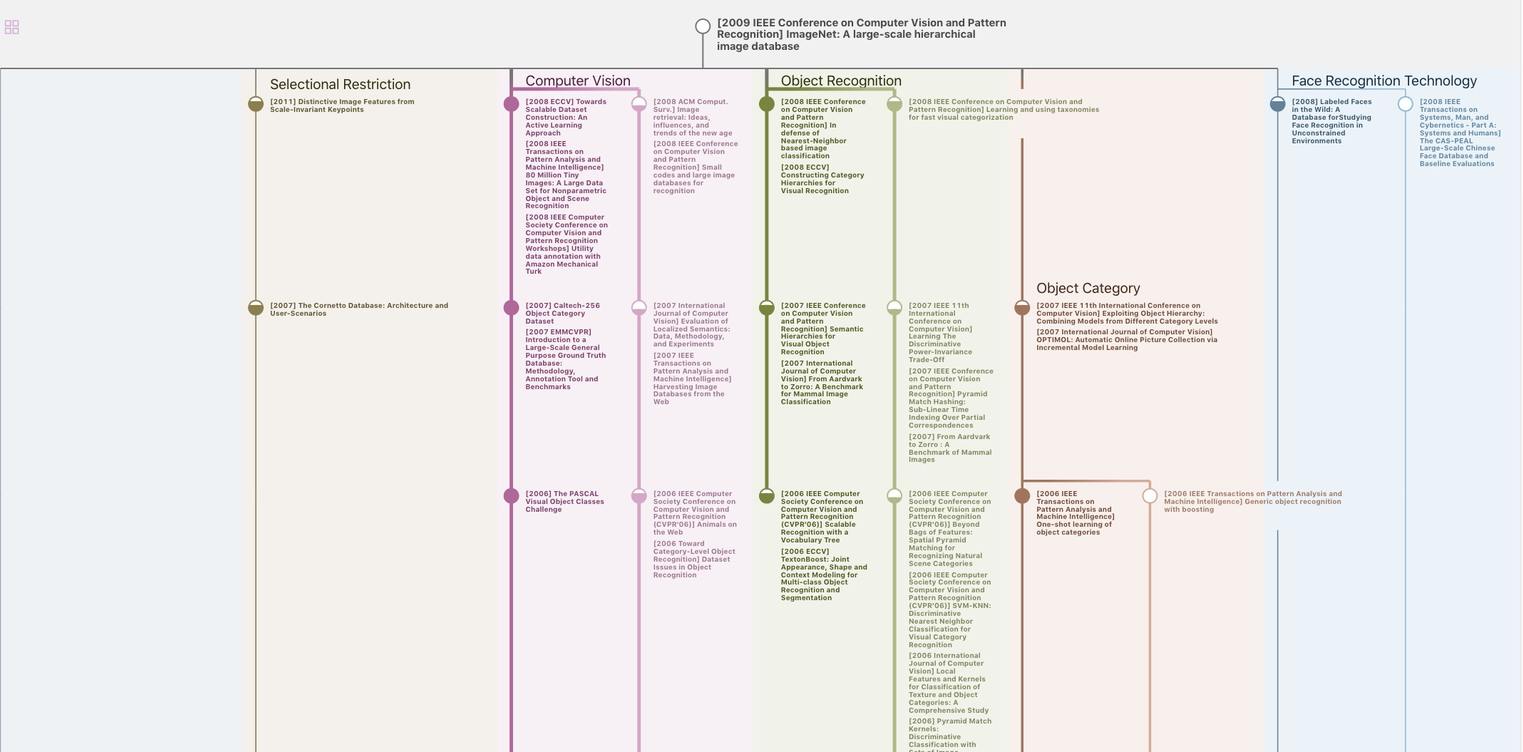
生成溯源树,研究论文发展脉络
Chat Paper
正在生成论文摘要