Small is Beautiful: Distributed Orchestration of Spatial Deep Learning Workloads
2020 IEEE/ACM 13th International Conference on Utility and Cloud Computing (UCC)(2020)
摘要
Several domains such as agriculture, urban sustainability, and meteorology entail processing satellite imagery for modeling and decision-making. In this study, we describe our novel methodology to train deep learning models over collections of satellite imagery. Deep learning models are computationally and resource expensive. As dataset sizes increase, there is a corresponding increase in the CPU, GPU, disk, and network I/O requirements to train models. Our methodology exploits spatial characteristics inherent in satellite data to partition, disperse, and orchestrate model training workloads. Rather than train a single, all-encompassing model we facilitate producing an ensemble of models - each tuned to a particular spatial extent. We support query-based retrieval of targeted portions of satellite imagery including those that satisfy properties relating to cloud occlusion, We validate the suitability of our methodology by supporting deep learning models for multiple spatial analyses. Our approach is agnostic of the underlying deep learning library. Our extensive empirical benchmark demonstrates the suitability of our methodology to not just preserve accuracy, but reduce completion times by 13.9× while reducing data movement costs by 4 orders of magnitude and ensuring frugal resource utilization.
更多查看译文
关键词
Deep Learning,Spatial Data,Workload Orchestration
AI 理解论文
溯源树
样例
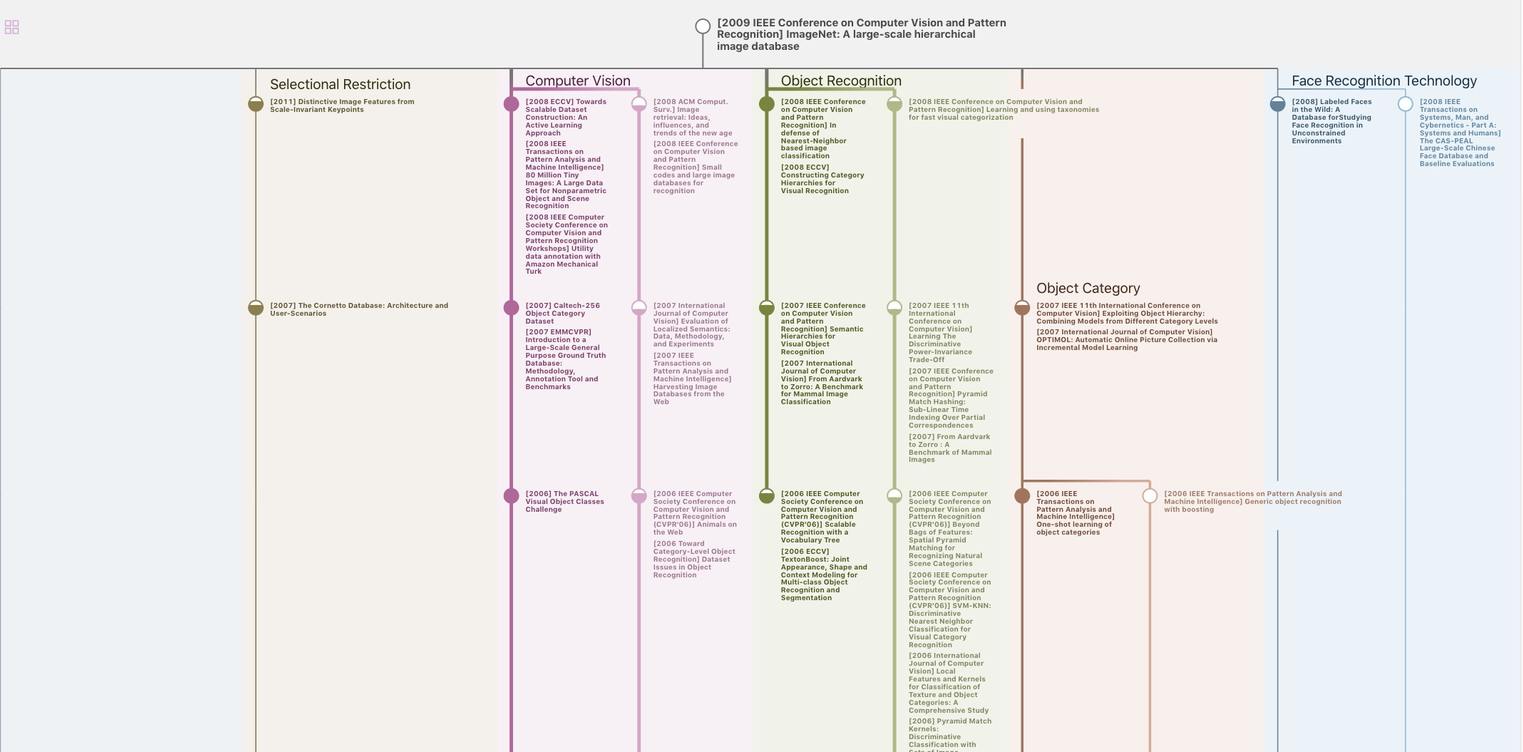
生成溯源树,研究论文发展脉络
Chat Paper
正在生成论文摘要