Clustered-Sparse Bayesian Learning For Channel Estimation In Underwater Acoustic Ofdm Systems
2020 12TH INTERNATIONAL CONFERENCE ON WIRELESS COMMUNICATIONS AND SIGNAL PROCESSING (WCSP)(2020)
摘要
Underwater acoustic channels typically exhibit clustered-sparse structure, and sparse channel recovery based on the clustered-sparse structure can achieve smaller structural error. We propose a novel clustered-sparse Bayesian model for sparse channel estimation in underwater acoustic (UWA) orthogonal frequency-division multiplexing (OFDM) communication systems. In this model, the sparsity and temporal correlation of channel impulse responses (CIRs) are controlled by a set of hyperparameters. For continuously transmitted OFDM blocks in one frame, the auto-regressive (AR) process is used to characterize temporal correlation of time-varying UWA channels, and different clusters are considered to have different AR model coefficients, as they are usually generated according to different transmission routes. A joint cluster partition and sparse Bayesian learning algorithm (JCP-SBL) is proposed to learn the clustered-sparse structure of UWA channels when the cluster partition is unknown. Results of numerical simulation and lake trial demonstrate the effectiveness of the JCP-SBL channel estimator in time-varying UWA channel, which achieve better channel estimation performance and lower bit error rate (BER) compared with the orthogonal matching pursuit (OMP), simultaneous OMP (SOMP) and temporal multiple sparse Bayesian learning (TMSBL).
更多查看译文
关键词
sparse channel estimation, clustered-sparse Bayesian learning, underwater acoustic channel, orthogonal frequency division multiplexing
AI 理解论文
溯源树
样例
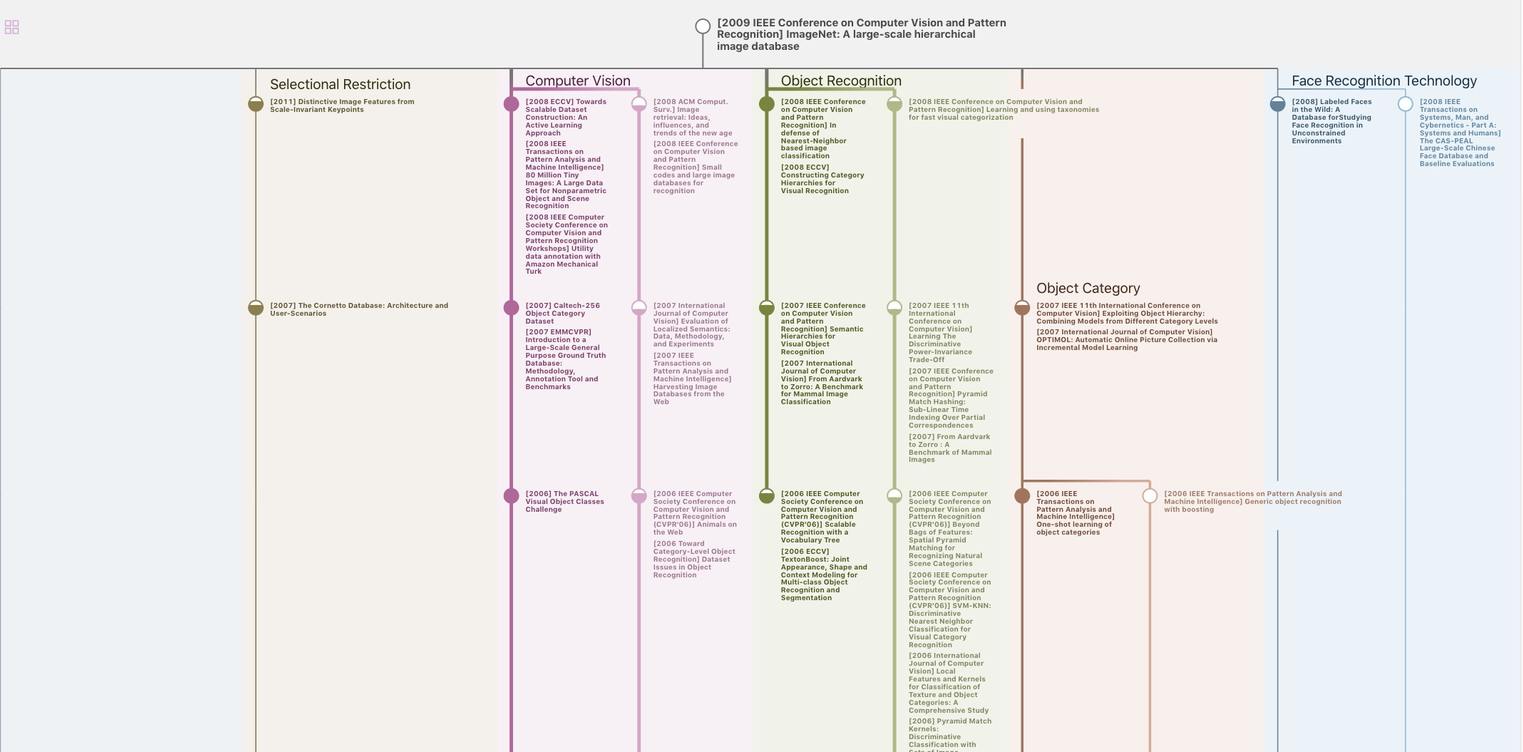
生成溯源树,研究论文发展脉络
Chat Paper
正在生成论文摘要