DeepHealth: Deep Representation Learning with Autoencoders for Healthcare Prediction
2020 IEEE Symposium Series on Computational Intelligence (SSCI)(2020)
摘要
General practitioners/doctors usually make disease diagnoses based on some predefined rules, which may lead to omitting some important hidden factors. Deep neural networks are well known for their ability to extract features from large amounts of labeled data, which can be used to learn and discover knowledge from electronic medical records (EMRs). Existing methods have mostly focused on treating deep networks as black-box feature extractors based on the information of labels. However, it is labor-intensive and expensive to manually generate ground-truth labels for EMRs. In this paper, we propose DeepHealth, a general semi-supervised learning framework for automatic diagnoses and feature interpretation based on autoencoders. Specifically, our end-to-end DeepHealth framework is constructed of two parts: a supervised classifier network for extracting high-level representation for classification; an unsupervised autoencoder network to make sure the features extracted from the input are meaningful and can be further used for reconstruction. Compared with existing methods, the experimental results show that our method, on both numerical data and medical images, can achieve the state-of-the-art results for EMRs classification. Hence, DeepHealth is a general flexible framework for high-precision diagnoses in healthcare, it can utilize both labeled and unlabeled data to address the problem of insufficient labels in EMRs, meanwhile, it can also reconstruct medical images to help interpret the features learned by a deep neural model.
更多查看译文
关键词
EMRs,DeepHealth,autoencoders,classification,reconstruction
AI 理解论文
溯源树
样例
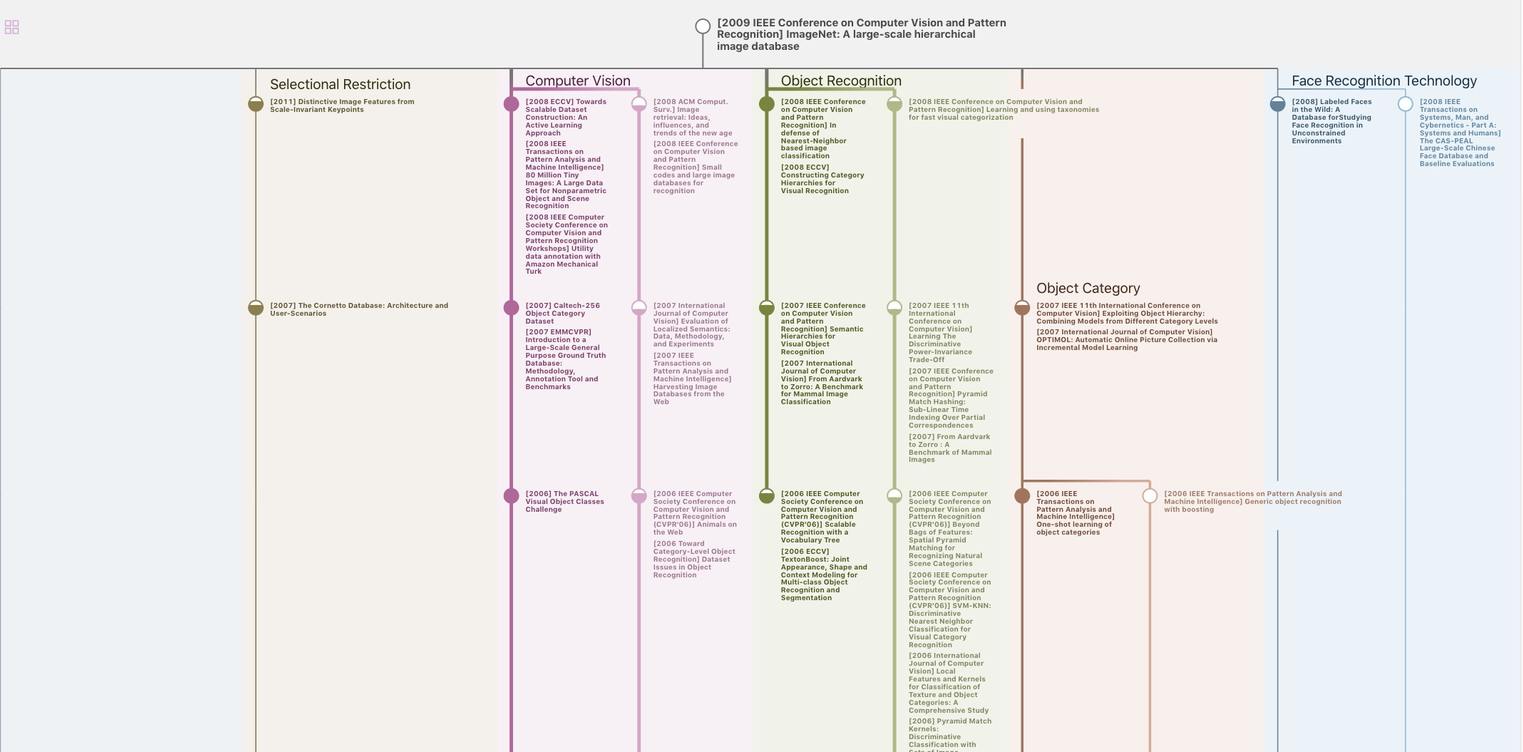
生成溯源树,研究论文发展脉络
Chat Paper
正在生成论文摘要