A New Random Forest Method for Longitudinal Data Classification Using a Lexicographic Bi-Objective Approach.
SSCI(2020)
摘要
Standard supervised machine learning methods often ignore the temporal information represented in longitudinal data, but that information can lead to more precise predictions in classification tasks. Data preprocessing techniques and classification algorithms can be adapted to cope directly with longitudinal data inputs, making use of temporal information such as the time-index of features and previous measurements of the class variable. In this article, we propose two changes to the classification task of predicting age-related diseases in a real-world dataset created from the English Longitudinal Study of Ageing. First, we explore the addition of previous measurements of the class variable, and estimating the missing data in those added features using intermediate classifiers. Second, we propose a new split-feature selection procedure for a random lorest's decision trees, which considers the candidate features' time-indexes, in addition to the information gain ratio. Our experiments compared the proposed approaches to baseline approaches, in 3 prediction scenarios, varying the "time gap" for the prediction - how many years in advance the class (occurrence of an age-related disease) is predicted. The experiments were performed on 10 datasets varying the class variable, and showed that the proposed approaches increased the random forest's predictive accuracy.
更多查看译文
关键词
split-feature selection procedure,information gain ratio,baseline approaches,class variable,random forest method,longitudinal data classification,classification task,data preprocessing techniques,classification algorithms,longitudinal data inputs,real-world dataset,lexicographic bi-objective approach,standard supervised machine learning methods,decision trees,candidate features time-indexes
AI 理解论文
溯源树
样例
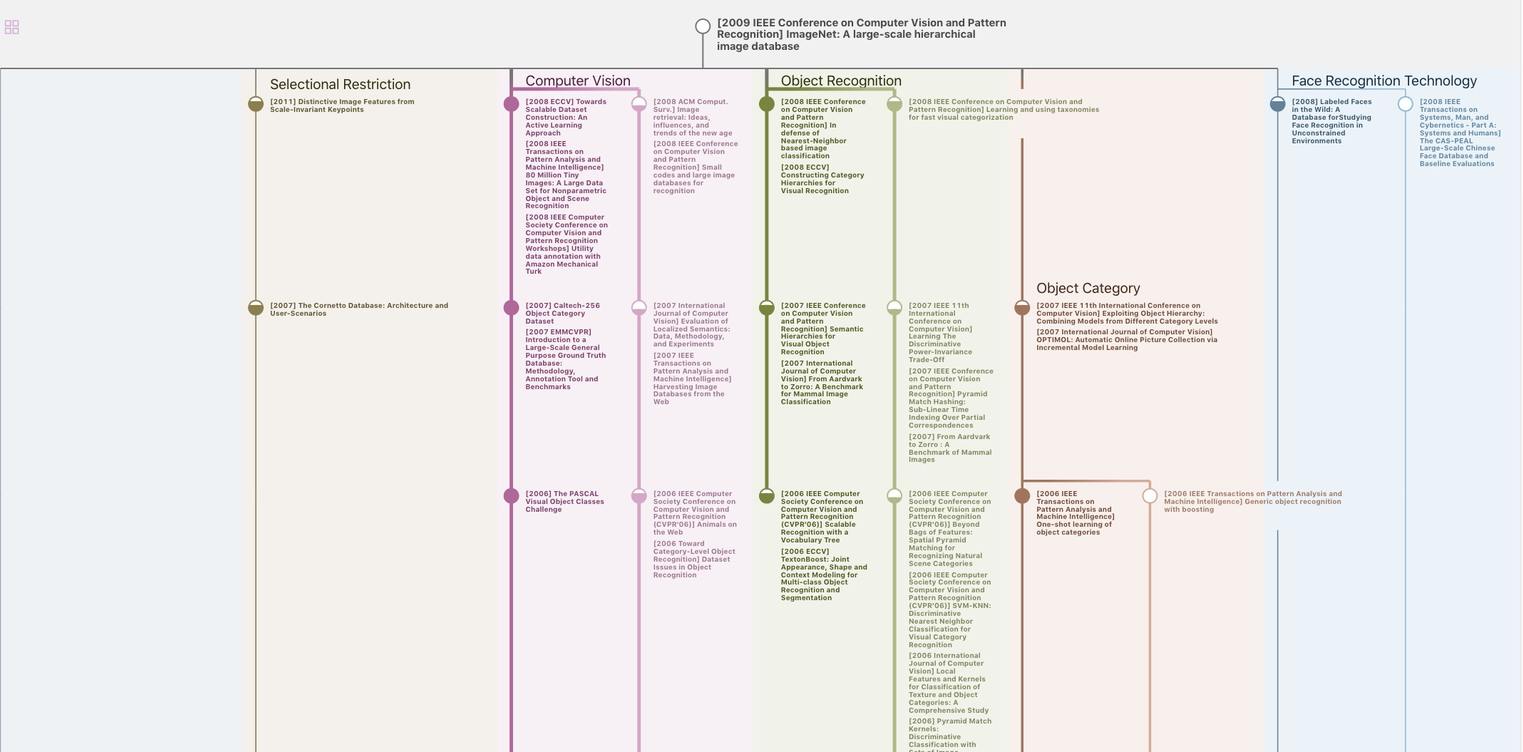
生成溯源树,研究论文发展脉络
Chat Paper
正在生成论文摘要