Learning Common And Transferable Feature Representations For Multi-Modal Data
2020 IEEE INTELLIGENT VEHICLES SYMPOSIUM (IV)(2020)
摘要
LiDAR sensors are crucial in automotive perception for accurate object detection. However, LiDAR data is hard to interpret for humans and consequently time-consuming to label. Whereas camera data is easy interpretable and thus, comparably simpler to label. Within this work we present a transductive transfer learning approach to transfer the knowledge for the object detection task from images to point cloud data. We propose a multi-modal adversarial Auto Encoder architecture which disentangles uni-modal features into two groups: common (transferable) features, and complementary (modality-specific) features. This disentanglement is based on the hypothesis that a set of common features exist. An important point of our framework is that the disentanglement is learned in an unsupervised manner. Furthermore, the results show that only a small amount of multi-modal data is needed to learn the disentanglement, and thus to transfer the knowledge between modalities. As a result we our experiments show that training with 75% less data of the KITTI objects, the classification accuracy achieved is of 71.75%, only 3.12% less than when using the full data set. The implications of these findings can have great impact in perception pipelines based on LIDAR data.
更多查看译文
关键词
transferable feature representations,multimodal data,LiDAR sensors,automotive perception,accurate object detection,LiDAR data,camera data,transductive transfer learning approach,object detection task,point cloud data,common features,modality-specific features,disentanglement,KITTI objects,unimodal features,multimodal adversarial autoencoder architecture,unsupervised learning,classification accuracy
AI 理解论文
溯源树
样例
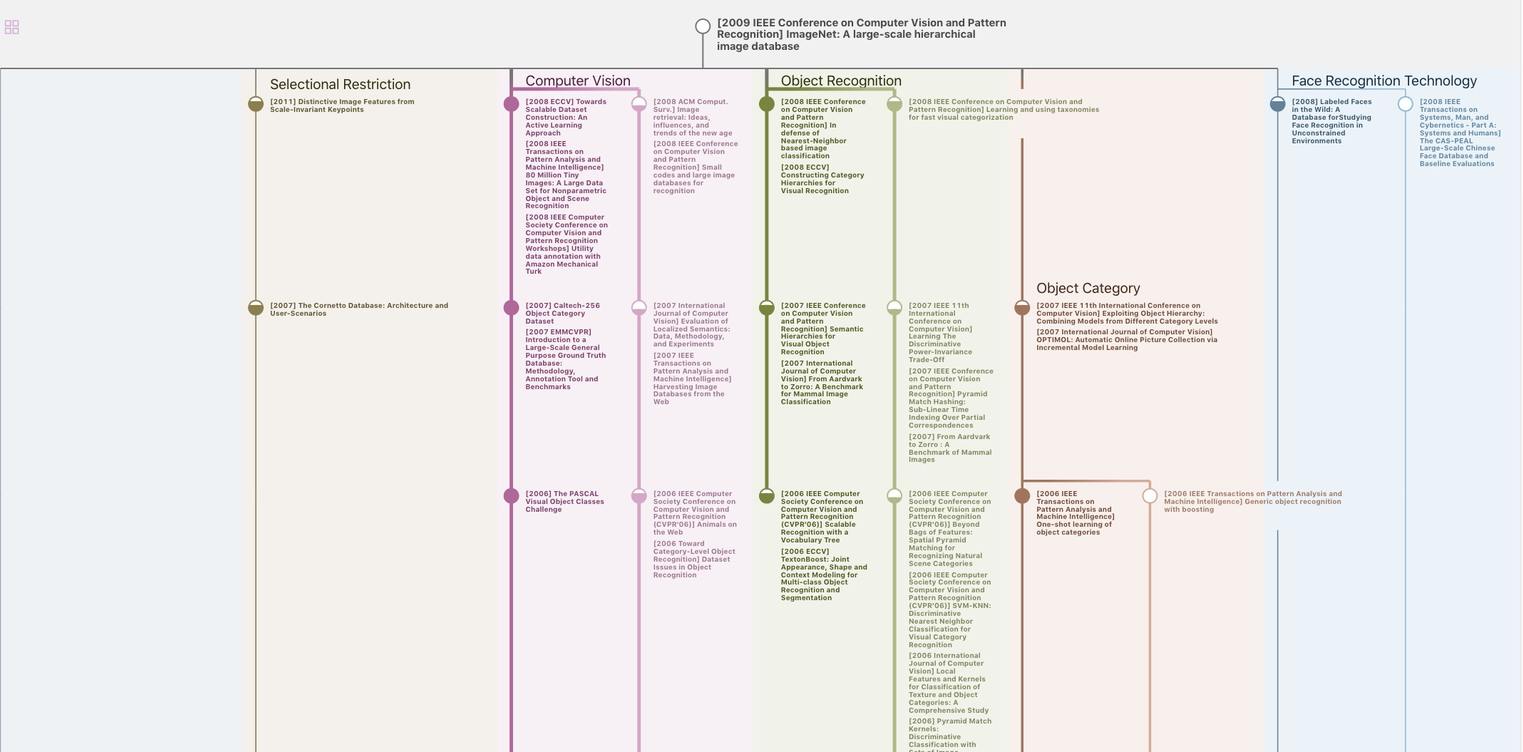
生成溯源树,研究论文发展脉络
Chat Paper
正在生成论文摘要