Beyond Floating-Point Ops: CNN Performance Prediction with Critical Datapath Length
2020 IEEE High Performance Extreme Computing Conference (HPEC)(2020)
摘要
We propose Critical Datapath Length (CDL), a powerful, interpretable metric of neural-network models that enables accurate execution time prediction on parallel device architectures. CDL addresses the fact that the total number of floating-point operations (FLOPs) in a model is an inconsistent predictor of real execution time due to the highly parallel nature of tensor operations and hardware accelerators. Our results show that, on GPUs, CDL correlates to execution time significantly better than FLOPs, making it a useful performance predictor.
更多查看译文
关键词
Benchmarks,Parallel Architecture,FLOPs,Deep Learning,Convolutional Neural Networks,Performance Optimization
AI 理解论文
溯源树
样例
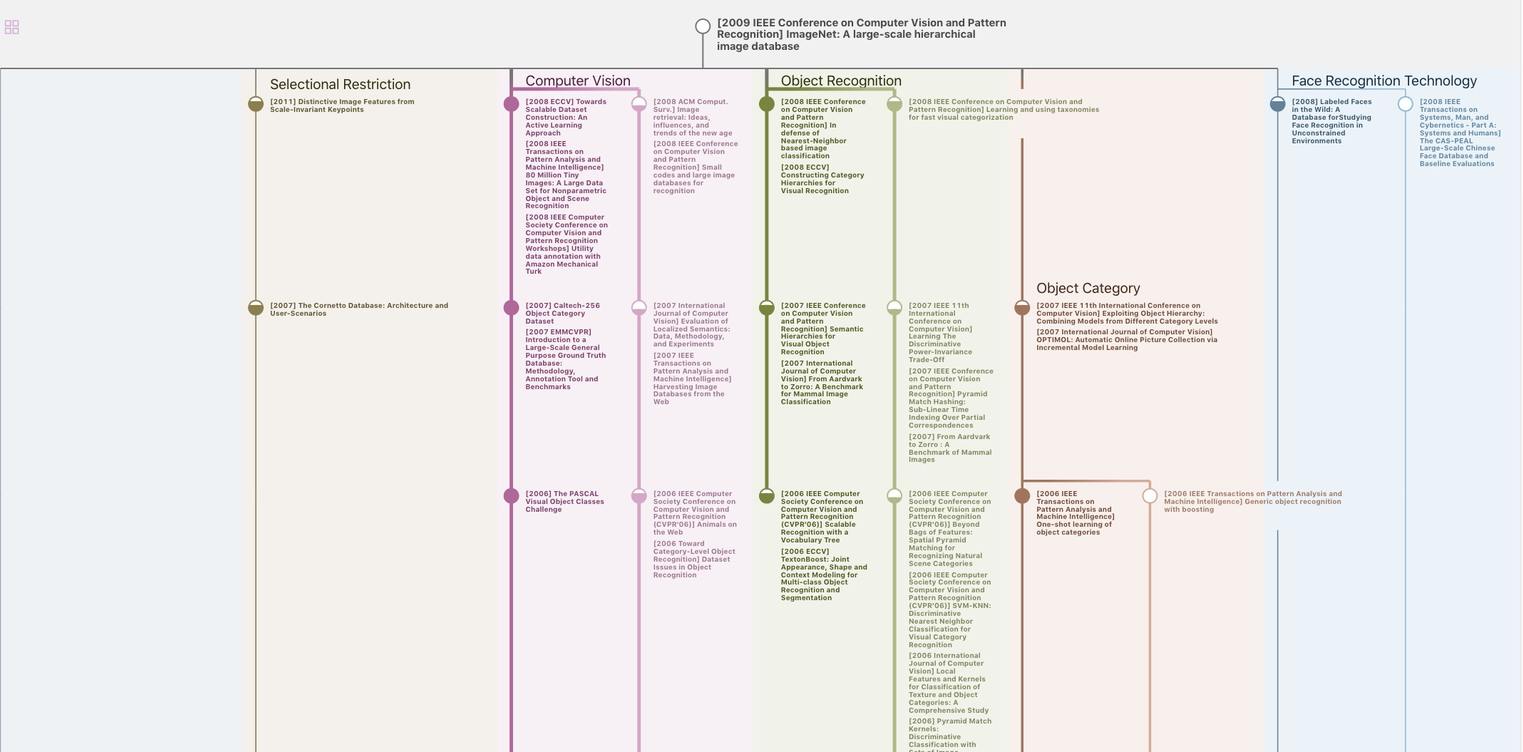
生成溯源树,研究论文发展脉络
Chat Paper
正在生成论文摘要