Invariant Neural Network Ansatz For Weakly Symmetric Open Quantum Lattices
PHYSICAL REVIEW A(2021)
摘要
We consider an open quantum system of N interacting spin-1/2 particles on a d-dimensional lattice whose time evolution is (weakly) symmetric under the action of a finite group G that is a subgroup of all the possible permutations of the lattice sites. We define a neural network representation for the system density operator that explicitly accounts for the system symmetries, and we show that its properties are preserved when using variational techniques such those recently used in the optimization of restricted-Boltzmann machines (RBMs). As a proof of principle, we demonstrate the validity of our approach by determining the steady state structure of the one-dimensional dissipative XYZ model in the presence of a uniform magnetic field and uniform dissipation. We first show a direct comparison between the results obtained by optimizing a RBM representation for the density operator and those obtained by means of the invariant neural network defined in the present paper, and then we show results concerning the convergence to the unique steady state configuration when using our approach only. Numerical results support the conjecture that the invariant Ansatz defined in this paper is more expressive that a standard RBM and can be optimized with lesser numerical efforts.
更多查看译文
AI 理解论文
溯源树
样例
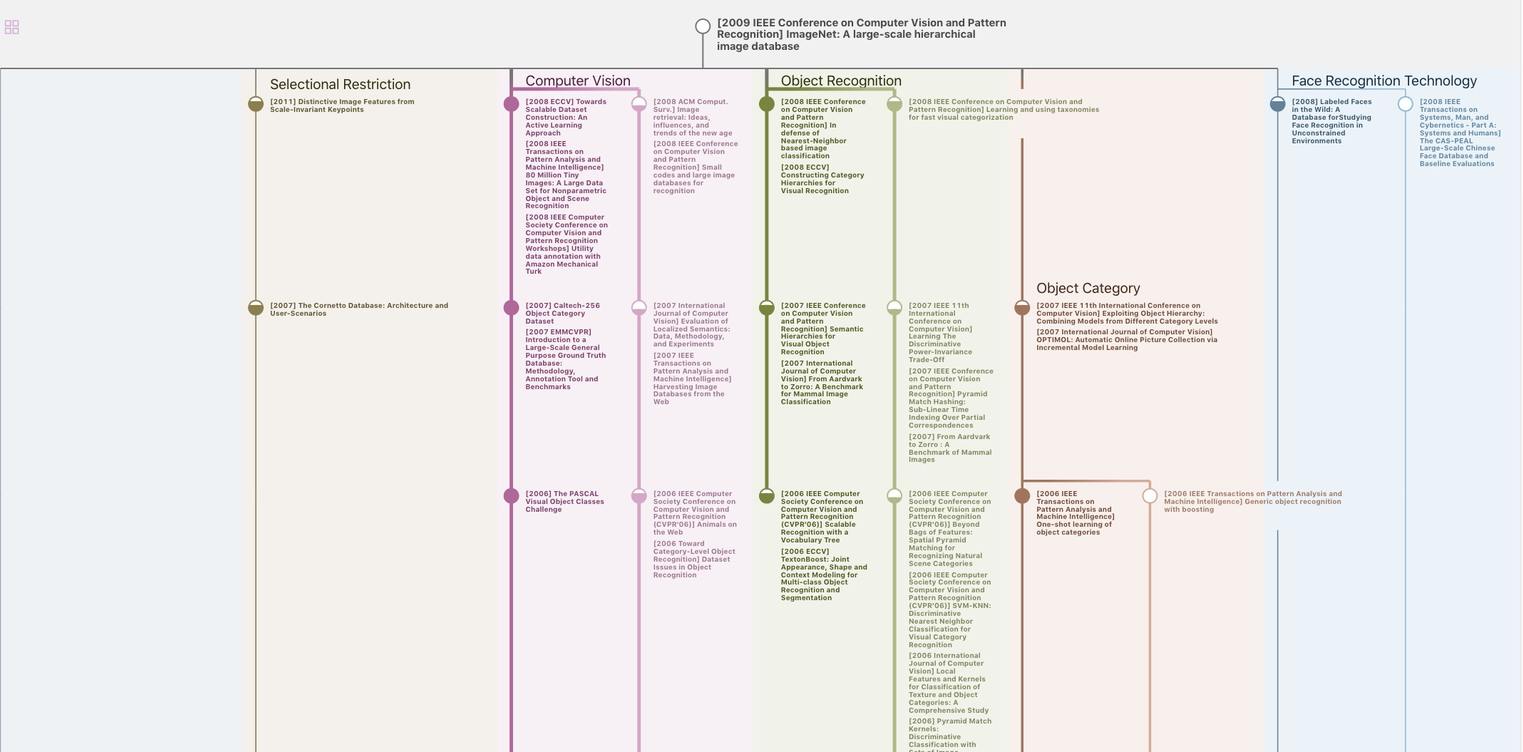
生成溯源树,研究论文发展脉络
Chat Paper
正在生成论文摘要