Statistical methods for cis-Mendelian randomization with two-sample summary-level data
Genetic Epidemiology(2023)
摘要
Mendelian randomization (MR) is the use of genetic variants to assess the existence of a causal relationship between a risk factor and an outcome of interest. Here, we focus on two-sample summary-data MR analyses with many correlated variants from a single gene region, particularly on cis-MR studies which use protein expression as a risk factor. Such studies must rely on a small, curated set of variants from the studied region; using all variants in the region requires inverting an ill-conditioned genetic correlation matrix and results in numerically unstable causal effect estimates. We review methods for variable selection and estimation in cis-MR with summary-level data, ranging from stepwise pruning and conditional analysis to principal components analysis, factor analysis, and Bayesian variable selection. In a simulation study, we show that the various methods have comparable performance in analyses with large sample sizes and strong genetic instruments. However, when weak instrument bias is suspected, factor analysis and Bayesian variable selection produce more reliable inferences than simple pruning approaches, which are often used in practice. We conclude by examining two case studies, assessing the effects of low-density lipoprotein-cholesterol and serum testosterone on coronary heart disease risk using variants in the HMGCR and SHBG gene regions, respectively.
更多查看译文
关键词
Bayesian variable selection,correlated instruments,factor analysis,Mendelian randomization,principal components analysis,pruning
AI 理解论文
溯源树
样例
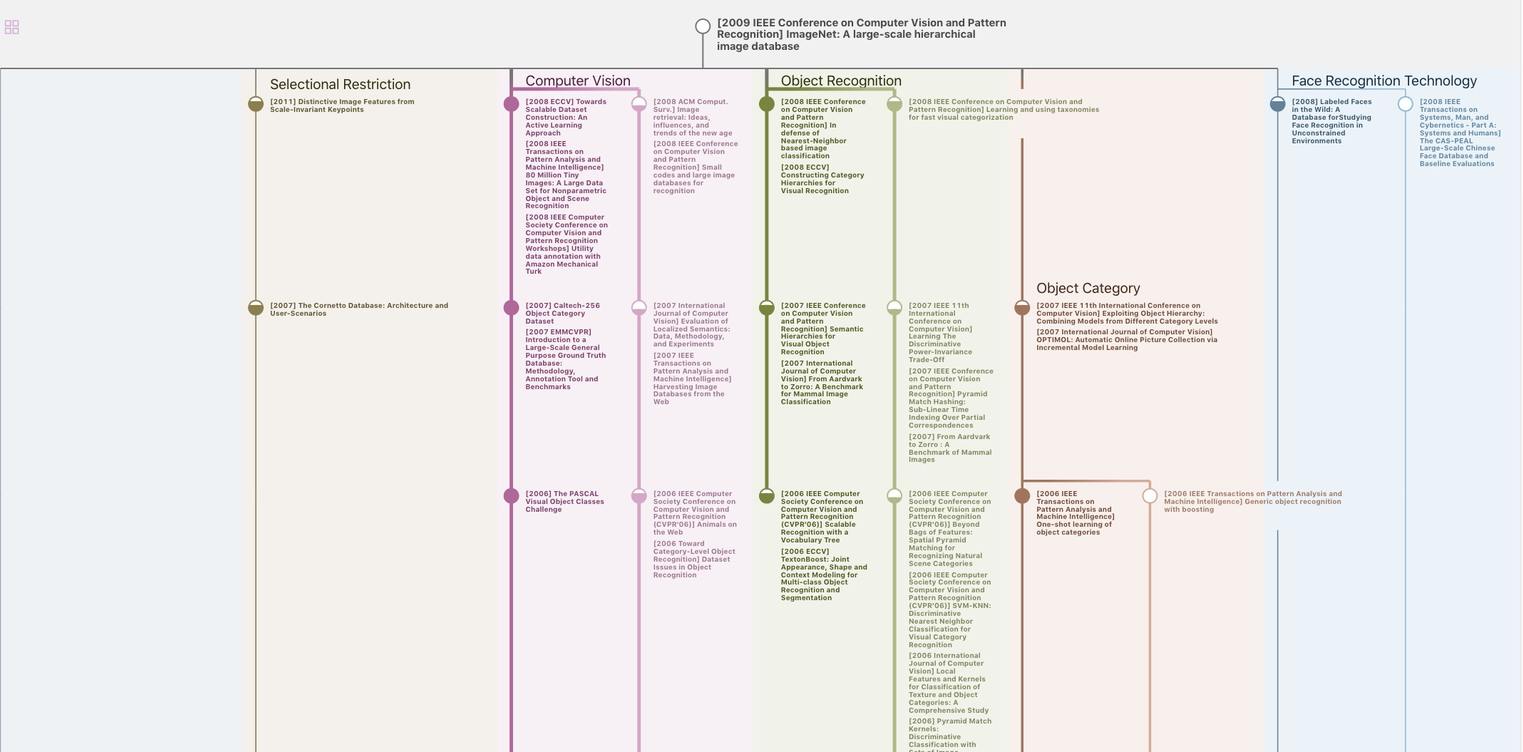
生成溯源树,研究论文发展脉络
Chat Paper
正在生成论文摘要