Unadjusted Langevin Algorithm for Non-convex Weakly Smooth Potentials
Communications in Mathematics and Statistics(2023)
摘要
Discretization of continuous-time diffusion processes is a widely recognized method for sampling. However, the canonical Euler Maruyama discretization of the Langevin diffusion process, referred as unadjusted Langevin algorithm (ULA), studied mostly in the context of smooth (gradient Lipschitz) and strongly log-concave densities, is a considerable hindrance for its deployment in many sciences, including statistics and machine learning. In this paper, we establish several theoretical contributions to the literature on such sampling methods for non-convex distributions. Particularly, we introduce a new mixture weakly smooth condition, under which we prove that ULA will converge with additional log-Sobolev inequality. We also show that ULA for smoothing potential will converge in L_2 -Wasserstein distance. Moreover, using convexification of nonconvex domain (Ma et al. in Proc Natl Acad Sci 116(42):20881–20885, 2019) in combination with regularization, we establish the convergence in Kullback–Leibler divergence with the number of iterations to reach ϵ -neighborhood of a target distribution in only polynomial dependence on the dimension. We relax the conditions of Vempala and Wibisono (Advances in Neural Information Processing Systems, 2019) and prove convergence guarantees under isoperimetry, and non-strongly convex at infinity.
更多查看译文
关键词
Langevin Monte Carlo,Kullback-Leibler divergence,Log-Sobolev inequality,Convexification,Mixture weakly smooth
AI 理解论文
溯源树
样例
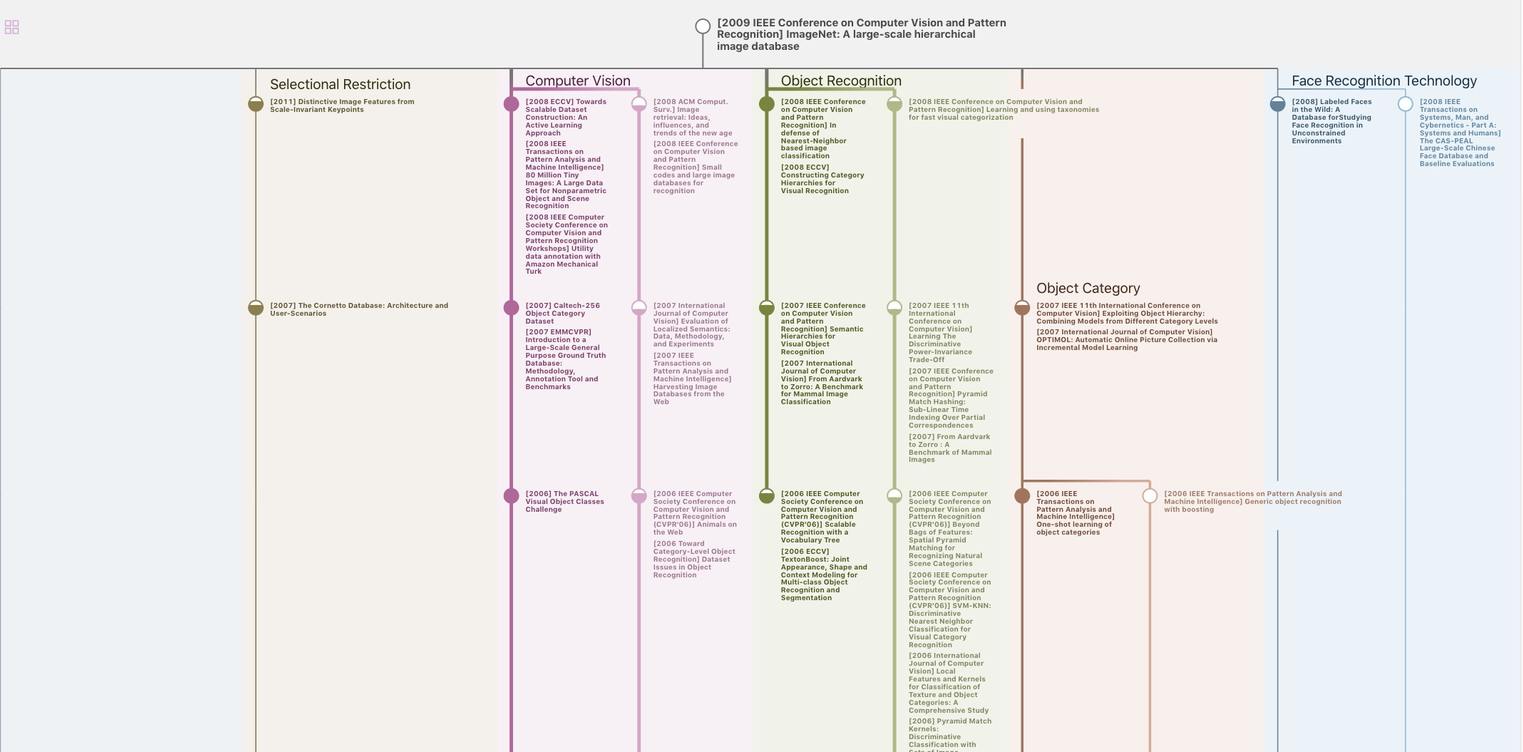
生成溯源树,研究论文发展脉络
Chat Paper
正在生成论文摘要