Privacy-Preserving Learning of Human Activity Predictors in Smart Environments
IEEE CONFERENCE ON COMPUTER COMMUNICATIONS (IEEE INFOCOM 2021)(2021)
摘要
The daily activities performed by a disabled or elderly person can be monitored by a smart environment, and the acquired data can be used to learn a predictive model of user behavior. To speed up the learning, several researchers designed collaborative learning systems that use data from multiple users. However, disclosing the daily activities of an elderly or disabled user raises privacy concerns. In this paper, we use state-of-the-art deep neural network-based techniques to learn predictive human activity models in the local, centralized, and federated learning settings. A novel aspect of our work is that we carefully track the temporal evolution of the data available to the learner and the data shared by the user. In contrast to previous work where users shared all their data with the centralized learner, we consider users that aim to preserve their privacy. Thus, they choose between approaches in order to achieve their goals of predictive accuracy while minimizing the shared data. To help users make decisions before disclosing any data, we use machine learning to predict the degree to which a user would benefit from collaborative learning. We validate our approaches on real-world data.
更多查看译文
关键词
privacy-preserving learning,human activity predictors,smart environment,daily activities,disabled person,elderly person,user behavior,privacy concerns,deep neural network-based techniques,predictive human activity models,centralized learner,predictive accuracy,machine learning,real-world data,collaborative learning system design,local learning settings,centralized learning settings,federated learning settings,data temporal evolution,shared data minimization,decision making
AI 理解论文
溯源树
样例
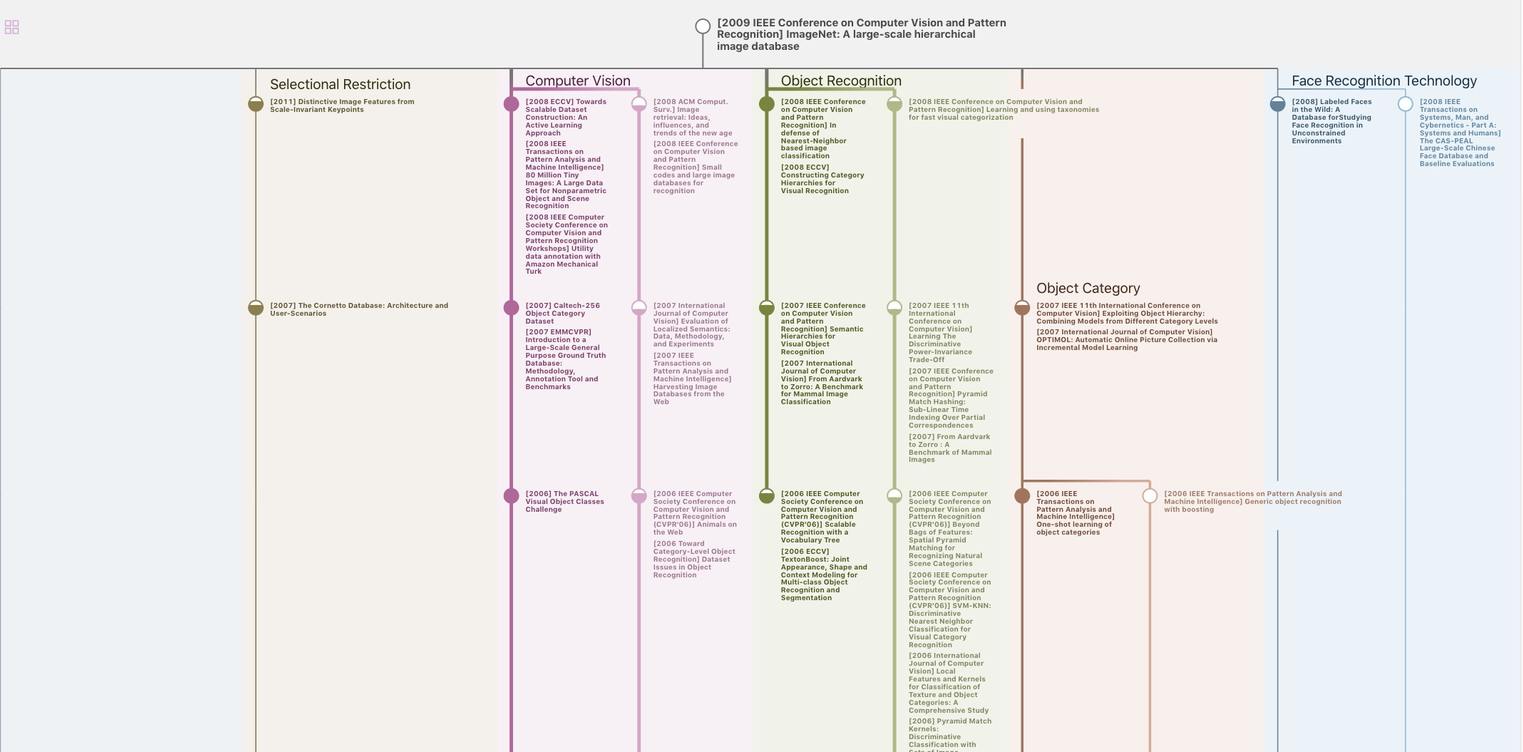
生成溯源树,研究论文发展脉络
Chat Paper
正在生成论文摘要