Solving phase retrieval with random initial guess is nearly as good as by spectral initialization
APPLIED AND COMPUTATIONAL HARMONIC ANALYSIS(2022)
摘要
The problem of recovering a signal x is an element of R-n from a set of magnitude measurements yi = |(a(i), x)|, i = 1, . .. , m is referred as phase retrieval, which has many applications in fields of physical sciences , engineering. In this paper we show that the smoothed amplitude flow based model for phase retrieval has benign geometric structure under the optimal sampling complexity. In particular, we show that when the measurements ai is an element of R-n are Gaussian random vectors and the number of measurements m > Cn, our smoothed amplitude flow based model has no spurious local minimizers with high probability, i.e., the target solution x is the unique global minimizer (up to a global phase) and the loss function has a negative directional curvature around each saddle point. Due to this benign geometric landscape, the phase retrieval problem can be solved by the gradient descent algorithms without spectral initialization. Numerical experiments show that the gradient descent algorithm with random initialization performs well even comparing with state-of-the-art algorithms with spectral initialization in empirical success rate and convergence speed. (C) 2022 Elsevier Inc. All rights reserved.
更多查看译文
关键词
Phase retrieval,Geometric landscape,Nonconvex,Phaseless measurements
AI 理解论文
溯源树
样例
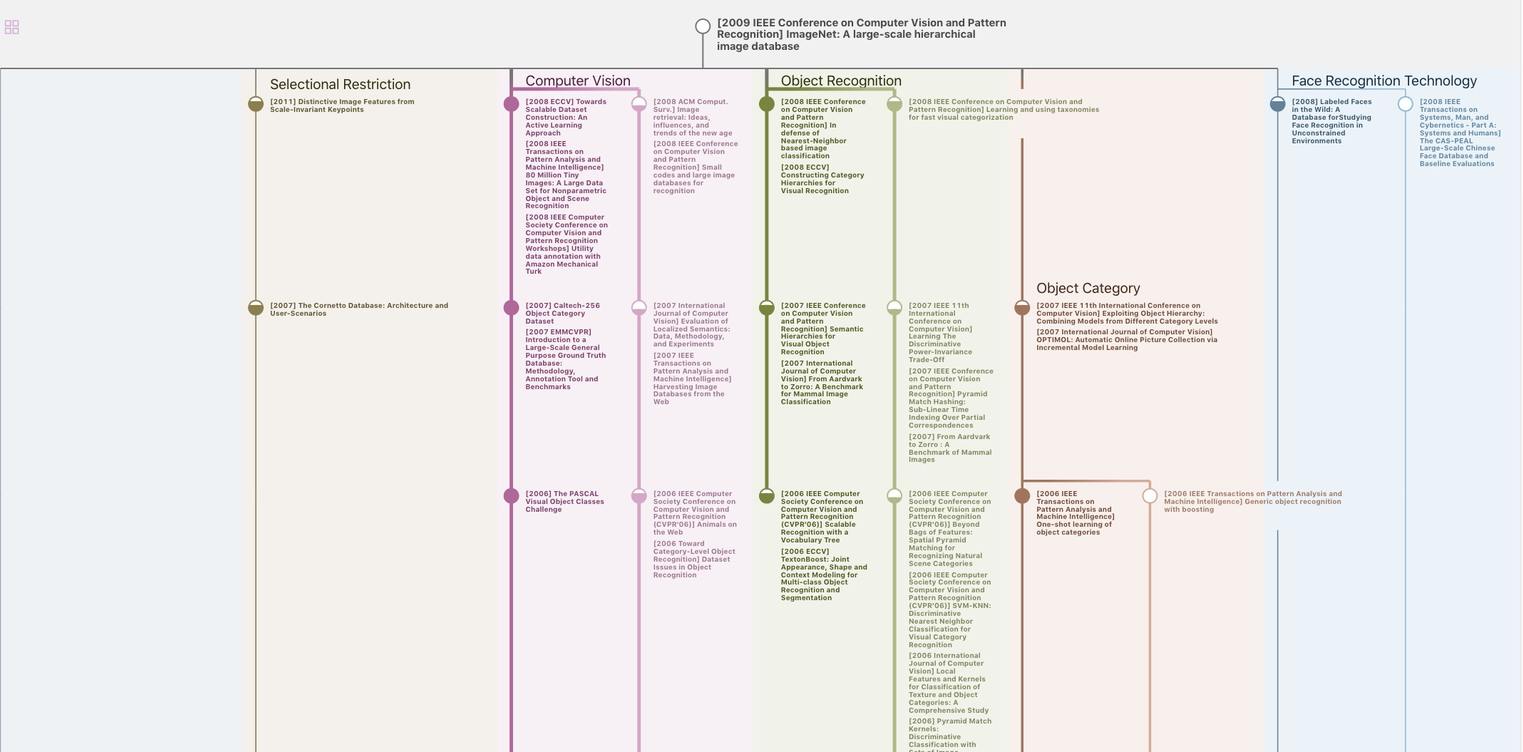
生成溯源树,研究论文发展脉络
Chat Paper
正在生成论文摘要