Deep Unsupervised Workload Sequence Anomaly Detection with Fusion of Spatial and Temporal Features in the Cloud
2020 IEEE 13th International Conference on Cloud Computing (CLOUD)(2020)
摘要
The abnormal detection of the workload sequence is designed to achieve intelligent operation and management of the cloud platform to improve operational efficiency. Due to the diversity of workload sequence variation patterns in the large-scale cloud, it is difficult for the traditional abnormal detection methods to extract features effectively, which leads to detecting anomalies inaccurately. In this paper, a deep unsupervised anomaly sequence detection model with fusion of spatial and temporal features of workload sequence (TS-DeepSVDD) is proposed. To extract the spatial and temporal features of the workload sequence, the model introduces convolutional recurrent neural network (CRNN) to improve network architecture with deep support vector data description (DeepSVDD). First, a convolutional neural network (CNN) module extracts the spatial features of the workload sequence. Second, for the acquired spatial features vectors, the bidirectional long short-term memory (BiLSTM) module extracts temporal features. Finally, the features vectors training support vector data description (SVDD) classifier fuses with the deep features of the workload sequence spatial and temporal features. TS-DeepSVDD extracts the deep features of the workload sequence from the spatial and temporal dimensions. It achieves a comprehensive description of the inherent law of the workload sequence, increasing the differentiation between normal and abnormal sequences. The simulation dataset and Google trace dataset are used respectively for verification. The results show TS-DeepSVDD can detect different abnormal sequences more accurately than the traditional unsupervised anomaly detection methods.
更多查看译文
关键词
cloud platform,workload sequence,spatial feature,temporal feature,anomaly detection
AI 理解论文
溯源树
样例
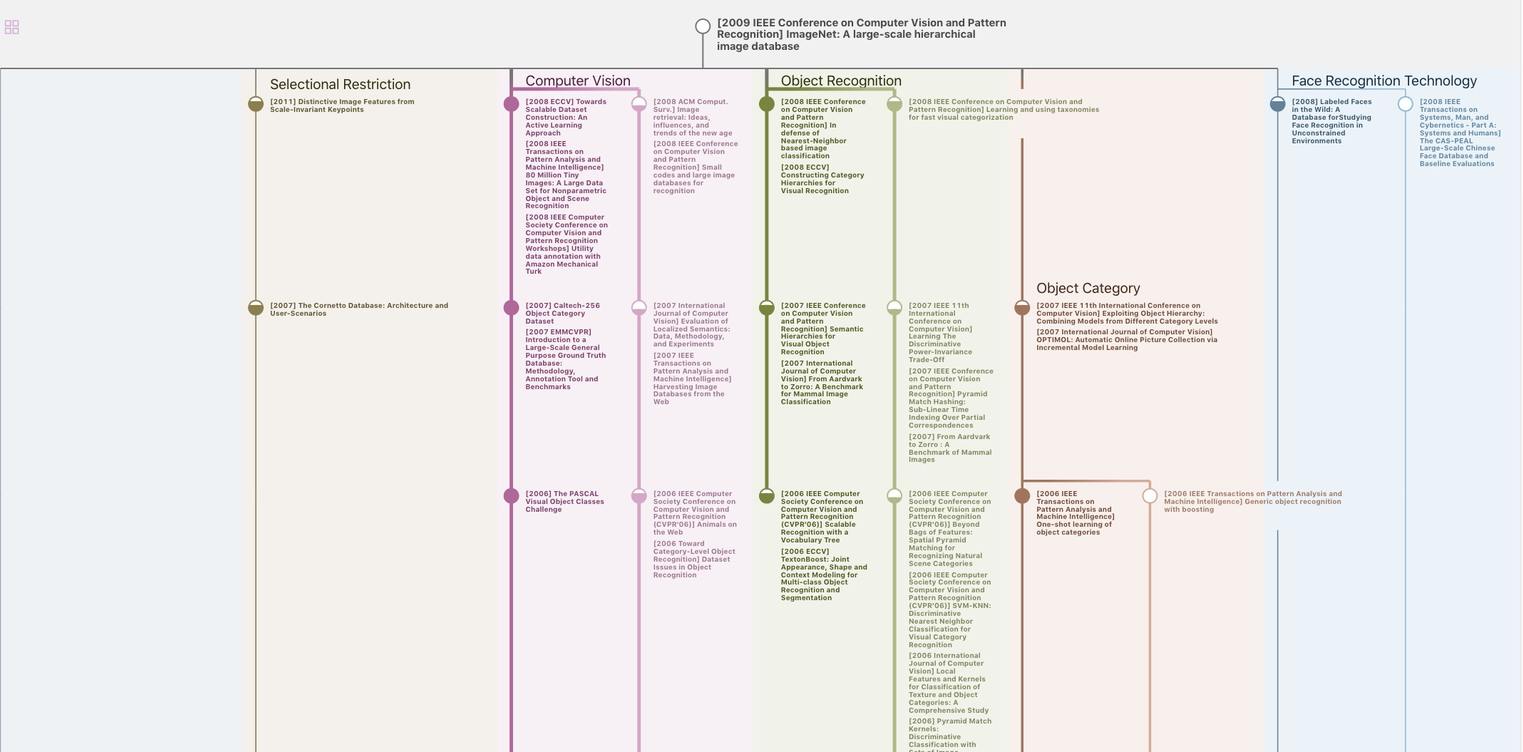
生成溯源树,研究论文发展脉络
Chat Paper
正在生成论文摘要