Adaptive Learning in Two-Player Stackelberg Games with Application to Network Security
arxiv(2021)
摘要
We study a two-player Stackelberg game with incomplete information such that the follower's strategy belongs to a known family of parameterized functions with an unknown parameter vector. We design an adaptive learning approach to simultaneously estimate the unknown parameter and minimize the leader's cost, based on adaptive control techniques and hysteresis switching. Our approach guarantees that the leader's cost predicted using the parameter estimate becomes indistinguishable from its actual cost in finite time, up to a preselected, arbitrarily small error threshold. Also, the first-order necessary condition for optimality holds asymptotically for the predicted cost. Additionally, if a persistent excitation condition holds, then the parameter estimation error becomes bounded by a preselected, arbitrarily small threshold in finite time as well. For the case where there is a mismatch between the follower's strategy and the parameterized function that is known to the leader, our approach is able to guarantee the same convergence results for error thresholds larger than the size of the mismatch. The algorithms and the convergence results are illustrated via a simulation example in the domain of network security.
更多查看译文
关键词
network security,learning,two-player
AI 理解论文
溯源树
样例
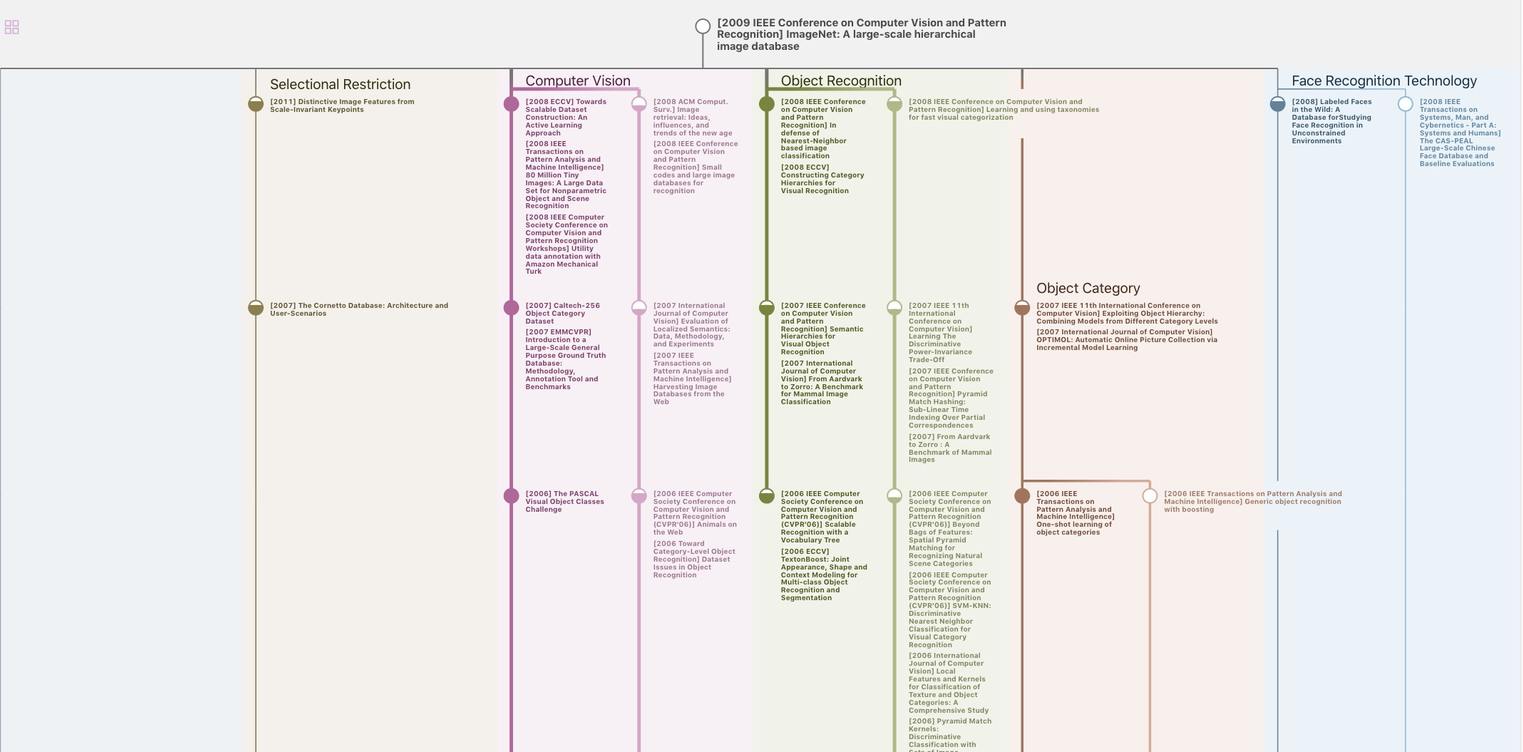
生成溯源树,研究论文发展脉络
Chat Paper
正在生成论文摘要