Multi-view learning for context-aware extractive summarization
SSCI(2020)
摘要
Natural language processing techniques have witnessed a notable success in many applications, such as dialogue generation, machine translation, and document summarization. Among them, the study of document summarization is an active research area, which aims to generate a concise version of the original document and preserve its most informative content. However, the existing work fails to consider the interaction among raw data (in terms of contextual similarity and difference), which results in inaccurate and even conflicting outcomes. In this paper, a novel context-aware extractive summarization algorithm is proposed by utilizing the concept of multi-view learning. Multi-view data are represented using latent topics, by parameterizing multiple Gaussian distributions with trainable hyper-parameters. Then those extracted latent topics are fused and integrated to produce the final summarization output. The experiments on one of the largest online-available cartoon data resources demonstrate the superior performance of the proposed algorithm, by achieving the state-of-the-art summarization results when compared with other contemporary approaches.
更多查看译文
关键词
Extractive summarization,Multi-view learning,Autoencoder,Natural language processing
AI 理解论文
溯源树
样例
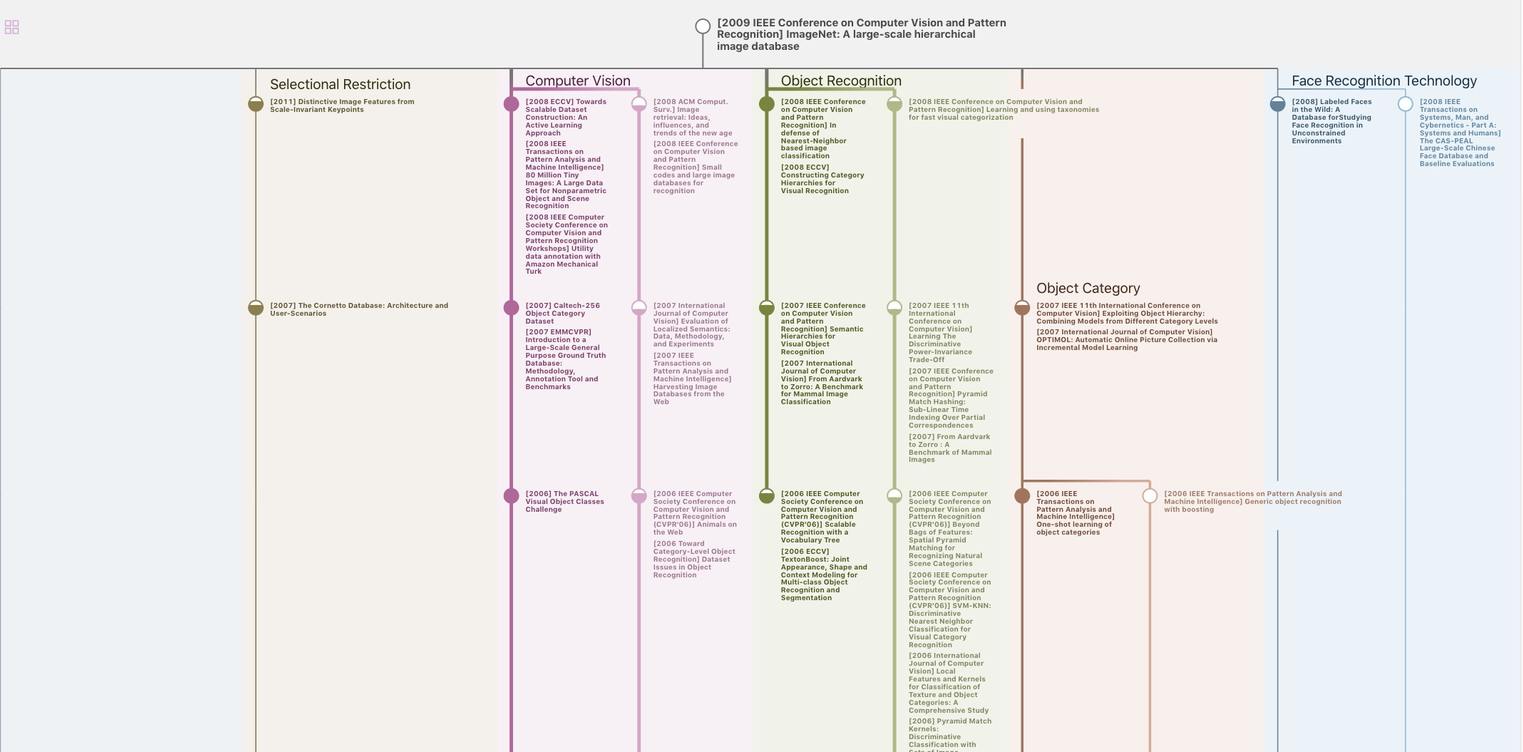
生成溯源树,研究论文发展脉络
Chat Paper
正在生成论文摘要