A Co-Attention Model with Sequential Behaviors and Side Information for Session- based Recommendation
2020 IEEE International Conference on Web Services (ICWS)(2020)
摘要
Session-based recommendation aims to recommend the next item a user might be interested in given limited session information, e.g., only clicks are available. Existing researches usually use the RNN-based method or combine user long-term and current session interests to learn certain user preferences. However, due to the uncertainty of user behaviors and the limited behavior information, these methods may lose information of the relevant behavioral features and introduce some noise of unrelated behaviors. For online platforms, such as knowledge base platforms, these items are not isolated but connected with each other. We can use the correlation between items to capture the potential long-distance interests of the user. Therefore, in this paper, we propose a co-attention model with sequential behaviors and side information to obtain a complete representation of user's preferences. For obtaining relevant side information outside the session, we aggregate the corresponding entities and relations of each item to get the representation of the neighborhood information. Finally, extensive experiments are carried out on two real datasets, and the experimental results demonstrate the validity of our model. In particular, the proposed model performs well in cold start scenarios and is well interpreted for the recommended results.
更多查看译文
关键词
Session-based Recommendation,Side Information,Attention Mechanism,Gated Recurrent Unit,Graph Convolutional Network
AI 理解论文
溯源树
样例
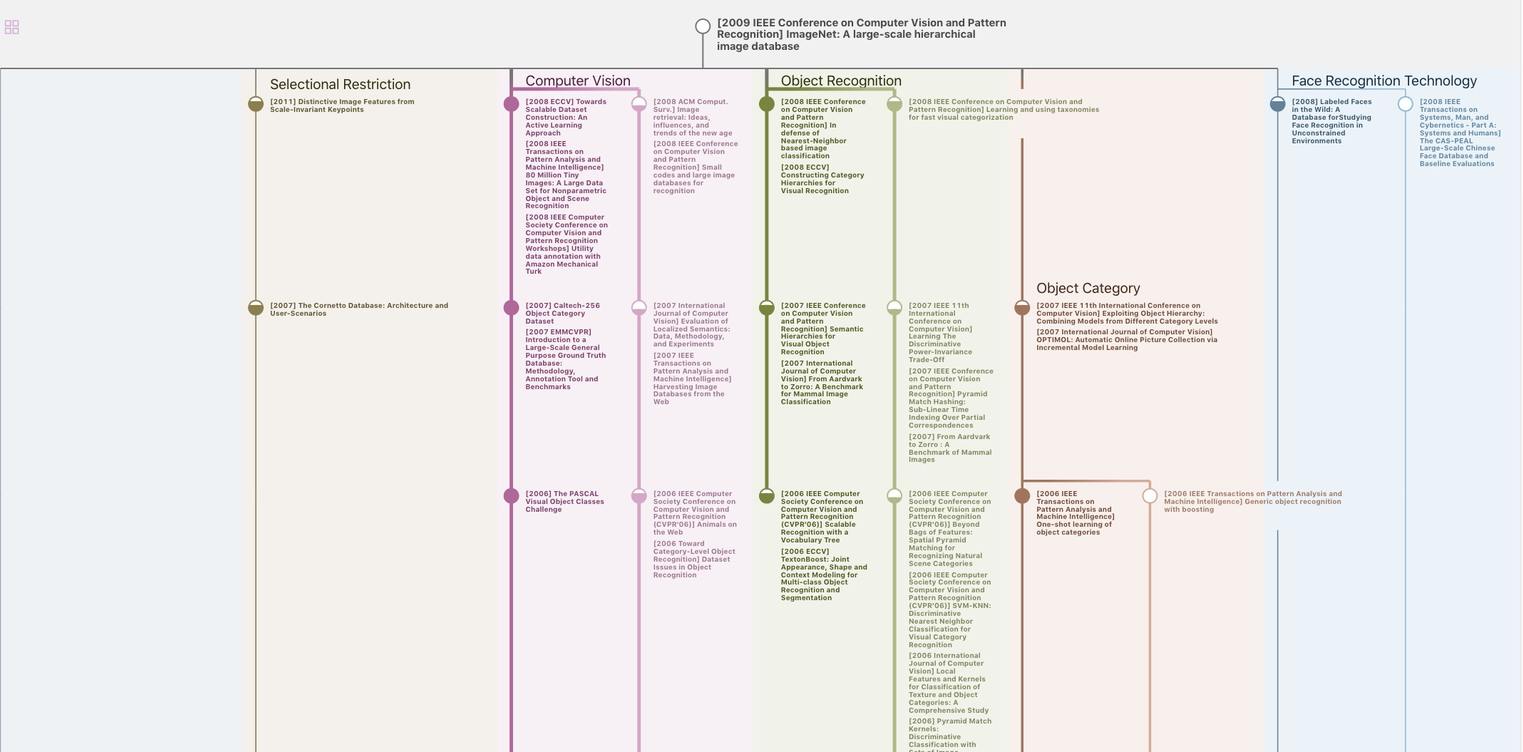
生成溯源树,研究论文发展脉络
Chat Paper
正在生成论文摘要