Infrared And Visible Image Fusion Using A Shallow Cnn And Structural Similarity Constraint
IET IMAGE PROCESSING(2020)
摘要
In recent years, image fusion methods based on deep networks have been proposed to combine infrared and visible images for achieving better fusion image. However, issues such as limited training data, scarce reference images and misalignment of multi-source images, still limit the fusion performance. To address these problems, we propose an end-to-end shallow convolutional neural network with structural constraints, which has only one convolutional layer to fuse infrared and visible images. Different from other methods, our proposed model requires less training data and reference images and is more robust to the misalignment of a couple of images. More specifically, the infrared image and the visible image are first provided as inputs to a convolutional layer to extract the information that should be fused; then, all feature maps are concatenated together and fed into a convolutional layer with one channel to obtain the fused image; finally, a structural similarity loss between the fused image and the input infrared and visible images is computed to update the network parameters and eliminate the effects of pixel misalignment. Extensive experiments show the effectiveness of our proposed method on fusion of infrared and visible images with the performance that outperforms the state-of-the-art methods.
更多查看译文
关键词
learning (artificial intelligence), infrared imaging, image fusion, convolutional neural nets, convolutional layer, fused single image, visible image, structural similarity constraint, infrared image fusion method, training data, scarce reference images, multisource images, deep models, end-to-end shallow convolutional neural network, visible image fusion, shallow CNN, rectified linear unit function, structural similarity loss, pixel misalignment
AI 理解论文
溯源树
样例
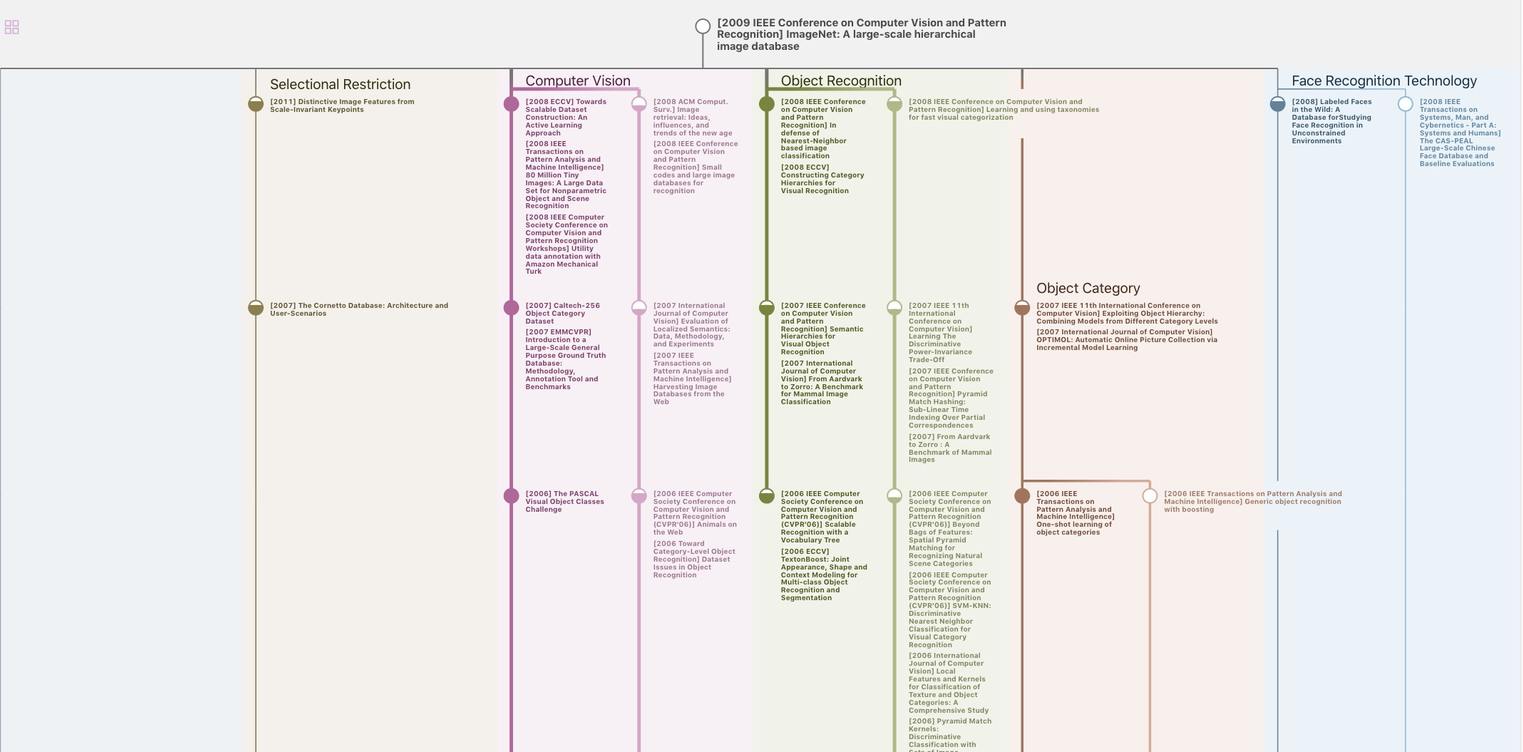
生成溯源树,研究论文发展脉络
Chat Paper
正在生成论文摘要