Chaos, Machine Learning and Deep Learning based Hybrid to forecast Consumer Price Index Inflation in India.
SSCI(2020)
摘要
In this study, we proposed a 2-stage hybrid approach for financial time series forecasting wherein chaos is modeled in stage-1 followed by forecasting is accomplished using machine learning and deep learning algorithms in stage-2. The effectiveness of the proposed hybrid is tested on forecasting Consumer Price Index Inflation of Food & Beverages, Fuel & Light, and Headline in India. This is a first-of-its-kind study where chaos is modeled and deep learning is employed in forecasting macroeconomic time series. From the results, it is inferred that Chaos + Machine learning hybrids yielded better forecasts than pure machine learning algorithms without Chaos in terms of Symmetric Mean Absolute Percentage Error (SMAPE), Theil's U statistic and Directional statistic across all the data sets. A deep learning model namely. Long Short Term Memory (LSTM) was also employed but without much success. The results of 2-stage hybrid models are compared with models without accounting for chaos. The results arc encouraging and these hybrids can be applied to predict other financial time series.
更多查看译文
关键词
Chaotic Time Series,Consumer price index inflation forecasting,GRRN,GMDH,Machine Learning,Deep Learning
AI 理解论文
溯源树
样例
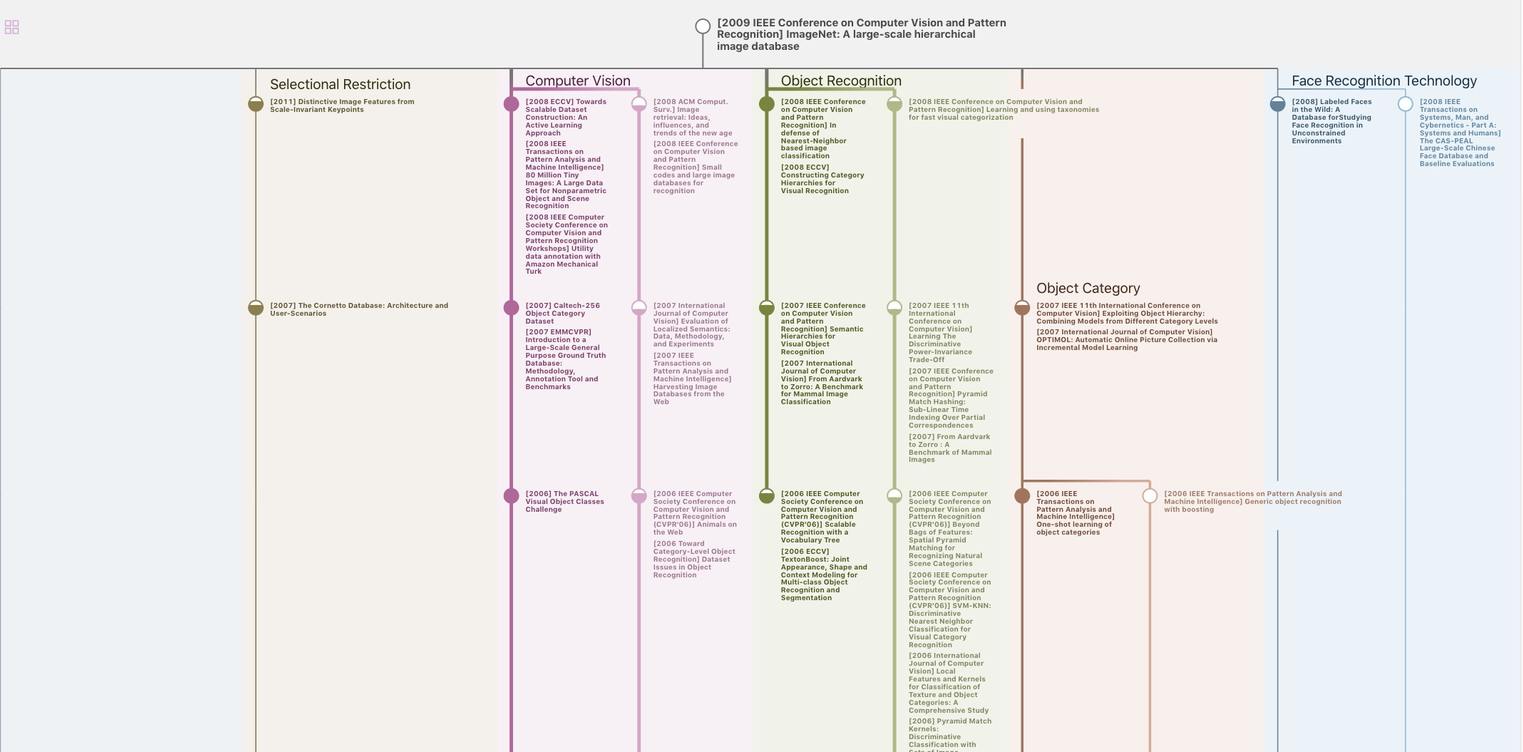
生成溯源树,研究论文发展脉络
Chat Paper
正在生成论文摘要