Multiscale Data Analysis Using Binning, Tensor Decompositions, And Backtracking
2020 IEEE HIGH PERFORMANCE EXTREME COMPUTING CONFERENCE (HPEC)(2020)
摘要
Large data sets can contain patterns at multiple scales (spatial, temporal, etc.). In practice, it is useful for data exploration techniques to detect patterns at each relevant scale. In this paper, we develop an approach to detect activities at multiple scales using tensor decomposition, an unsupervised high-dimensional data analysis technique that finds correlations between different features in the data. This method typically requires that the feature values are discretized during the construction of the tensor in a process called "binning." We develop a method of constructing and decomposing tensors with different binning schemes of various features in order to uncover patterns across a set of user-defined scales. While binning is necessary to obtain interpretable results from tensor decompositions, it also decreases the specificity of the data. Thus, we develop backtracking methods that enable the recovery of original source data corresponding to patterns found in the decomposition. These technique are discussed in the context of spatiotemporal and network traffic data, and in particular on Automatic Identification System (AIS) data.
更多查看译文
关键词
Tensor Decomposition, Geospatial, Spatiotemporal, Multiscale
AI 理解论文
溯源树
样例
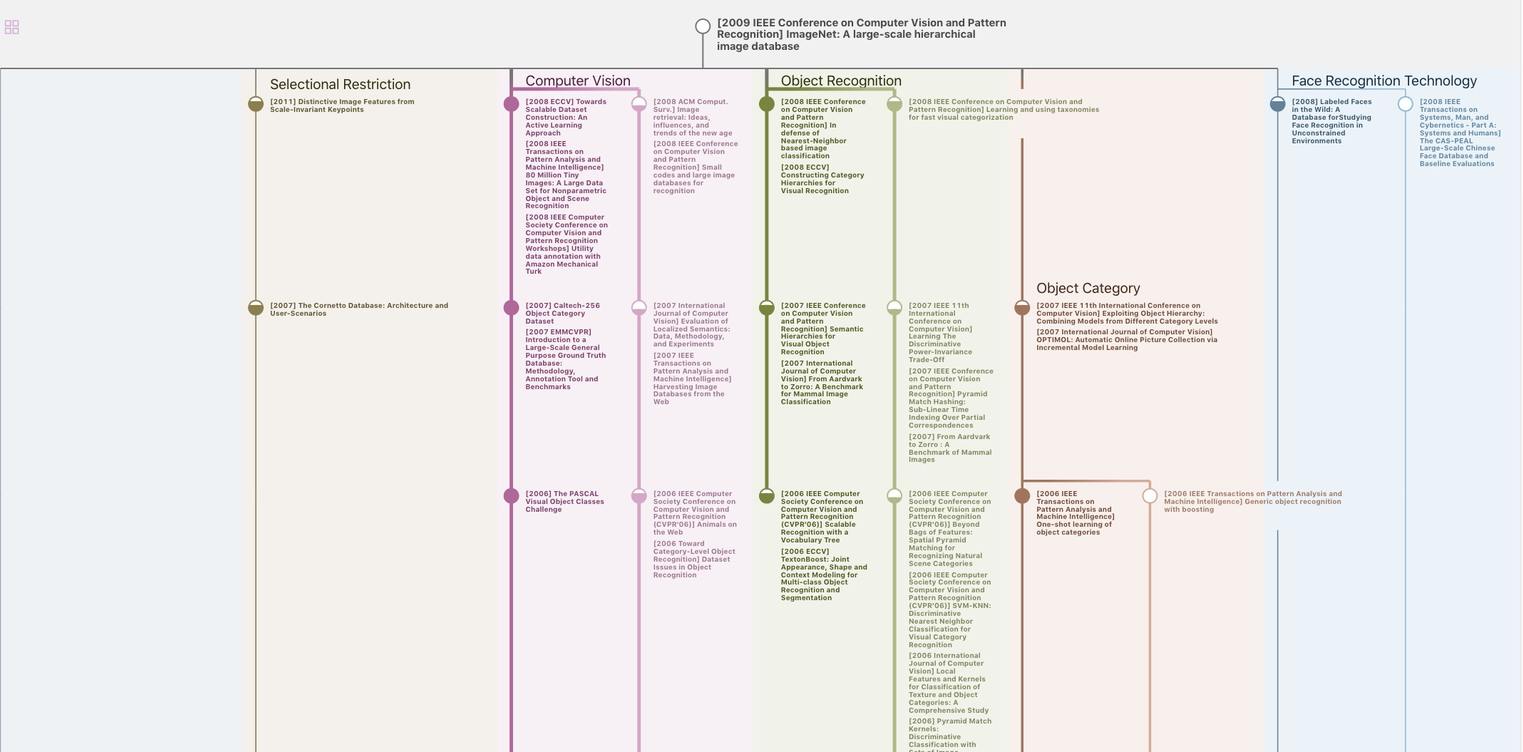
生成溯源树,研究论文发展脉络
Chat Paper
正在生成论文摘要