Deep Reinforcement Learning-Based Edge Caching in Single-Cell Wireless Networks
2020 International Conference on Wireless Communications and Signal Processing (WCSP)(2020)
摘要
In this paper, edge caching is considered in a single-cell network in order to alleviate the traffic congestion of wireless networks as well as improve the users' quality of experience. Instead of assuming a content popularity distribution known a priori as in most existing works, we consider a practical scenario where the content popularity varies over time and it is intractable to know the content popularity distribution in advance. In order to make efficient content caching and updating decisions according to the dynamic content popularity, an optimization problem is first formulated to maximize the long-term cache-hit-ratio at the base station. To solve this problem, we then propose a deep reinforcement learning-based algorithm that can adjust the caching and updating strategies based on the historical observations. In order to simulate the dynamics of content popularity, we use a real-world data set from MovieLens to evaluate the performance of the proposed algorithm. By comparisons with existing caching policies, numerical results demonstrate that the long-term cache-hit-ratio can be significantly improved by the proposed algorithm.
更多查看译文
关键词
Edge caching,dynamic content popularity,deep reinforcement learning,cache-hit-ratio
AI 理解论文
溯源树
样例
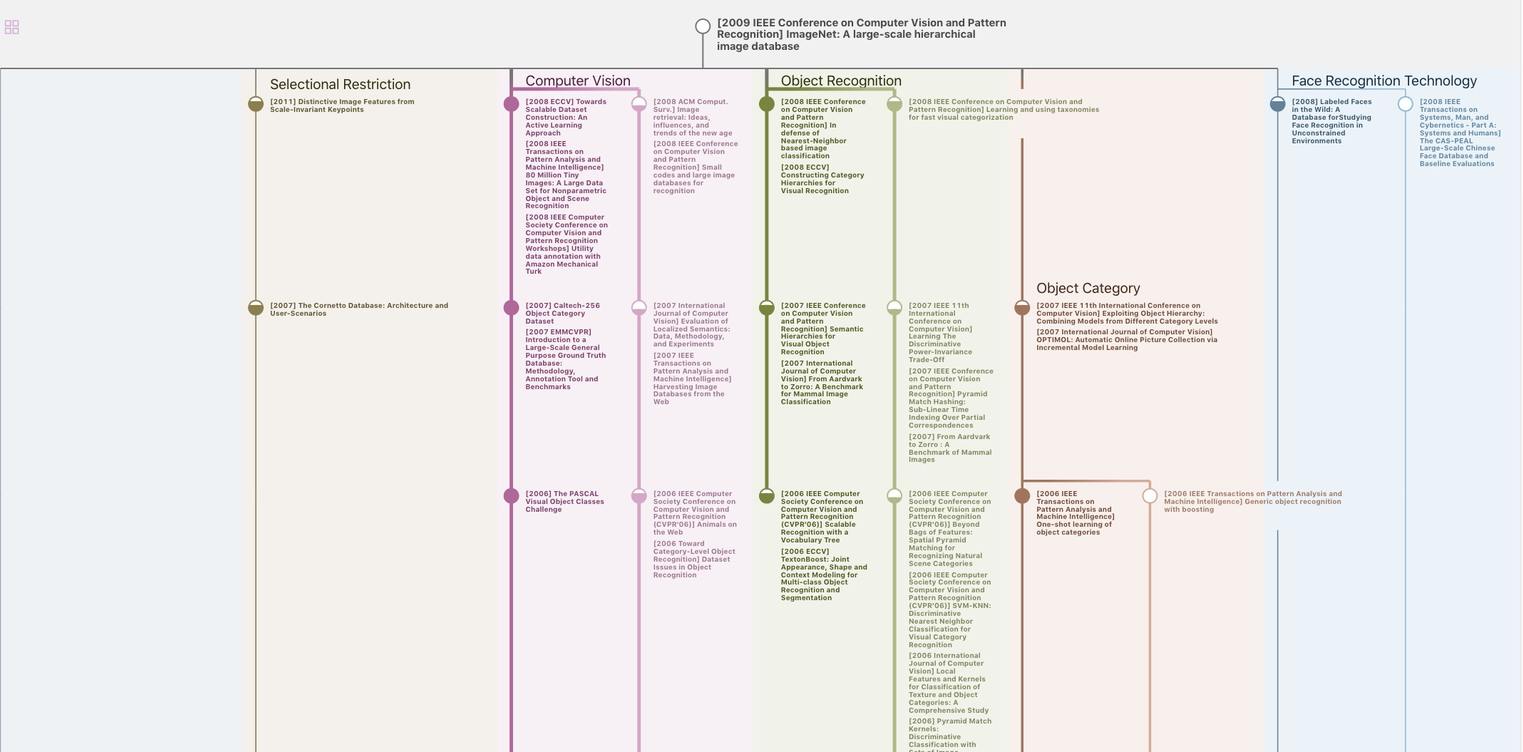
生成溯源树,研究论文发展脉络
Chat Paper
正在生成论文摘要