Deep Inverse Reinforcement Learning for Behavior Prediction in Autonomous Driving: Accurate Forecasts of Vehicle Motion
IEEE Signal Processing Magazine(2021)
摘要
Accurate behavior anticipation is essential for autonomous vehicles when navigating in close proximity to other vehicles, pedestrians, and cyclists. Thanks to the recent advances in deep learning and inverse reinforcement learning (IRL), we observe a tremendous opportunity to address this need, which was once believed impossible given the complex nature of human decision making. In this article, we summarize the importance of accurate behavior modeling in autonomous driving and analyze the key approaches and major progress that researchers have made, focusing on the potential of deep IRL (D-IRL) to overcome the limitations of previous techniques. We provide quantitative and qualitative evaluations substantiating these observations. Although the field of D-IRL has seen recent successes, its application to model behavior in autonomous driving is largely unexplored. As such, we conclude this article by summarizing the exciting pathways for future breakthroughs.
更多查看译文
关键词
deep inverse reinforcement learning,behavior prediction,autonomous driving,vehicle motion,autonomous vehicles,deep learning,human decision making,deep IRL,D-IRL,behavior anticipation,navigation,behavior modeling,quantitative evaluations,qualitative evaluations
AI 理解论文
溯源树
样例
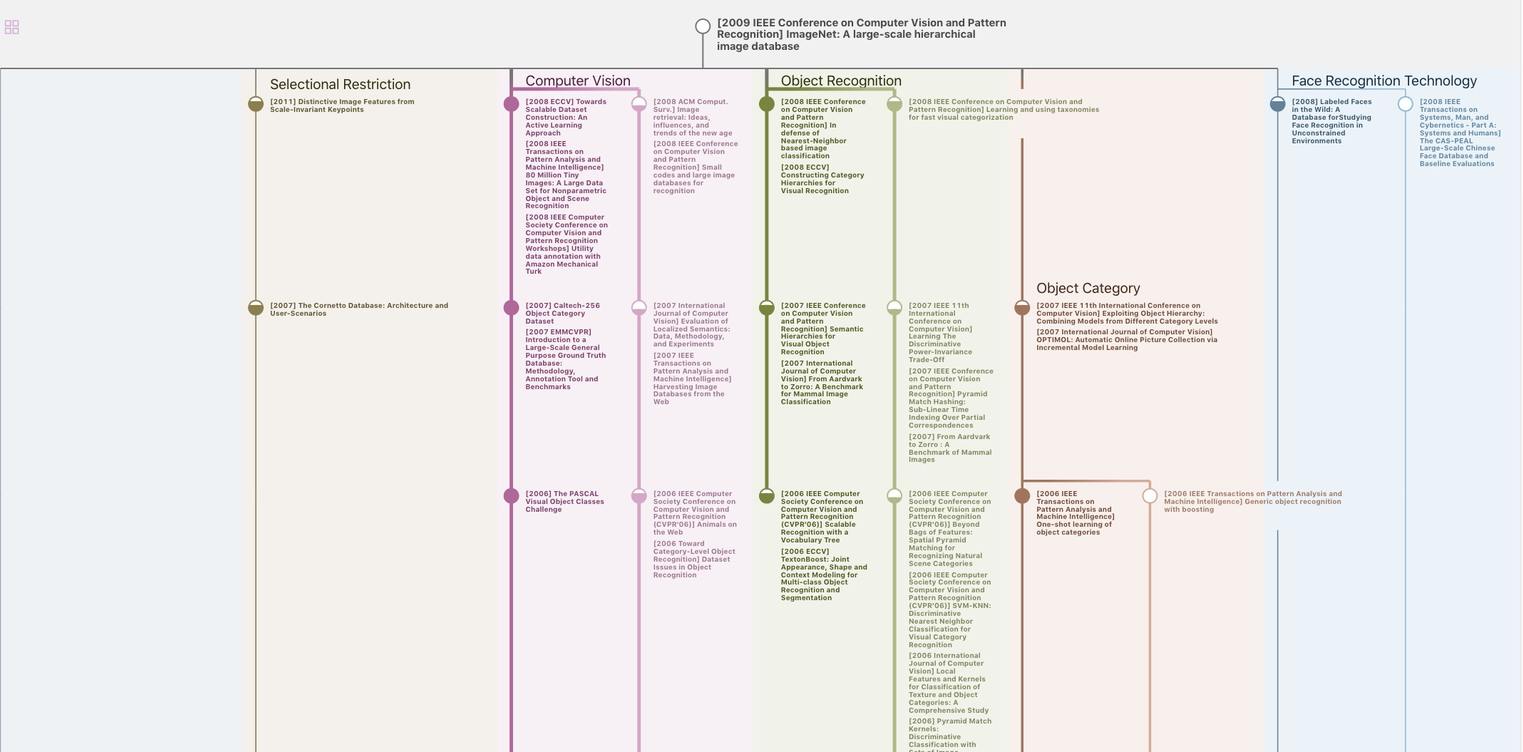
生成溯源树,研究论文发展脉络
Chat Paper
正在生成论文摘要